Regret Bounds for Risk-Sensitive Reinforcement Learning
NeurIPS 2022(2022)
摘要
In safety-critical applications of reinforcement learning such as healthcare and robotics, it is often desirable to optimize risk-sensitive objectives that account for tail outcomes rather than expected reward. We prove the first regret bounds for reinforcement learning under a general class of risk-sensitive objectives including the popular CVaR objective. Our theory is based on a novel characterization of the CVaR objective as well as a novel optimistic MDP construction.
更多查看译文
关键词
Risk-sensitive reinforcement learning,CVaR objective
AI 理解论文
溯源树
样例
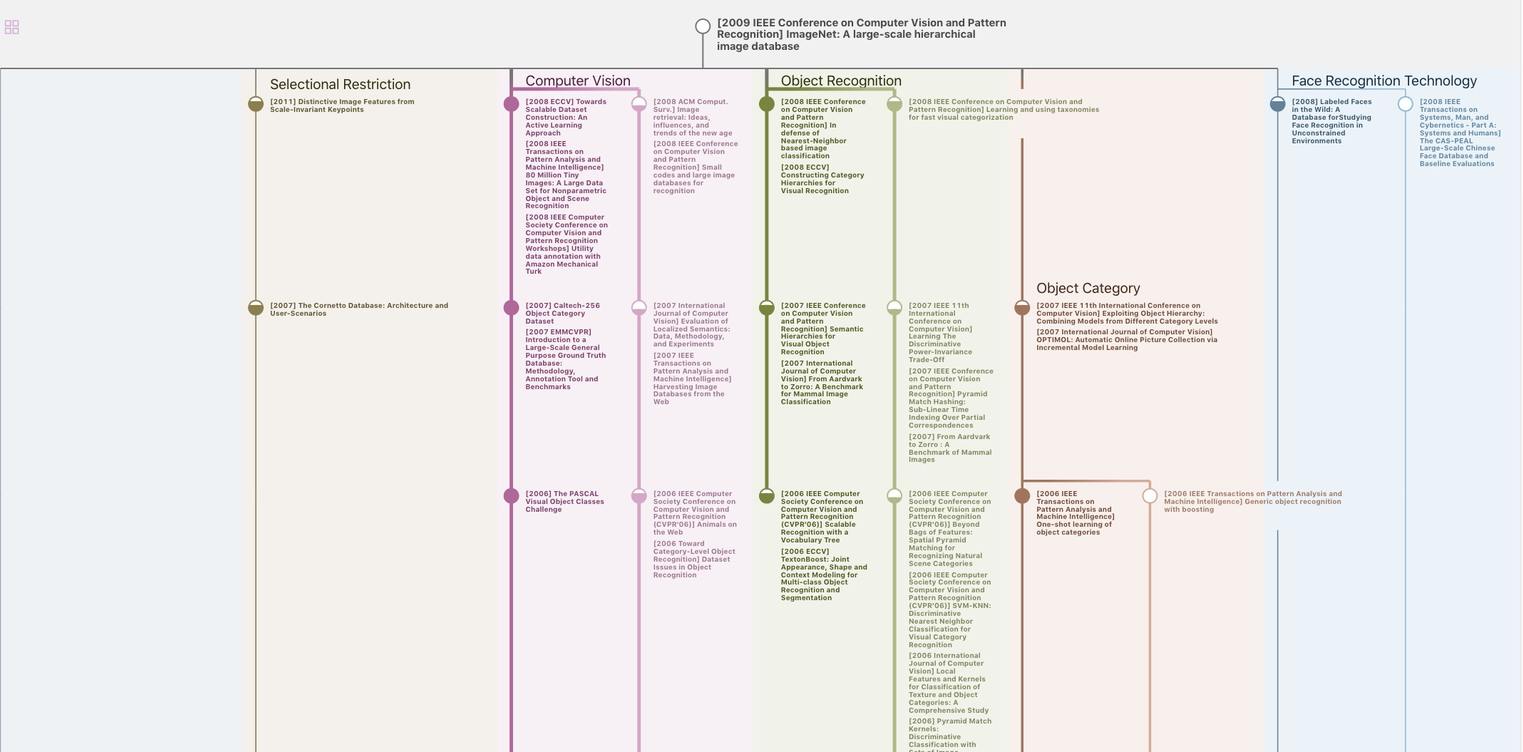
生成溯源树,研究论文发展脉络
Chat Paper
正在生成论文摘要