Masked Autoencoders for Low dose CT denoising
arxiv(2022)
摘要
Low-dose computed tomography (LDCT) reduces the X-ray radiation but compromises image quality with more noises and artifacts. A plethora of transformer models have been developed recently to improve LDCT image quality. However, the success of a transformer model relies on a large amount of paired noisy and clean data, which is often unavailable in clinical applications. In computer vision and natural language processing fields, masked autoencoders (MAE) have been proposed as an effective label-free self-pretraining method for transformers, due to its excellent feature representation ability. Here, we redesign the classical encoder-decoder learning model to match the denoising task and apply it to LDCT denoising problem. The MAE can leverage the unlabeled data and facilitate structural preservation for the LDCT denoising model when ground truth data are missing. Experiments on the Mayo dataset validate that the MAE can boost the transformer's denoising performance and relieve the dependence on the ground truth data.
更多查看译文
关键词
low dose ct denoising
AI 理解论文
溯源树
样例
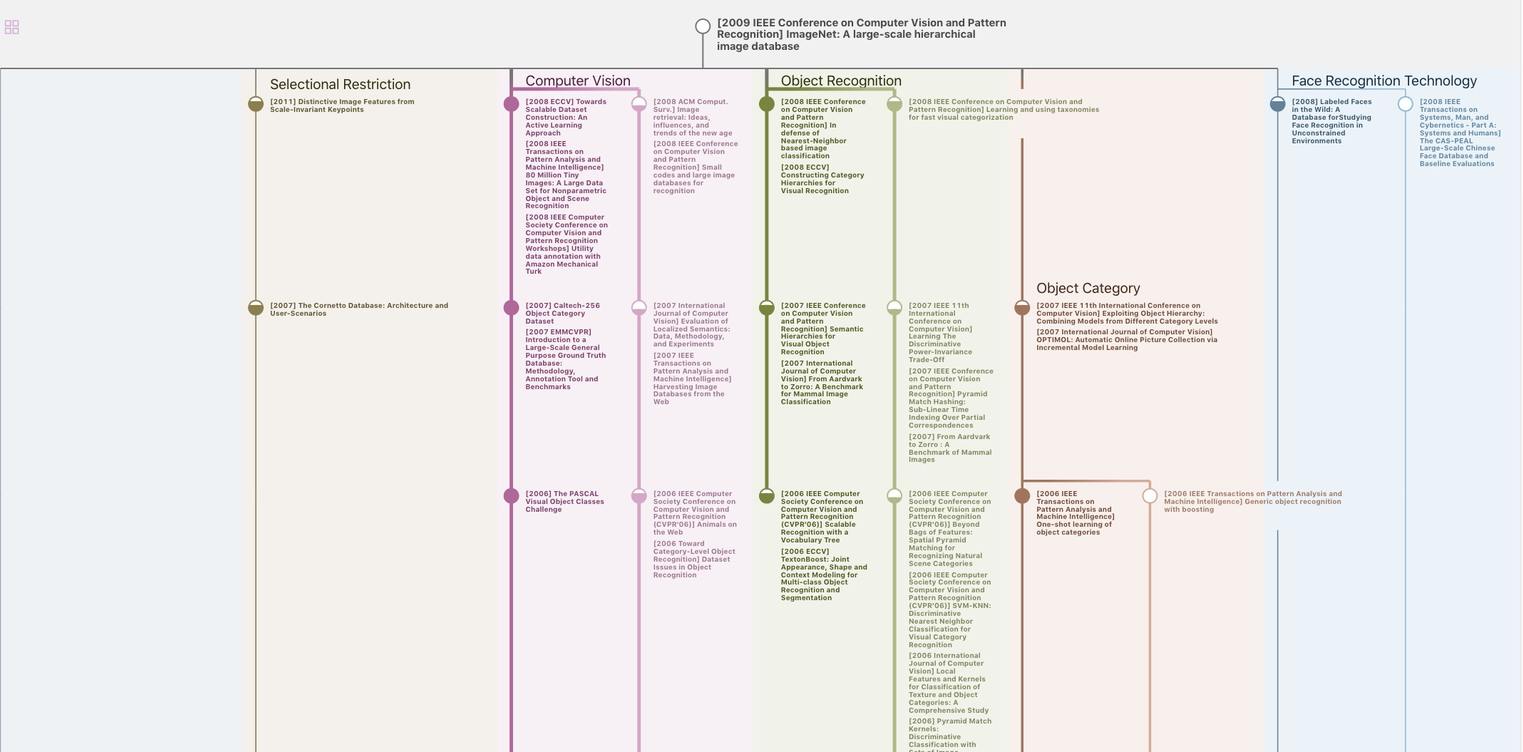
生成溯源树,研究论文发展脉络
Chat Paper
正在生成论文摘要