Detecting chaos in adversarial examples
Chaos, Solitons & Fractals(2022)
摘要
The puzzling phenomenon of adversarial examples continues to attract significant research within the machine learning community. The confirmation that adversarial examples can arise in natural real-life circumstances has but increased the interest. While several methods have been proposed for both generating adversarial examples and defending against them, in this work we focus on a previous serendipitous discovery indicating that they can be considered as chaotic signals. More specifically, it has been recently shown that measures of chaoticity in the input signal can be used to detect adversarial examples efficiently. In this work, we extend that approach in two aspects, leading to significant improvements in detection accuracy as demonstrated by results obtained in experiments with four datasets and using seven different attack methods.
更多查看译文
关键词
Adversarial examples,Lyapunov stability,Chaos theory,Trustworthy machine learning,Neural networks,Deep learning
AI 理解论文
溯源树
样例
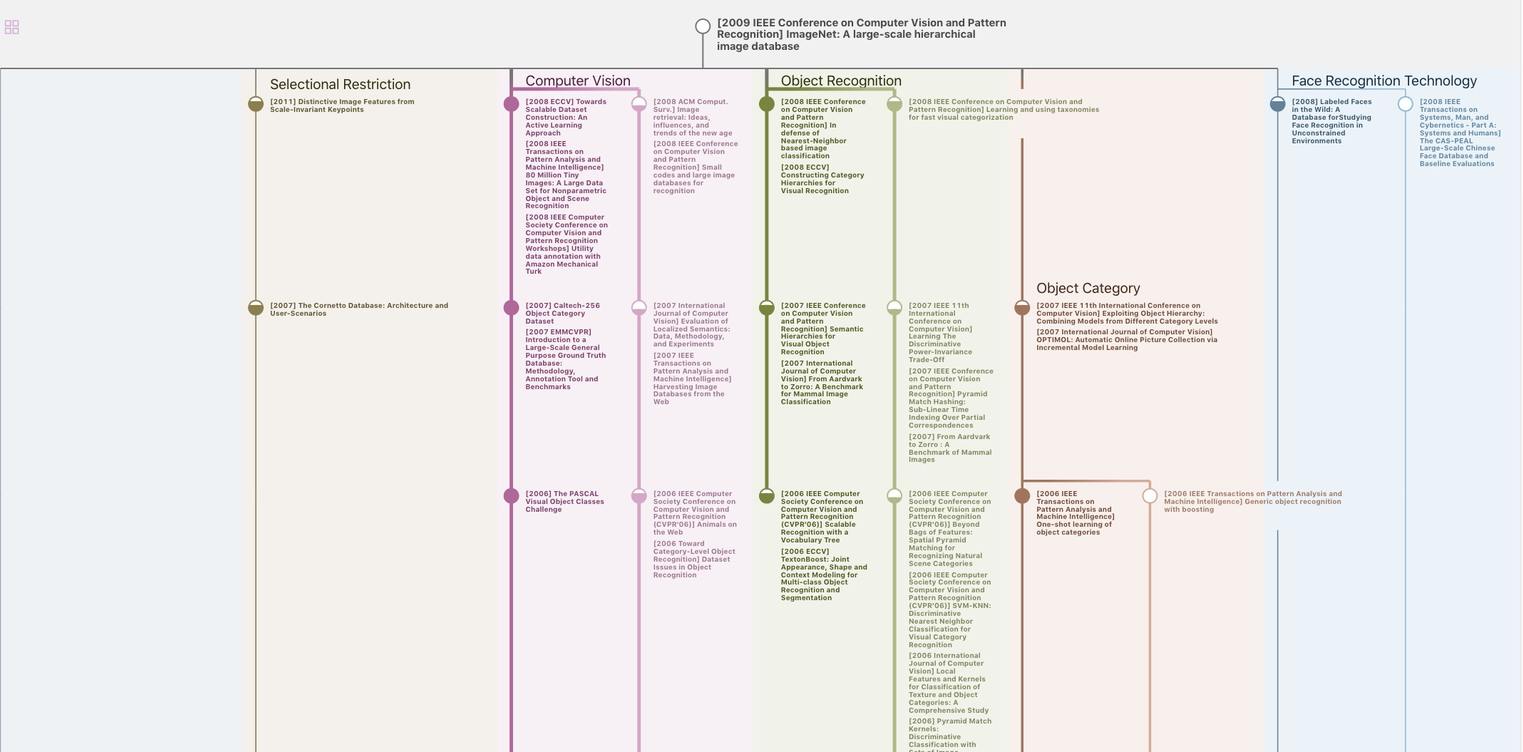
生成溯源树,研究论文发展脉络
Chat Paper
正在生成论文摘要