Deep sparse representation via deep dictionary learning for reinforcement learning
2022 41st Chinese Control Conference (CCC)(2022)
摘要
Reinforcement Learning (RL) currently has been widely applied for various fields of decision-making, which relies on the function approximation for tackling complicated tasks. However, most RL models including Deep Reinforcement Learning (DRL) suffer from the catastrophic interference, resulting in poor control performance. Recently, the sparse representation has been proven effective for avoiding the interference and is easy to train, but shallow sparse representations have limited representation capability. In this paper, we proposed to employ the deep dictionary learning (DDL) model for reinforcement learning as a joint optimization problem over the deep sparse representation and the function approximation, which considers a multi-layer dictionary for obtaining a deeper latent sparse representation with better data representation capability. Firstly, to address the difficulties in optimization of DDL, we employ the decomposition method that we can decompose the multilayer problem into a set of single-layer subproblems. Secondly, to solve the joint optimization problem efficiently, we apply the alternating update scheme for updating the sparse representation, multi-layer dictionary, and weight for value prediction alternately. Thirdly, we can directly obtain the closed-form solution of the sparse representation by the Proximal Operator (PO), multi-layer dictionary and weight are trained by the gradient descent method. Finally, the agent utilizes the pre-trained multilayer dictionary and weight for RL control, and the weight is updated dynamically at each step. The experimental results on two benchmark environments demonstrate that the performance of the RL control training based on the DDL model can be significantly improved.
更多查看译文
关键词
Reinforcement Learning, Deep sparse representation, Deep dictionary learning, Proximal operator
AI 理解论文
溯源树
样例
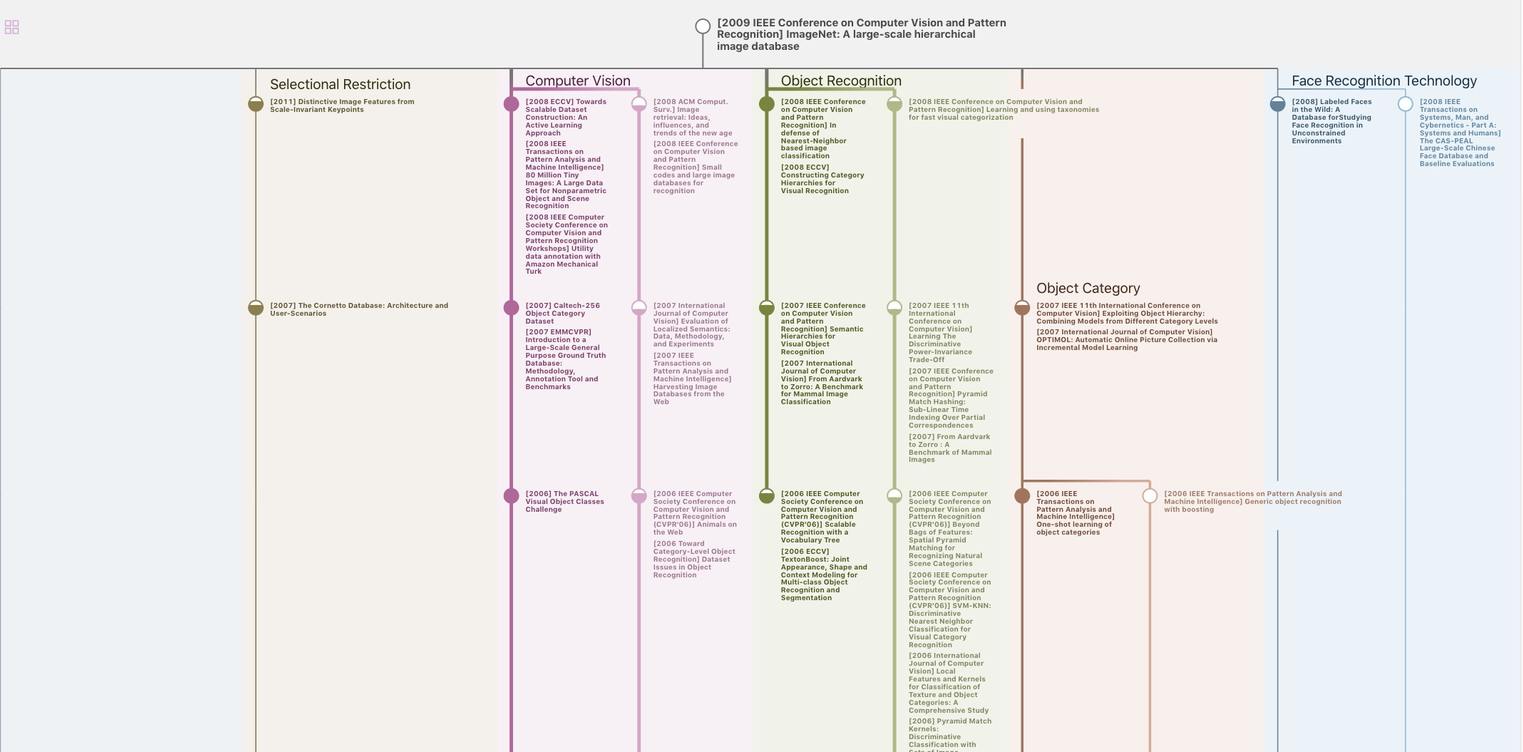
生成溯源树,研究论文发展脉络
Chat Paper
正在生成论文摘要