Rail Fatigue Crack Classification by WSCNN-GRU using Acoustic Emission
2022 41st Chinese Control Conference (CCC)(2022)
摘要
Aiming at achieving the classification of rail fatigue crack signals in high-speed railway using Acoustic Emission (AE) technology, a novel method based on the deep learning theory is proposed in this paper. The proposed method combines self-normalizing convolutional neural networks with wide first-layer kernels (WSCNN) and gated recurrent unit (GRU) to construct an effective model named WSCNN-GRU. By taking advantage of CNN and GRU, the local important information features in signals space and the temporal relevance are extracted from the AE signals. What's more, the self-normalizing properties of the activation function SeLU is selected to maintain the stability and convergence of the fatigue crack classification WSCNN-GRU model. Adaptive batch normalization (AdaBN) is utilized to improve the domain adaptation ability of the model. The method is fully verified in rail fatigue experiments, and the results show that the proposed WSCNN-GRU method achieve outstanding classification accuracy. Meanwhile, it provides an excellent way for rail fatigue crack to guarantee the high-speed railway safety.
更多查看译文
关键词
Rail Fatigue, Crack Classification, Acoustic Emission, WSCNN-GRU
AI 理解论文
溯源树
样例
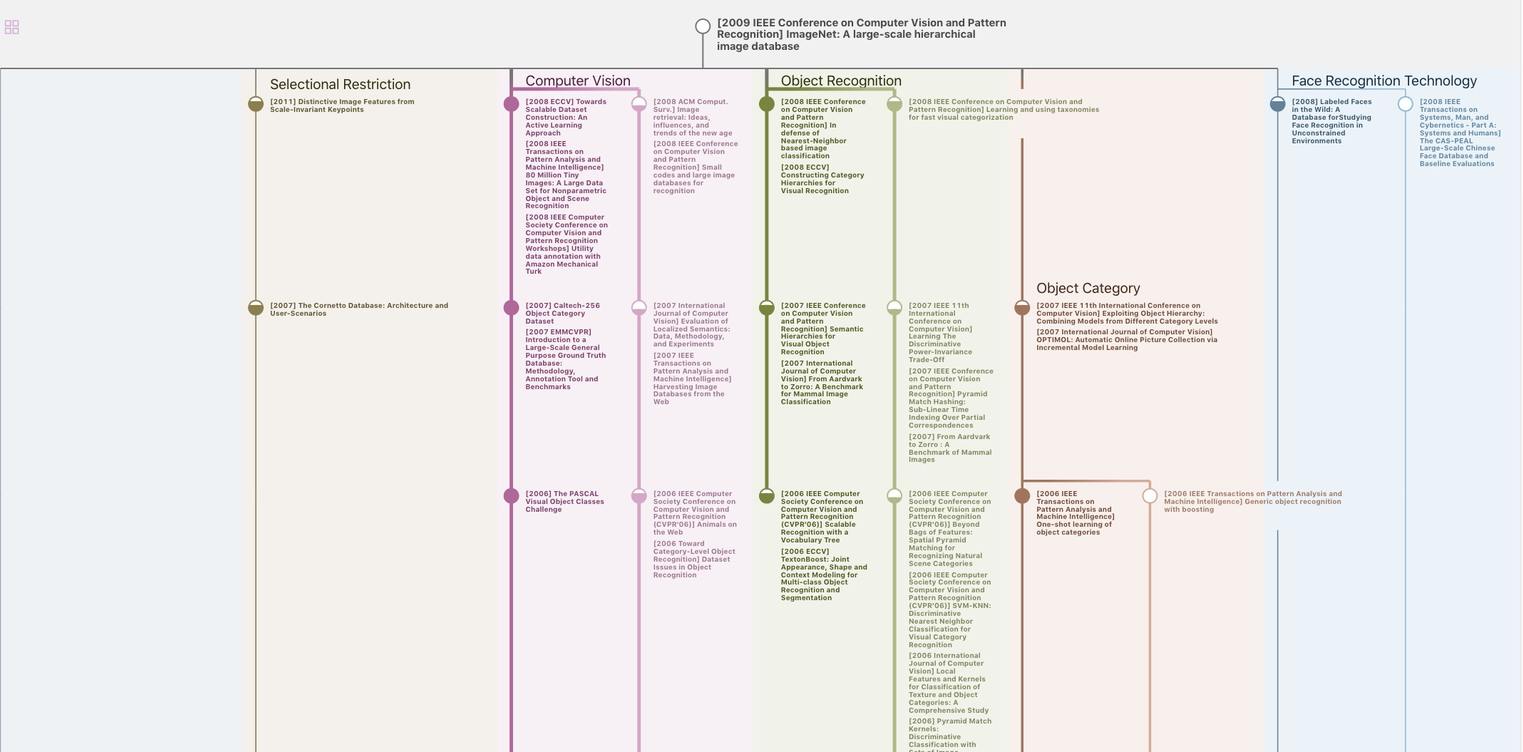
生成溯源树,研究论文发展脉络
Chat Paper
正在生成论文摘要