Joint sparse model with coupled dictionary for medical image fusion
Biomedical Signal Processing and Control(2023)
摘要
Medical image fusion has made great progress benefit from strong feature association ability of joint sparse model. Since medical images are obtained by different sensors with different imaging mechanisms, single dictionary cannot effectively characterize innovation and common information, which becomes stumbling block for effective fusion. In this paper, we propose novel joint sparse model with coupled dictionary learning for medical image fusion. Our framework design new fusion rule to enhance ability of multi-source signal preservation and retain edge/texture information. Firstly, source medical images was represented as a common sparse component and innovation sparse components with over-complete coupled dictionaries. Secondly, fused sparse coefficient is obtained by using the designed novel rule. Finally, fused results are reconstructed using fused coefficients and coupled dictionaries. Our proposed method is evaluated and compared with 9 state-of-the-art fusion methods on public Harvard dataset. Qualitative and quantitative results show that our method is generally better than the compared methods in terms of retaining source image structural and functional information.
更多查看译文
关键词
Medical image fusion,Coupled dictionary,Joint sparse model,Structural information,Functional information
AI 理解论文
溯源树
样例
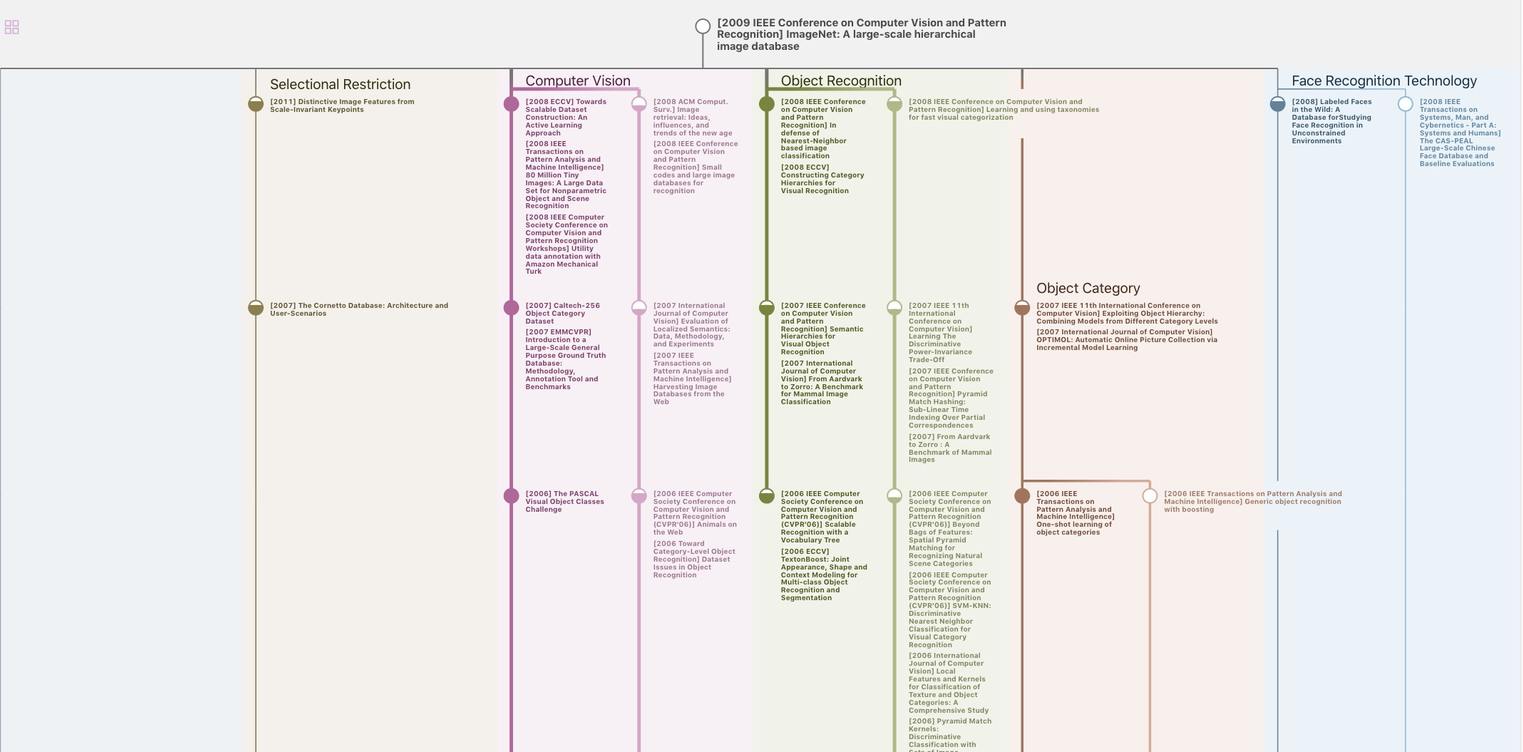
生成溯源树,研究论文发展脉络
Chat Paper
正在生成论文摘要