MFNet: The Spatio-Temporal Network for Meteorological Forecasting With Architecture Search
IEEE Geoscience and Remote Sensing Letters(2022)
摘要
Exploiting deep learning for the meteorological forecasting (MF) task is challenging due to the complex spatio-temporal correlation, non-stationarity, and imbalanced data distribution. Though with elaborate design, handcraft hierarchical architectures adopted by current methods could be far from optimal in sufficiently modeling the dynamics of meteorological data. For the MF task, this letter presents the MFNet, which is a spatio-temporal network with the Neural Architecture Search (NAS) technique. Working in the data-driven paradigm, our method is capable of automatically generating suitable architecture to model the spatio-temporal correlation. Moreover, the nonstationarity of meteorological data is explicitly modeled through simulating spatio-temporal variations in response to the intrinsic driven force of the meteorological state, and the Error Sensitive Regression (ESR) loss is introduced accounting for the imbalanced data distribution. Extensive experiments exhibit the capability of our method and demonstrate that deep learning is potential for serving as an operational technique for global MF.
更多查看译文
关键词
Deep learning,meteorological forecasting (MF),neural architecture search (NAS)
AI 理解论文
溯源树
样例
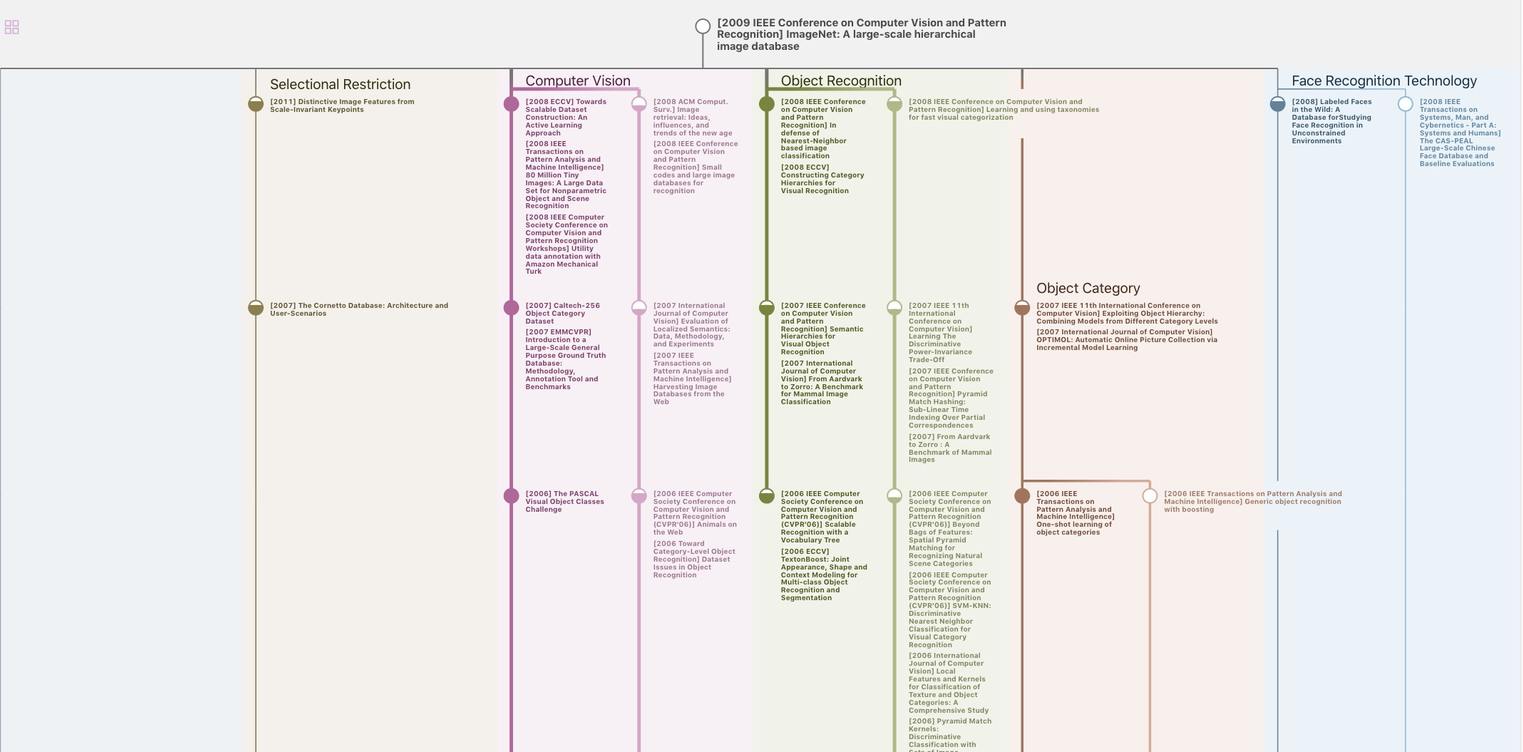
生成溯源树,研究论文发展脉络
Chat Paper
正在生成论文摘要