Learning to automatically spectate games for Esports using object detection mechanism
Expert Systems with Applications(2023)
摘要
Human game observers, who control in-game cameras and provide viewers with an engaging experience, are a vital part of electronic sports (Esports) which has emerged as a rapidly growing industry in recent years. However, such a professional human observer poses several problems. For example, they are prone to missing events occurring concurrently across the map. Further, human game observers are difficult to afford when only a small number of spectators are spectating the game. Consequently, various methods to create automatic observers have been explored, and these methods are based on defining in-game events and focus on defined events. However, these event-based methods necessitate detailed predefined events, demanding high domain knowledge when developing. Additionally, these methods cannot show scenes that contain undefined events. In this paper, we propose a method to overcome these problems by utilizing multiple human observational data and an object detection method, Mask R-CNN, in a real-time strategy game (e.g., StarCraft). By learning from human observational data, our method can observe scenes that are not defined as events in advance. The proposed model utilizes an object detection mechanism to find the area where the human spectator is interested. We consider the pattern of the two-dimensional spatial area that the spectator is looking at as the object to find, rather than treating a single unit or building as an object which is conventionally done in object detection. Consequently, we show that our automatic observer outperforms both current rule-based methods and human observers. The game observation video that compares our method and the rule-based method is available at https://www.youtube.com/watch?v=61JIfSrLHVk.
更多查看译文
关键词
Esports,Spectators,Automatic observer,Mask R-CNN,StarCraft
AI 理解论文
溯源树
样例
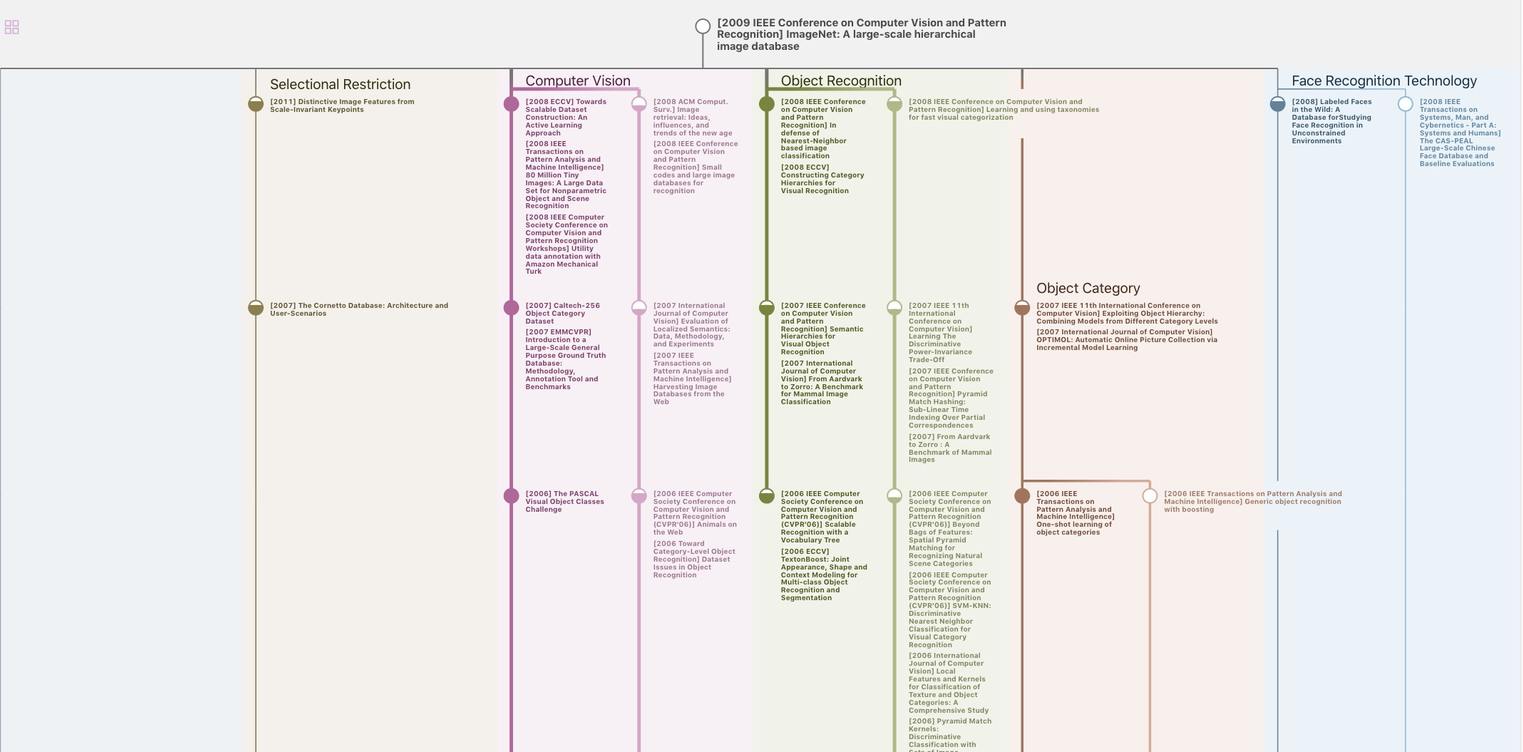
生成溯源树,研究论文发展脉络
Chat Paper
正在生成论文摘要