Experiential Explanations for Reinforcement Learning
arxiv(2023)
摘要
Reinforcement Learning approaches are becoming increasingly popular in various key disciplines, including robotics and healthcare. However, many of these systems are complex and non-interpretable, making it challenging for non-AI experts to understand or intervene in their decisions. One of the challenges of explaining RL agent behavior is that, when learning to predict future expected rewards, agents discard contextual information about their experiences when training in an environment and rely solely on expected utility. We propose a technique, Experiential Explanations, for generating local counterfactual explanations that can answer users' why-not questions by explaining the qualitative effects of the various environmental rewards on the agent's behavior. We achieve this by training additional models alongside the policy model. These models, called influence predictors, capture how different reward sources influence the agent's policy, thus restoring lost contextual information about how the policy reflects the environment. To generate explanations, we use these influence predictors in addition to the policy model to contrast between the agent's intended behavior trajectory and a counterfactual trajectory suggested by the user. A human evaluation study revealed that participants had a higher probability of correctly predicting the agent's subsequent action when presented with Experiential Explanations than other explanation types. Moreover, compared to other baseline types, participants found Experiential Explanations more useful and more often utilized the kinds of information presented in them when reasoning about the agent's actions. Experiential Explanations also outperformed other explanations in understandability, satisfaction, amount of details, completeness, usefulness, and accuracy.
更多查看译文
关键词
learning
AI 理解论文
溯源树
样例
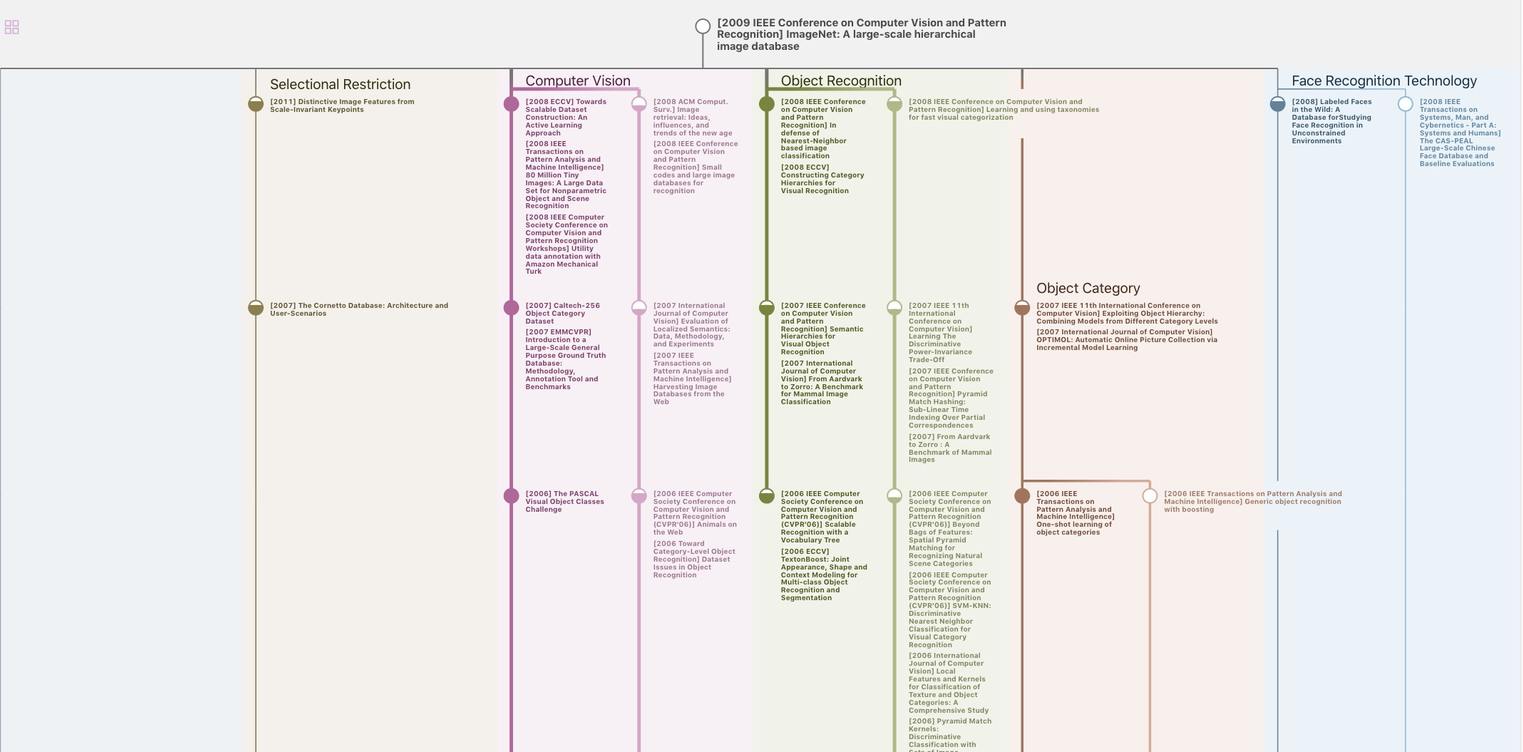
生成溯源树,研究论文发展脉络
Chat Paper
正在生成论文摘要