Neural networks for first order HJB equations and application to front propagation with obstacle terms
arxiv(2023)
摘要
We consider a deterministic optimal control problem, focusing on a finite horizon scenario. Our proposal involves employing deep neural network approximations to capture Bellman’s dynamic programming principle. This also corresponds to solving first-order Hamilton–Jacobi–Bellman (HJB) equations. Our work builds upon the research conducted by Huré et al. (SIAM J Numer Anal 59(1):525–557, 2021), which primarily focused on stochastic contexts. However, our objective is to develop a completely novel approach specifically designed to address error propagation in the absence of diffusion in the dynamics of the system. Our analysis provides precise error estimates in terms of an average norm. Furthermore, we provide several academic numerical examples that pertain to front propagation models incorporating obstacle constraints, demonstrating the effectiveness of our approach for systems with moderate dimensions (e.g., ranging from 2 to 8) and for nonsmooth value functions.
更多查看译文
关键词
first order hjb equations,front propagation,neural networks
AI 理解论文
溯源树
样例
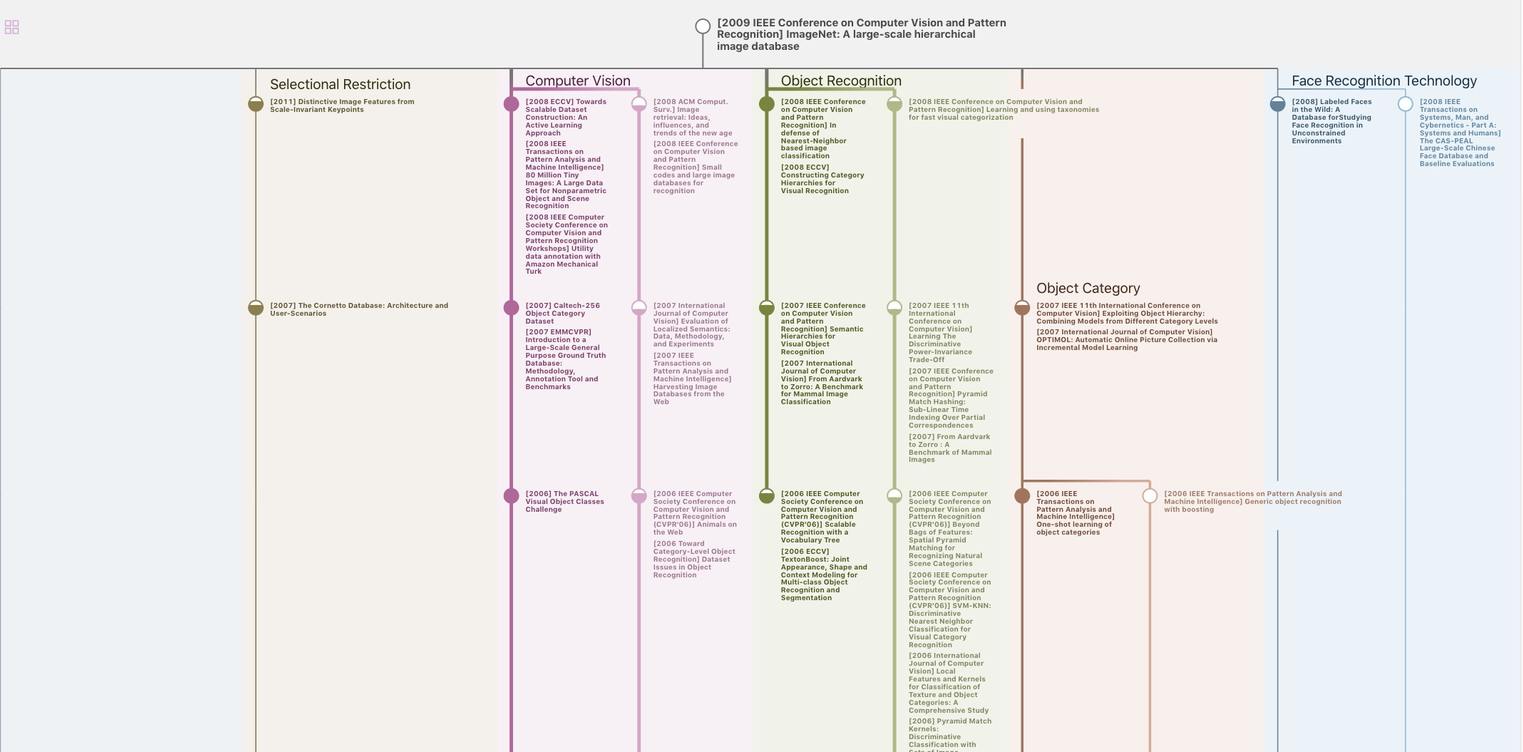
生成溯源树,研究论文发展脉络
Chat Paper
正在生成论文摘要