Dual Part Discovery Network for Zero-Shot Learning
Proceedings of the 30th ACM International Conference on Multimedia(2022)
摘要
ABSTRACTZero-Shot Learning (ZSL) aims to recognize unseen classes by transferring knowledge from seen classes. Recent methods focus on learning a common semantic space to align visual and attribute information. However, they always over-relied on provided attributes and ignored the category discriminative information that contributes to accurate unseen class recognition, resulting in weak transferability. To this end, we propose a novel Dual Part Discovery Network (DPDN) that considers both attribute and category discriminative information by discovering attribute-guided parts and category-guided parts simultaneously to improve knowledge transfer. Specifically, for attribute-guided parts discovery, DPDN can localize the regions with specific attribute information and significantly bridge the gap between visual and semantic information guided by the given attributes. For category-guided parts discovery, the local parts are explored to discover other important regions that bring latent crucial details ignored by attributes, with the guidance of adaptive category prototypes. To better mine the transferable knowledge, we impose class correlations constraints to regularize the category prototypes. Finally, attribute- and category-guided parts complement each other and provide adequate discriminative subtle information for more accurate unseen class recognition. Extensive experimental results demonstrate that DPDN can discover discriminative parts and outperform state-of-the-art methods on three standard benchmarks.
更多查看译文
关键词
dual part discovery network,learning,zero-shot
AI 理解论文
溯源树
样例
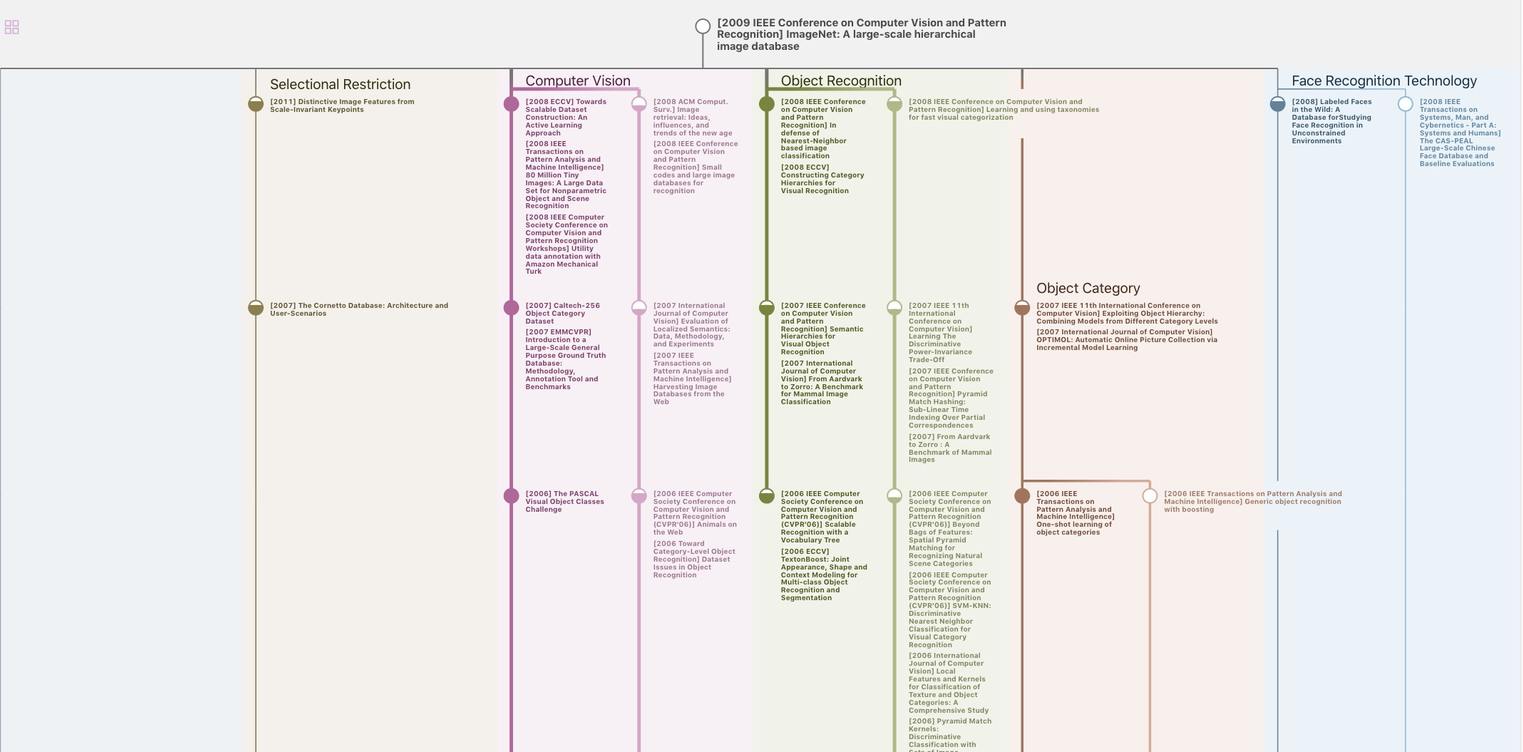
生成溯源树,研究论文发展脉络
Chat Paper
正在生成论文摘要