AdaMask: Enabling Machine-Centric Video Streaming with Adaptive Frame Masking for DNN Inference Offloading
ACM International Conference on Multimedia (MM)(2022)
摘要
ABSTRACTThis paper presents AdaMask, a machine-centric video streaming framework for remote deep neural network (DNN) inference. The objective is to optimize the accuracy of downstream DNNs, offloaded to a remote machine, by adaptively changing video compression control knobs at runtime. Our main contributions are twofold. First, we propose frame masking as an effective mechanism to reduce the bandwidth consumption of video stream, which only preserves regions that potentially contain objects of interest. Second, we design a new adaptation algorithm that achieves the Pareto-optimal tradeoff between accuracy and bandwidth by controlling the masked portions of frames together with conventional H.264 control knobs (eg. resolution). Through extensive evaluations on three sensing scenarios (dash camera, traffic surveillance, and drone), frame masking saves the bandwidth by up to 65% with < 1% accuracy degradation, and AdaMask improves the accuracy by up to 14% over the baselines against the network dynamics.
更多查看译文
关键词
dnn inference offloading,streaming,adaptive frame masking,machine-centric
AI 理解论文
溯源树
样例
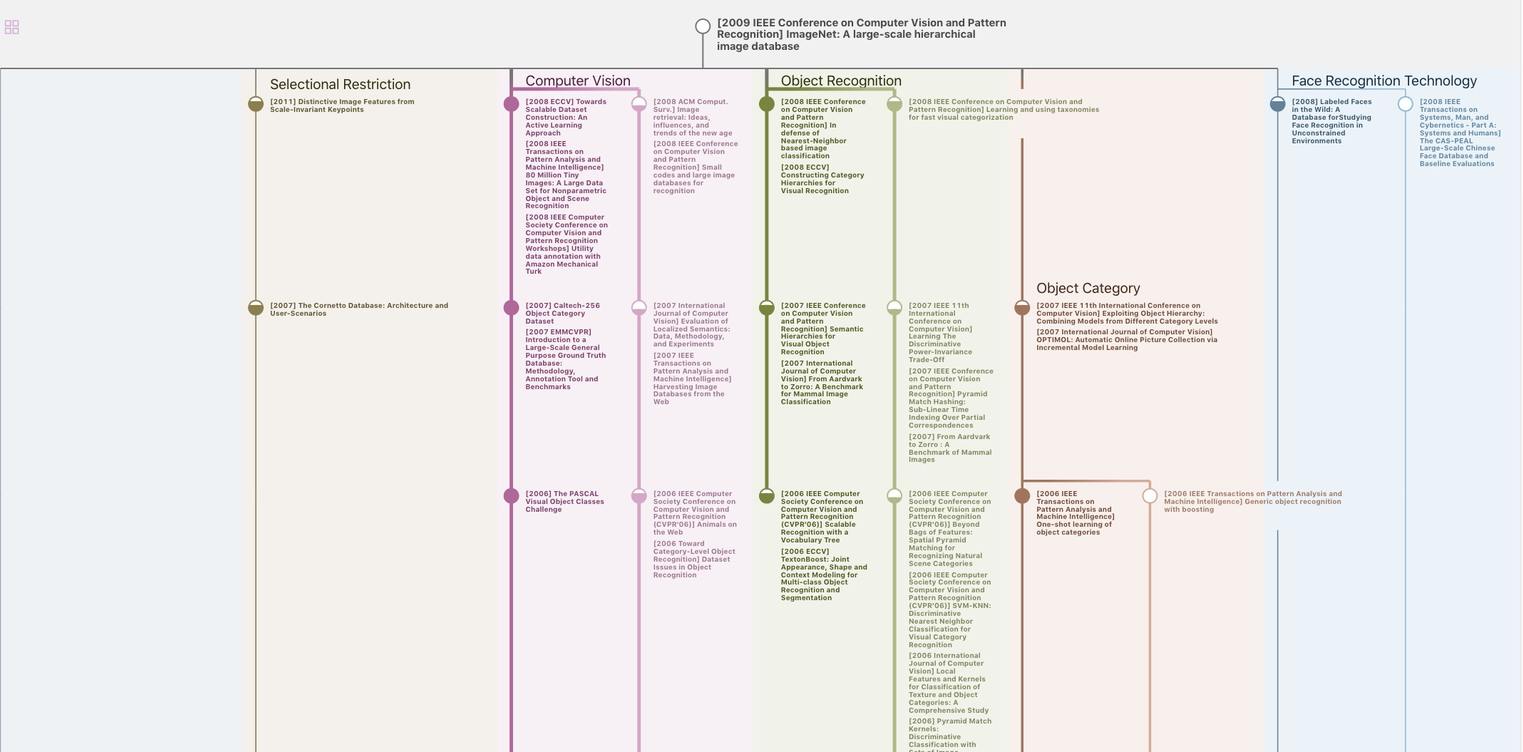
生成溯源树,研究论文发展脉络
Chat Paper
正在生成论文摘要