Disparity-based Stereo Image Compression with Aligned Cross-View Priors
Proceedings of the 30th ACM International Conference on Multimedia(2022)
摘要
With the wide application of stereo images in various fields, the research on stereo image compression (SIC) attracts extensive attention from academia and industry. The core of SIC is to fully explore the mutual information between the left and right images and reduce redundancy between views as much as possible. In this paper, we propose DispSIC, an end-to-end trainable deep neural network, in which we jointly train a stereo matching model to assist in the image compression task. Based on the stereo matching results (i.e. disparity), the right image can be easily warped to the left view, and only the residuals between the left and right views are encoded for the left image. A three-branch auto-encoder architecture is adopted in DispSIC, which encodes the right image, the disparity map and the residuals respectively. During training, the whole network can learn how to adaptively allocate bitrates to these three parts, achieving better rate-distortion performance at the cost of a lower disparity map bitrates. Moreover, we propose a conditional entropy model with aligned cross-view priors for SIC, which takes the warped latents of the right image as priors to improve the accuracy of the probability estimation for the left image. Experimental results demonstrate that our proposed method achieves superior performance compared to other existing SIC methods on the KITTI and InStereo2K datasets both quantitatively and qualitatively.
更多查看译文
关键词
stereo image compression,disparity-based,cross-view
AI 理解论文
溯源树
样例
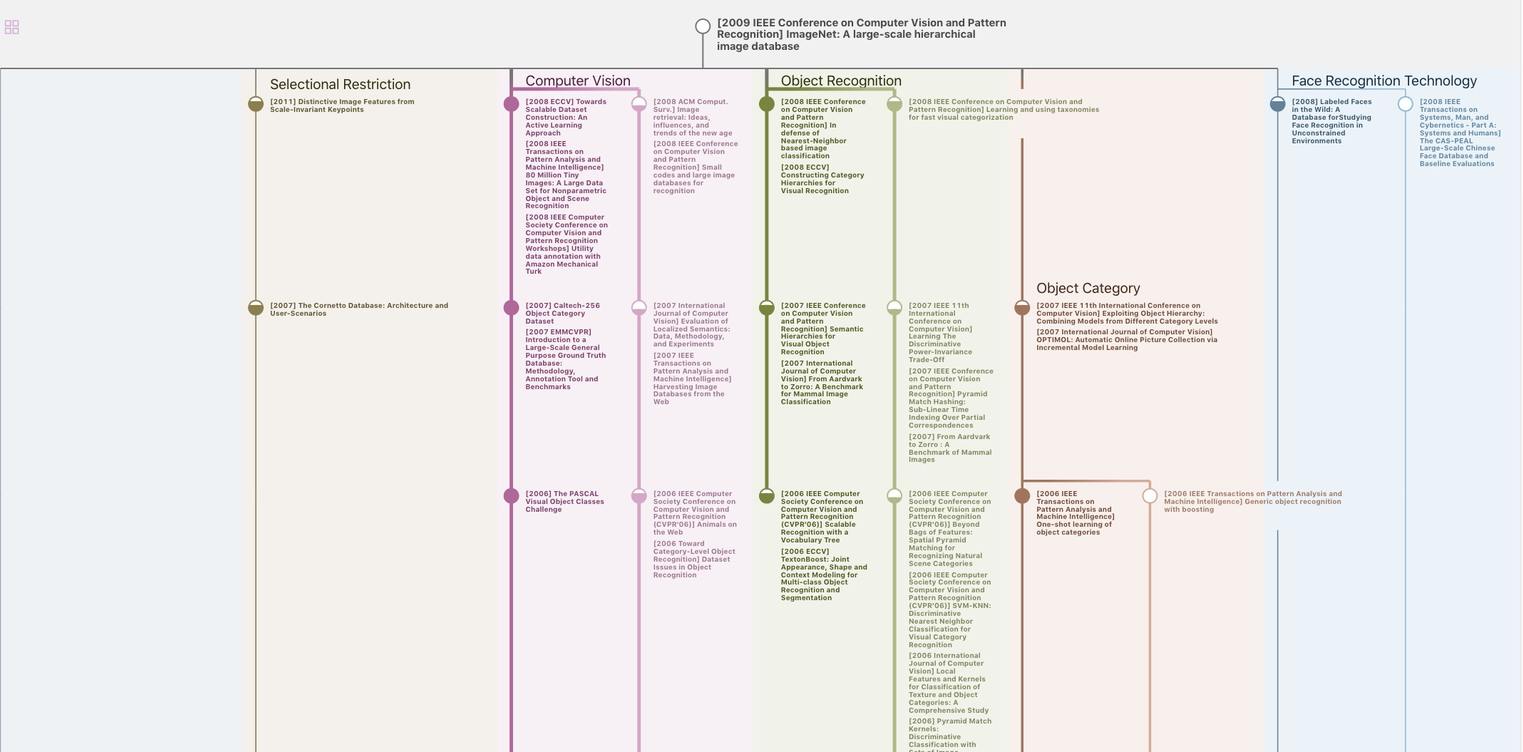
生成溯源树,研究论文发展脉络
Chat Paper
正在生成论文摘要