Recursive least mean dual p-power solution to the generalization of evolving fuzzy system under multiple noises
Information Sciences(2022)
摘要
During the last two decades, evolving fuzzy systems (EFSs) have attracted more and more attention owing to their capacity to self-adapt both system structures and parameters dynamically. Conventional EFSs optimize the consequent parameters using the recursive least square (RLS) alg orithm based on mean square error (MSE) criterion. It only works well in Gaussian noise or noise-free situations. In this paper, we further propose an alternative approach to update consequent parameters based on the novel least mean dual p-power (LMDP) error criterion. By contrast with the MSE criterion, the LMDP criterion has a stronger noise rejection ability on non-Gaussian noise environments. Moreover, the LMDP criterion extends the range of p value in conventional LMP criterion from positive integer to floating point for more fine-grained optimum solutions. Details in this work, the recursive solution of LMDP criterion is respectively derived from global learning and local learning to update consequent parameter and the theoretical stability analyses for both learning cases are proven in that the stability of our considered EFS is guaranteed. The experiments on various benchmark datasets and a real-world typhoon path prediction validate that the proposed method is more accurate and owns better generalization performance under multiple noise conditions.
更多查看译文
关键词
Recursive least mean dual p-power,Evolving fuzzy system,Non-Gaussian noises,Stability
AI 理解论文
溯源树
样例
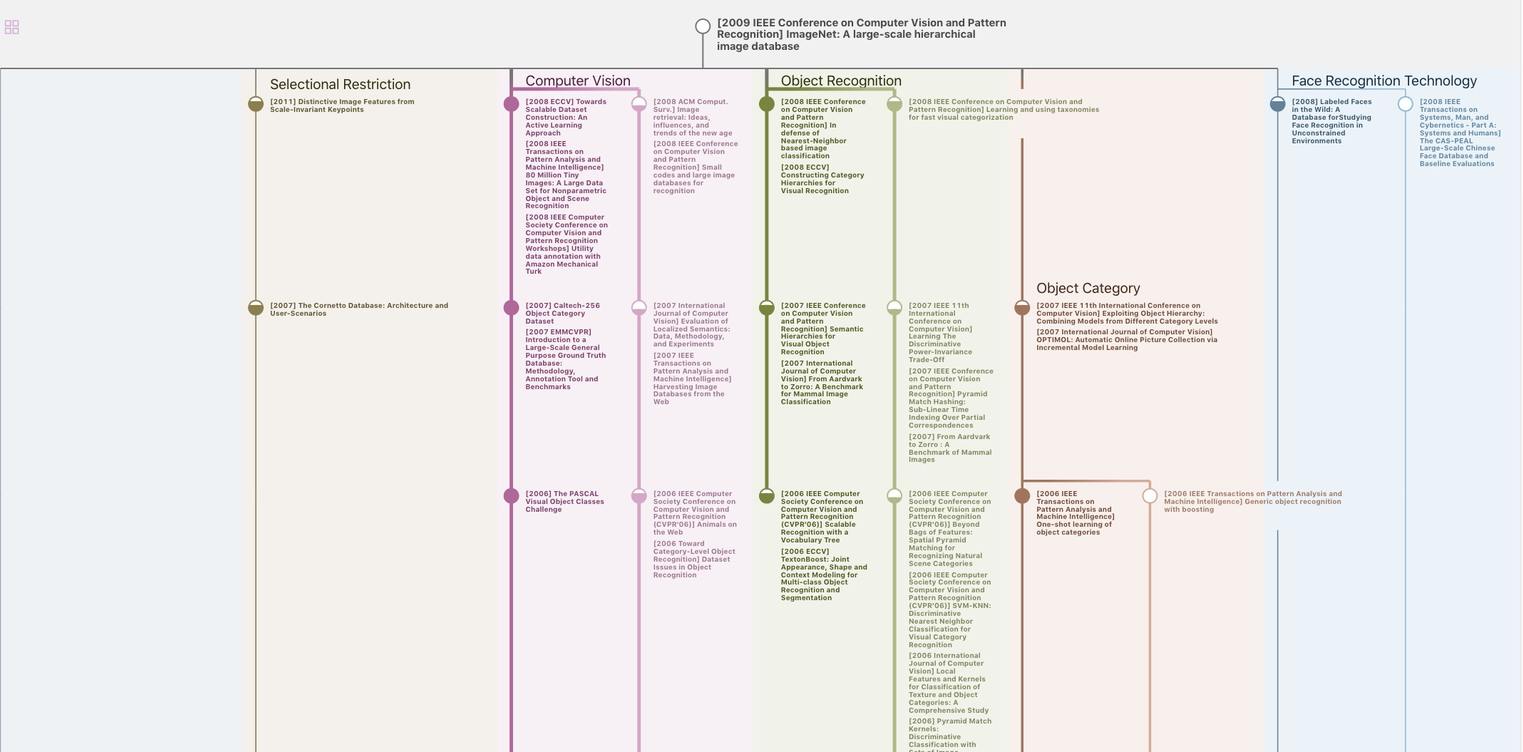
生成溯源树,研究论文发展脉络
Chat Paper
正在生成论文摘要