Multi-nomenclature, multi-resolution joint translation: an application to land-cover mapping.
Int. J. Geogr. Inf. Sci.(2023)
摘要
Land-use/land-cover (LULC) maps describe the Earth's surface with discrete classes at a specific spatial resolution. The chosen classes and resolution highly depend on peculiar uses, making it mandatory to develop methods to adapt these characteristics for a large range of applications. Recently, a convolutional neural network (CNN)-based method was introduced to take into account both spatial and geographical context to translate a LULC map into another one. However, this model only works for two maps: one source and one target. Inspired by natural language translation using multiple-language models, this article explores how to translate one LULC map into several targets with distinct nomenclatures and spatial resolutions. We first propose a new data set based on six open access LULC maps to train our CNN-based encoder-decoder framework. We then apply such a framework to convert each of these six maps into each of the others using our Multi-Landcover Translation network (MLCT-Net). Extensive experiments are conducted at a country scale (namely France). The results reveal that our MLCT-Net outperforms its semantic counterparts and gives on par results with mono-LULC models when evaluated on areas similar to those used for training. Furthermore, it outperforms the mono-LULC models when applied to totally new landscapes.
更多查看译文
关键词
Land-cover,land-use,translation,deep learning,harmonization
AI 理解论文
溯源树
样例
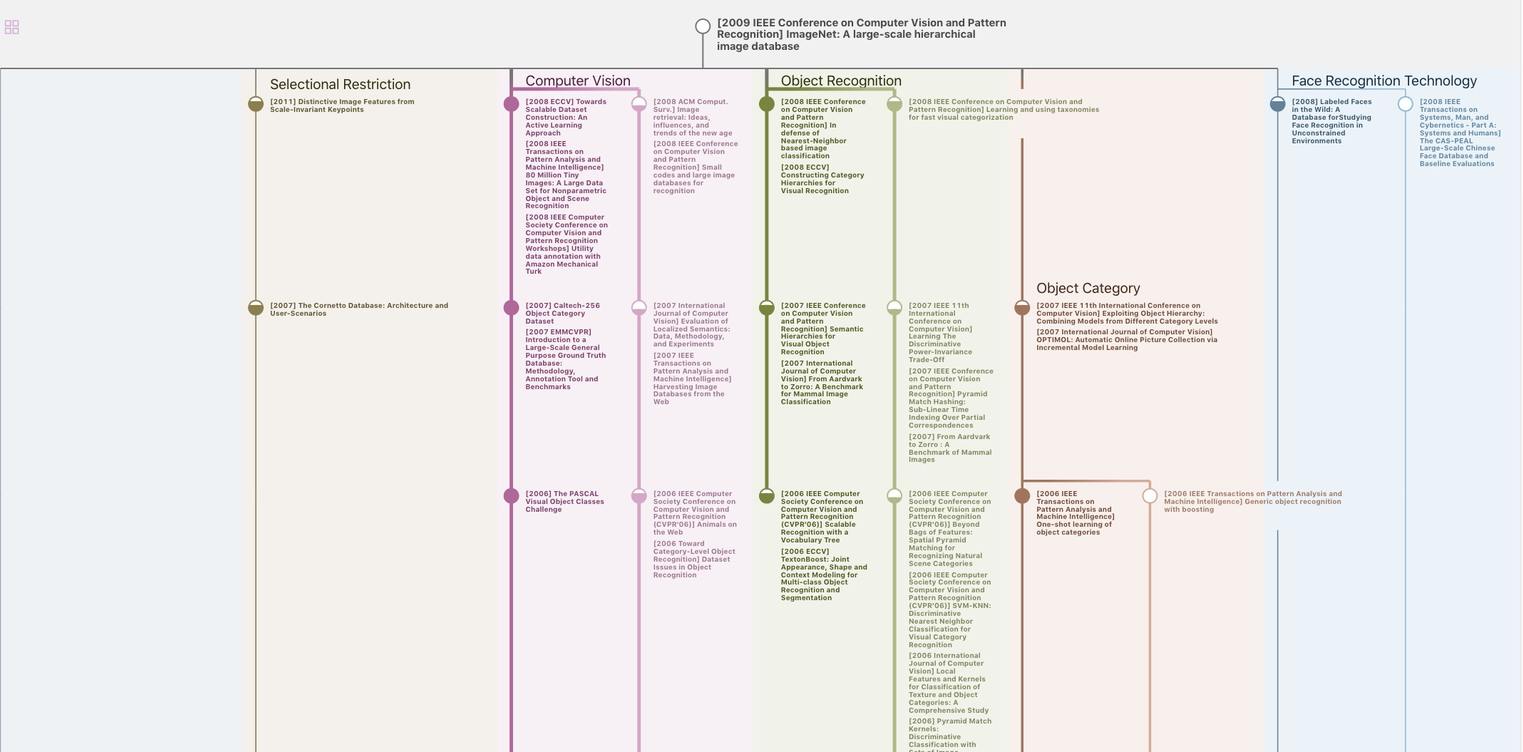
生成溯源树,研究论文发展脉络
Chat Paper
正在生成论文摘要