Deep learning for inflammatory diseases classification based on reflectance confocal microscopy.
Journal of the American Academy of Dermatology(2022)
摘要
To the Editor: Of the various patterns of dermatitis, interface dermatitis is most commonly associated with systemic illnesses,1Sontheimer R.D. Lichenoid tissue reaction/interface dermatitis: clinical and histological perspectives.J Invest Dermatol. 2009; 129: 1088-1099https://doi.org/10.1038/sj.jid.2009.4Crossref PubMed Scopus (0) Google Scholar and reflectance confocal microscopy (RCM) can be applied to the classification of inflammatory skin diseases.2Malciu A.M. Lupu M. Voiculescu V.M. Artificial intelligence-based approaches to reflectance confocal microscopy image analysis in dermatology.J Clin Med. 2022; 11: 429https://doi.org/10.3390/jcm11020429Crossref Scopus (9) Google Scholar Here, we developed and evaluated a deep convolutional neural network (CNN) model to distinguish interface dermatitis from other inflammatory skin diseases including psoriatic dermatitis and spongiotic dermatitis in RCM images. The system was evaluated with 329 RCM images at the dermoepidermal junction from 84 cases with interface dermatitis and 180 RCM images from 53 cases with other inflammatory skin diseases having similar clinical manifestations diagnosed at the Wuhan No. 1 Hospital between October 1, 2020, and June 1, 2021. All the RCM images were reviewed by a confocalist and an RCM expert, and images with significant and insignificant features were randomly selected for machine. Using image augmentation techniques to expand the data set, 2632 RCM images of interface dermatitis and 1440 RCM images of other inflammatory skin diseases were obtained. A deep learning-based model (Fig 1) was established, including the use of a VGG16-based network to extract features and the use of a three-layer fully connected network to classify the RCM images. The recognition accuracy of the trained CNN model was 97.78%. To validate this model, 75 newly collected RCM images were tested, including 38 images from 13 patients with interface dermatitis and 37 images from 12 patients with other inflammatory skin diseases. A questionnaire including these RCM images was sent to 12 dermatologists (2 expert dermatologists with more than 8 years experience of RCM reading and 10 general dermatologists trained with confocal reading on dermatitis) to compare the accuracy of the model with dermatologists. Receiver-operating characteristic curves were drawn, and the area under the curve was calculated. The accuracy of CNN was 94.67%, the sensitivity was 89.47%, the specificity was 100%, and the κ value was .893. The accuracy of CNN was comparable to the performance of the expert dermatologists (94.67 % vs 92.67%, χ2 = 0.118, P = .731) and superior to the general dermatologists (94.67% vs 75.73%, χ2 = 10.44, P = .001). The receiver-operating characteristic curve (Fig 2) shows that the sensitivity-specificity point of all 12 dermatologists lies below the curve, and the area under the curve was 0.997. Numerous deep learning technologies have been developed in recent years to provide a more objective approach to RCM image analysis, but little work has involved the recognition of inflammatory skin disease by RCM.2Malciu A.M. Lupu M. Voiculescu V.M. Artificial intelligence-based approaches to reflectance confocal microscopy image analysis in dermatology.J Clin Med. 2022; 11: 429https://doi.org/10.3390/jcm11020429Crossref Scopus (9) Google Scholar Our results demonstrated that deep learning combined with RCM can effectively identify interface dermatitis and distinguish it from other inflammatory skin diseases, and the proposed model may be comparable to the performance of the expert dermatologists. This finding is consistent with previous studies.3Tschandl P. Rinner C. Apalla Z. et al.Human-computer collaboration for skin cancer recognition.Nat Med. 2020; 26: 1229-1234https://doi.org/10.1038/s41591-020-0942-0Crossref PubMed Scopus (227) Google Scholar Artificial intelligence–based support of clinical decision-making improves diagnostic accuracy over that of either artificial intelligence or physicians alone, and the least experienced clinicians gain the most from the human-computer collaboration.3Tschandl P. Rinner C. Apalla Z. et al.Human-computer collaboration for skin cancer recognition.Nat Med. 2020; 26: 1229-1234https://doi.org/10.1038/s41591-020-0942-0Crossref PubMed Scopus (227) Google Scholar In the future, we will expand the data set to include other inflammatory skin diseases. None disclosed.
更多查看译文
AI 理解论文
溯源树
样例
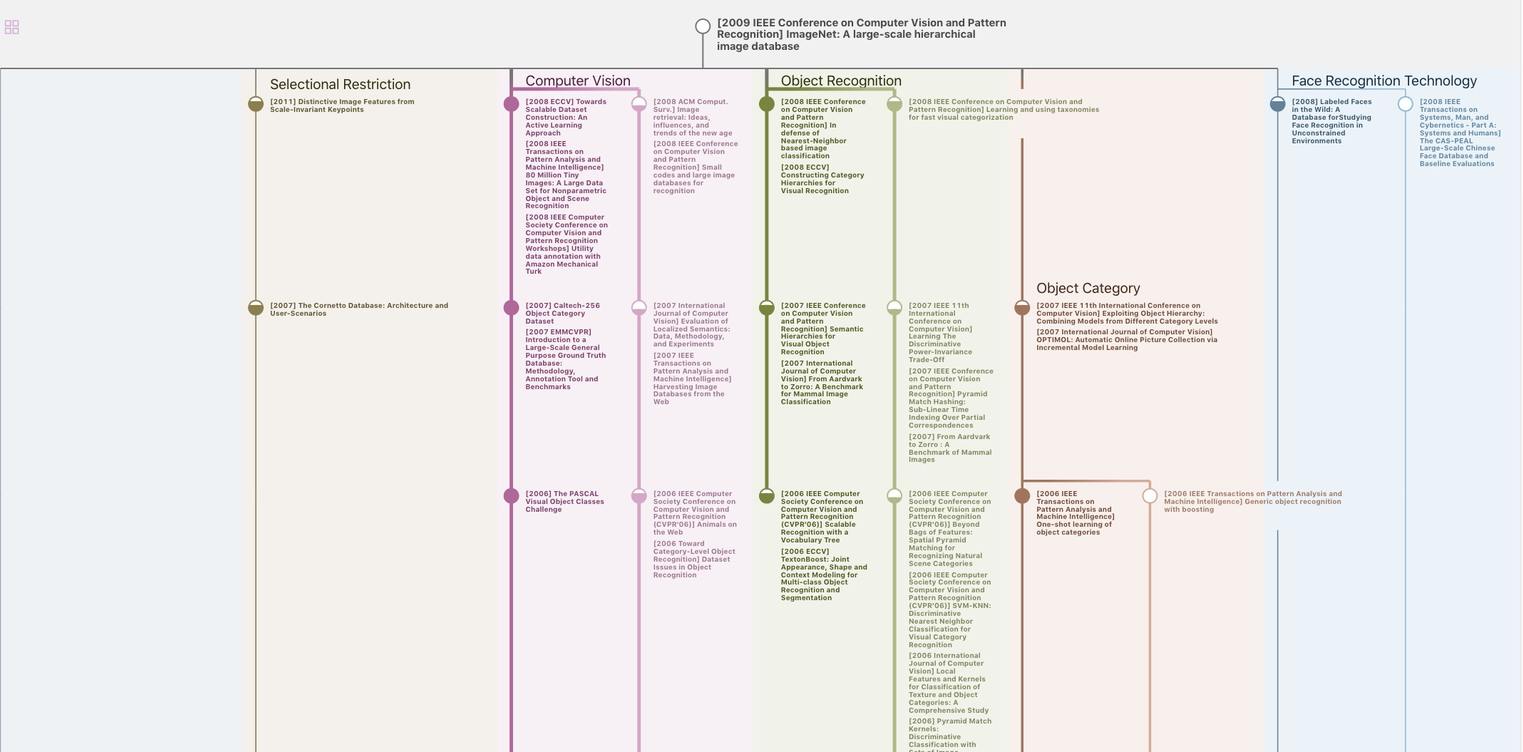
生成溯源树,研究论文发展脉络
Chat Paper
正在生成论文摘要