Hybrid model of a physics-based model and machine learning for real-time estimation of unmeasurable parts: Mapping from measurable to unmeasurable variables
Ocean Engineering(2022)
摘要
In some dynamic systems, such as offshore drilling, it is important to estimate the behaviour of unmeasurable parts in real-time, using measurable data. This article proposes a novel hybrid model of a physics-based model and machine learning (proposed model), which maps measurable variables to unmeasurable variables. The effectiveness of the proposed model was verified by comparing it with the conventional physics-based model and grey-box model, using an offshore drilling system as a case study application. As a result, it was confirmed that the proposed model could relax the required conditions of the measured data’s sampling period and time length when compared to the conventional model by directly inputting the measurable data. Additionally, we confirmed that the proposed model solves the time cost for parameter adjustment in the grey-box model and is superior in real-time performance. The proposed model was also shown to be robust against measurement errors. Thus, it is demonstrated that the proposed model has a high performance when the real phenomena can be represented within the physics-based model’s uncertainty of the initial states and parameters. The proposed model is expected to be suitable for various dynamic systems in addition to offshore drilling systems.
更多查看译文
关键词
Hybrid modelling,Grey-box model,Machine learning,Offshore drilling
AI 理解论文
溯源树
样例
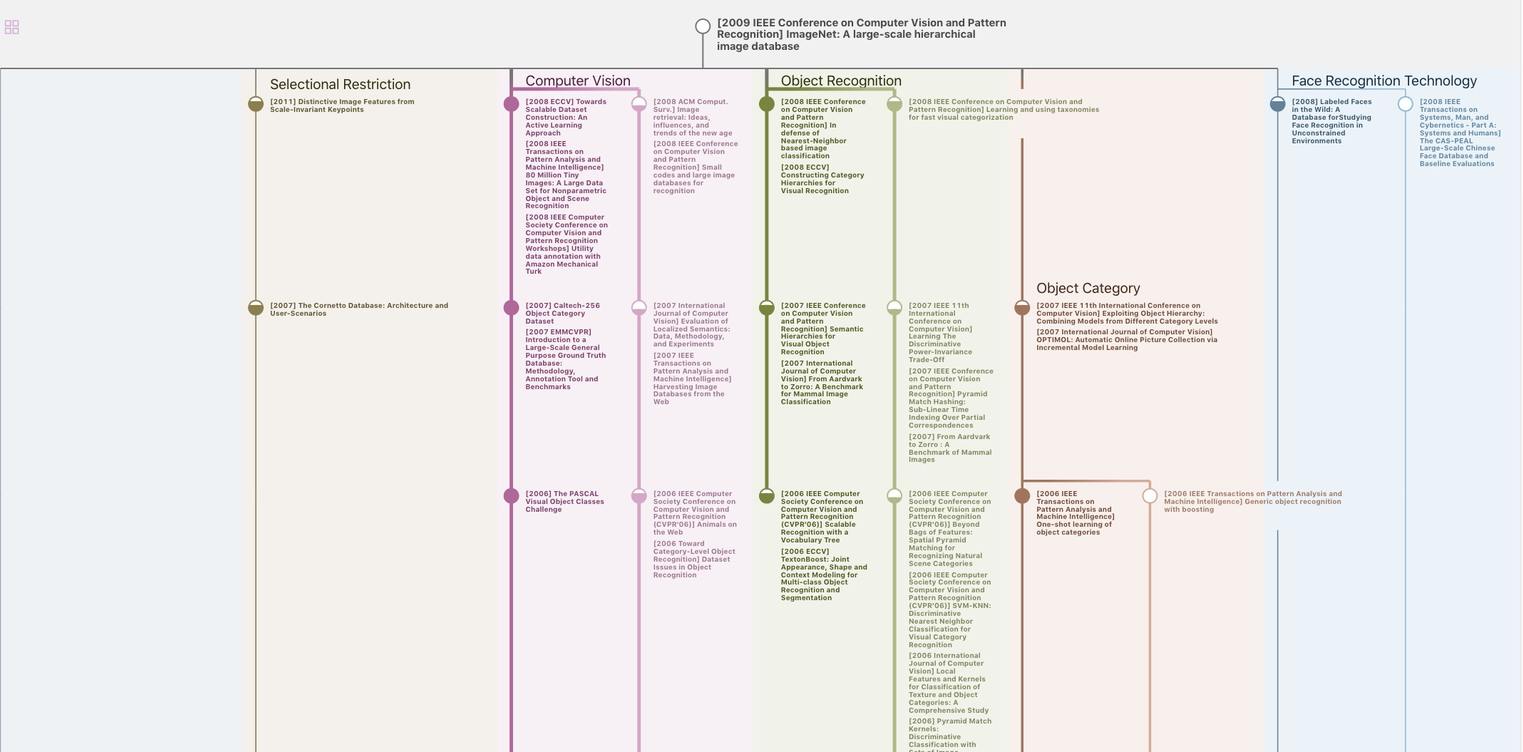
生成溯源树,研究论文发展脉络
Chat Paper
正在生成论文摘要