VLDeformer: Vision–Language Decomposed Transformer for fast cross-modal retrieval
Knowledge-Based Systems(2022)
摘要
Cross-model retrieval has emerged as one of the most important upgrades for text-only search engines (SE). Recently, with powerful representation for pairwise text–image inputs via early interaction, the accuracy of vision–language (VL) transformers has outperformed existing methods for text–image retrieval. However, when the same paradigm is used for inference, the efficiency of the VL transformers is still too low to be applied in a real cross-modal SE. Inspired by the mechanism of human learning and using cross-modal knowledge, this paper presents a novel Vision–Language Decomposed Transformer (VLDeformer), which greatly increases the efficiency of VL transformers while maintaining their outstanding accuracy. By the proposed method, the cross-model retrieval is separated into two stages: the VL transformer learning stage, and the VL decomposition stage. The latter stage plays the role of single modal indexing, which is to some extent like the term indexing of a text SE. The model learns cross-modal knowledge from early-interaction pre-training and is then decomposed into an individual encoder. The VLDeformer requires only small target datasets for supervision and achieves both 1000+ times acceleration and less than 0.6% average recall drop. VLDeformer also outperforms state-of-the-art visual-semantic embedding methods on COCO and Flickr30k.11Code is available at .
更多查看译文
关键词
Image retrieval,Cross-modal retrieval,Visual-semantic embedding,Similarity search,Vision and language
AI 理解论文
溯源树
样例
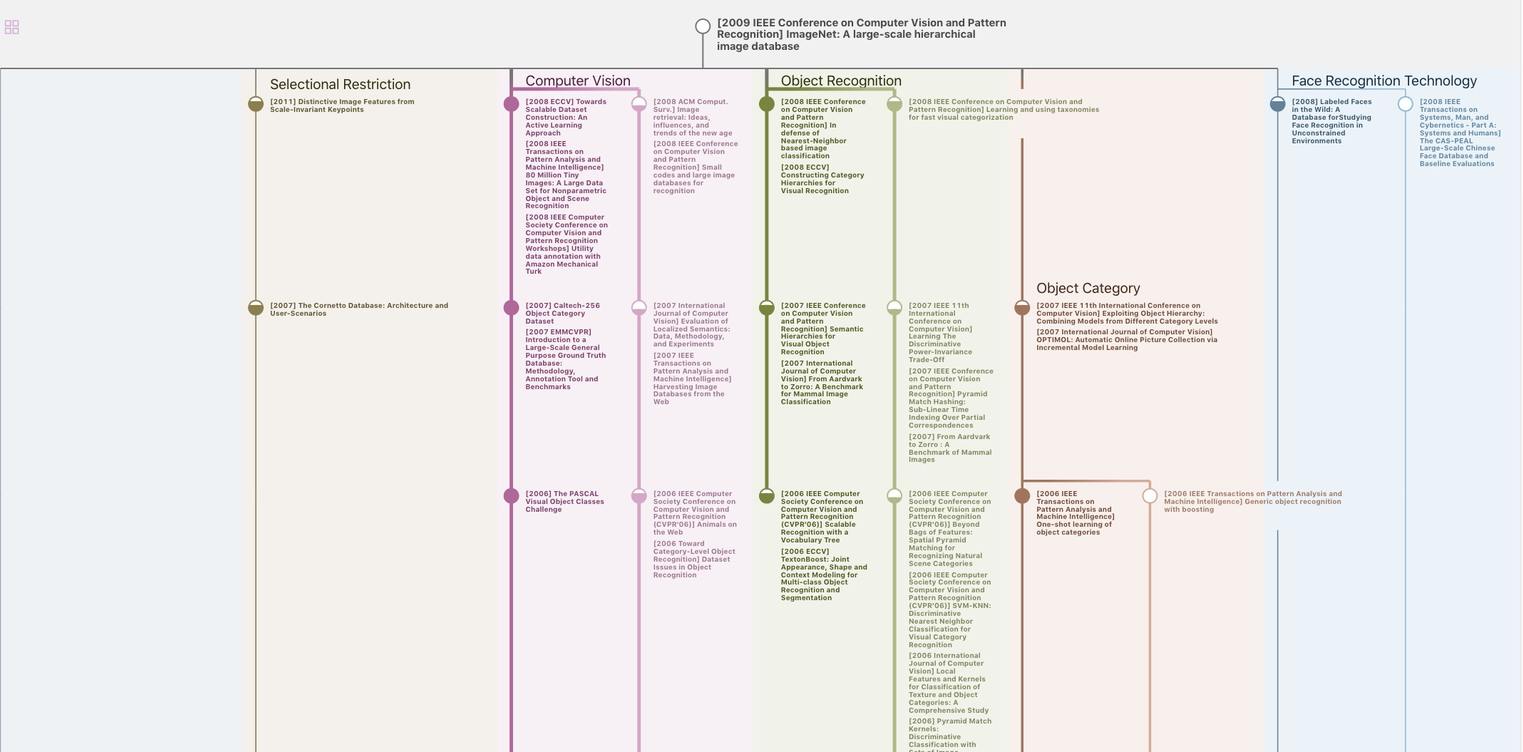
生成溯源树,研究论文发展脉络
Chat Paper
正在生成论文摘要