Lightweight Network with Variable Asymmetric Rebalancing Strategy for Small and Imbalanced Fault Diagnosis
Machines(2022)
摘要
Deep learning-related technologies have achieved remarkable success in the field of intelligent fault diagnosis. Nevertheless, the traditional intelligent diagnosis methods are often based on the premise of sufficient annotation signals and balanced distribution of classes, and the model structure is so complex that it requires huge computational resources. To this end, a lightweight class imbalanced diagnosis framework based on a depthwise separable Laplace-wavelet convolution network with variable-asymmetric focal loss (DSLWCN-VAFL) is established. Firstly, a branch with few parameters for time-frequency feature extraction is designed by integrating wavelet and depthwise separable convolution. It is combined with the branch of regular convolution that fully learns time-domain features to jointly capture abundant discriminative features from limited samples. Subsequently, a new asymmetric soft-threshold loss, VAFL, is designed, which reasonably rebalances the contributions of distinct samples during the model training. Finally, experiments are conducted on the data of bearing and gearbox, which demonstrate the superiority of the DSLWCN-VAFL algorithm and its lightweight diagnostic framework in handling class imbalanced data.
更多查看译文
关键词
fault diagnosis,class imbalanced data,small sample,Laplace wavelet,loss function
AI 理解论文
溯源树
样例
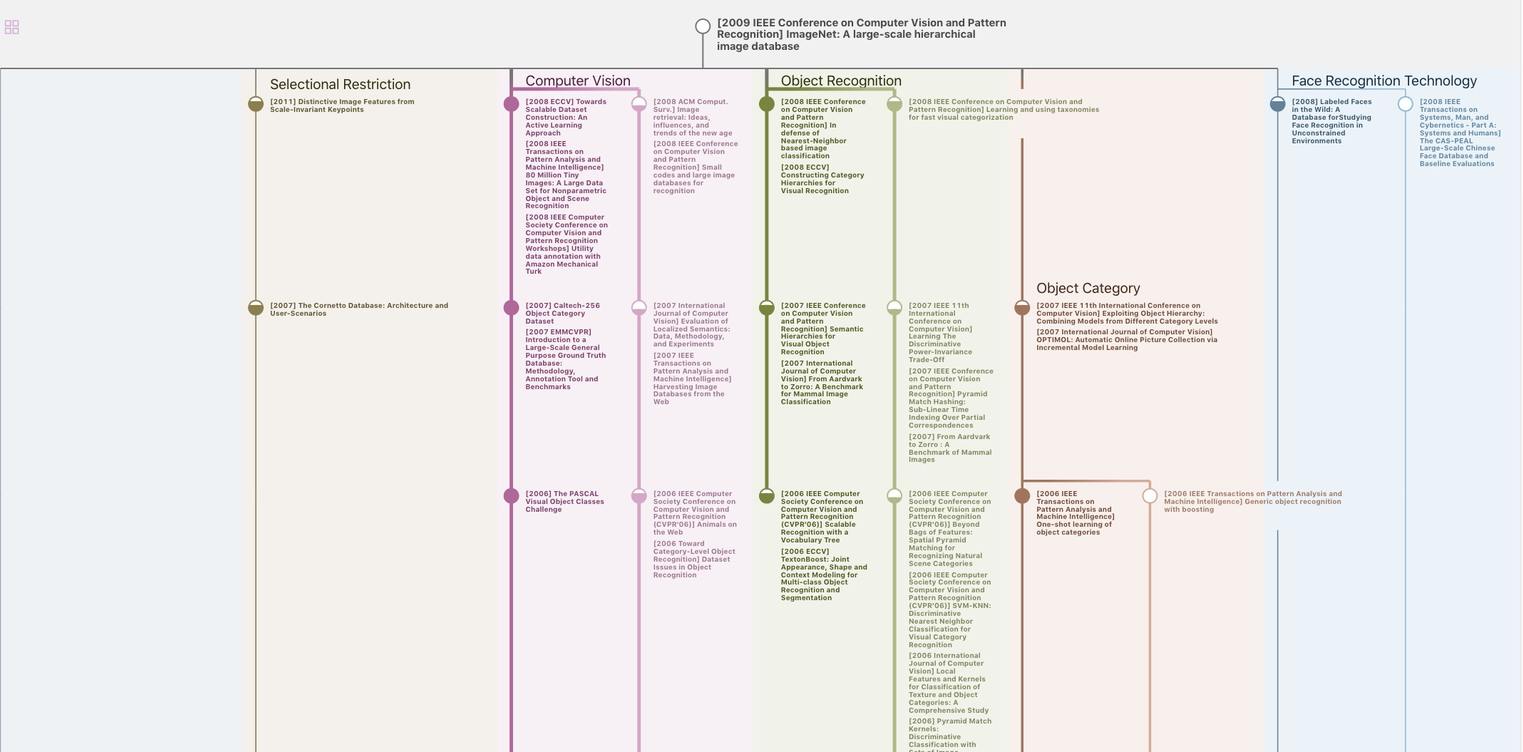
生成溯源树,研究论文发展脉络
Chat Paper
正在生成论文摘要