Framing Network Flow for Anomaly Detection Using Image Recognition and Federated Learning
Electronics(2022)
摘要
The intrusion detection system (IDS) must be able to handle the increase in attack volume, increasing Internet traffic, and accelerating detection speeds. Network flow feature (NTF) records are the input of flow-based IDSs that are used to determine whether network traffic is normal or malicious in order to avoid IDS from difficult and time-consuming packet content inspection processing since only flow records are examined. To reduce computational power and training time, this paper proposes a novel pre-processing method merging a specific amount of NTF records into frames, and frame transformation into images. Federated learning (FL) enables multiple users to share the learned models while maintaining the privacy of their training data. This research suggests federated transfer learning and federated learning methods for NIDS employing deep learning for image classification and conducting tests on the BOUN DDoS dataset to address the issue of training data privacy. Our experimental results indicate that the proposed Federated transfer learning (FTL) and FL methods for training do not require data centralization and preserve participant data privacy while achieving acceptable accuracy in DDoS attack identification: FTL (92.99%) and FL (88.42%) in comparison with Traditional transfer learning (93.95%).
更多查看译文
关键词
network intrusion detection, deep learning, federated learning, image representation
AI 理解论文
溯源树
样例
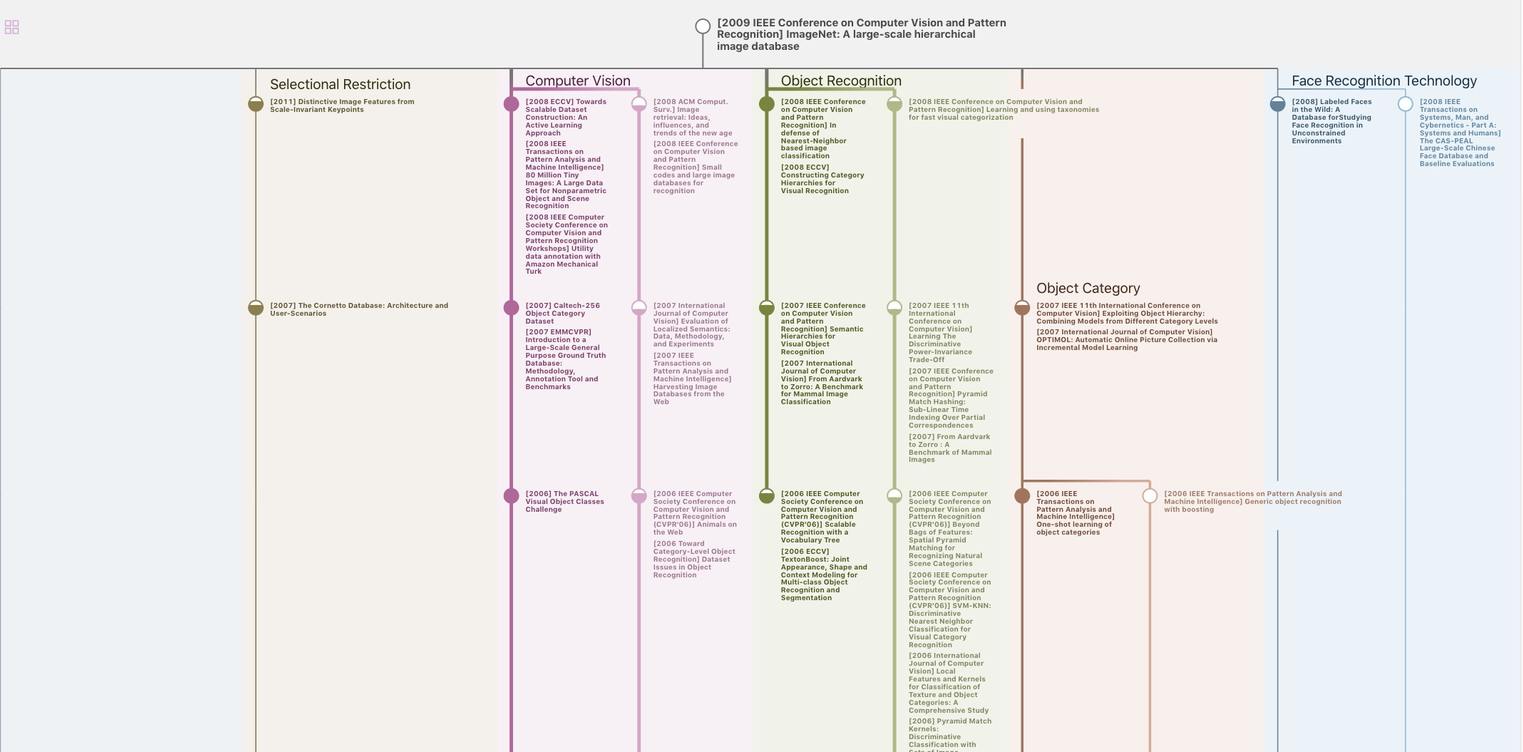
生成溯源树,研究论文发展脉络
Chat Paper
正在生成论文摘要