Compressed sensing in photonics: tutorial
Journal of the Optical Society of America B(2023)
摘要
Traditional optical imaging and sensing methods capture signals of interest by direct sampling in the domain of interest such as by forming images on pixelated camera sensors or by regular temporal sampling of a waveform. These methods are indispensable in our daily lives and for many scientific disciplines such as microscopy in biology and spectroscopy in chemistry. Using these approaches, the sampling constraints and their impact on the bounds on signal fidelity are well understood through the Nyquist-Shannon sampling theorem. However, the problems of modern science require ever increasing amounts of data at unprecedented temporal and spatial scales and res-olutions, which challenges the limits of traditional sensing. The increased availability of computational power combined with recent strides in signal processing promise to surpass many of the problems associated with tradi-tional sensing methods through computational imaging and sensing methods. Within the realm of computational sensing, compressed sensing (CS), in particular, has enabled the capture of signals with lower sampling resources than traditionally required by the Nyquist-Shannon sampling theorem using prior information such as sparsity. In this tutorial, we focus on the operation and impact of such sub-Nyquist sampling schemes through the use of CS in photonic sensing and imaging systems. Emphasis is placed on intuition, but mathematical results are derived or cited where appropriate. Finally, we highlight several applications in macroscopic and microscopic imaging, spectroscopy, and microwave photonic sensing.(c) 2022 Optica Publishing Group
更多查看译文
关键词
compressed sensing,photonics
AI 理解论文
溯源树
样例
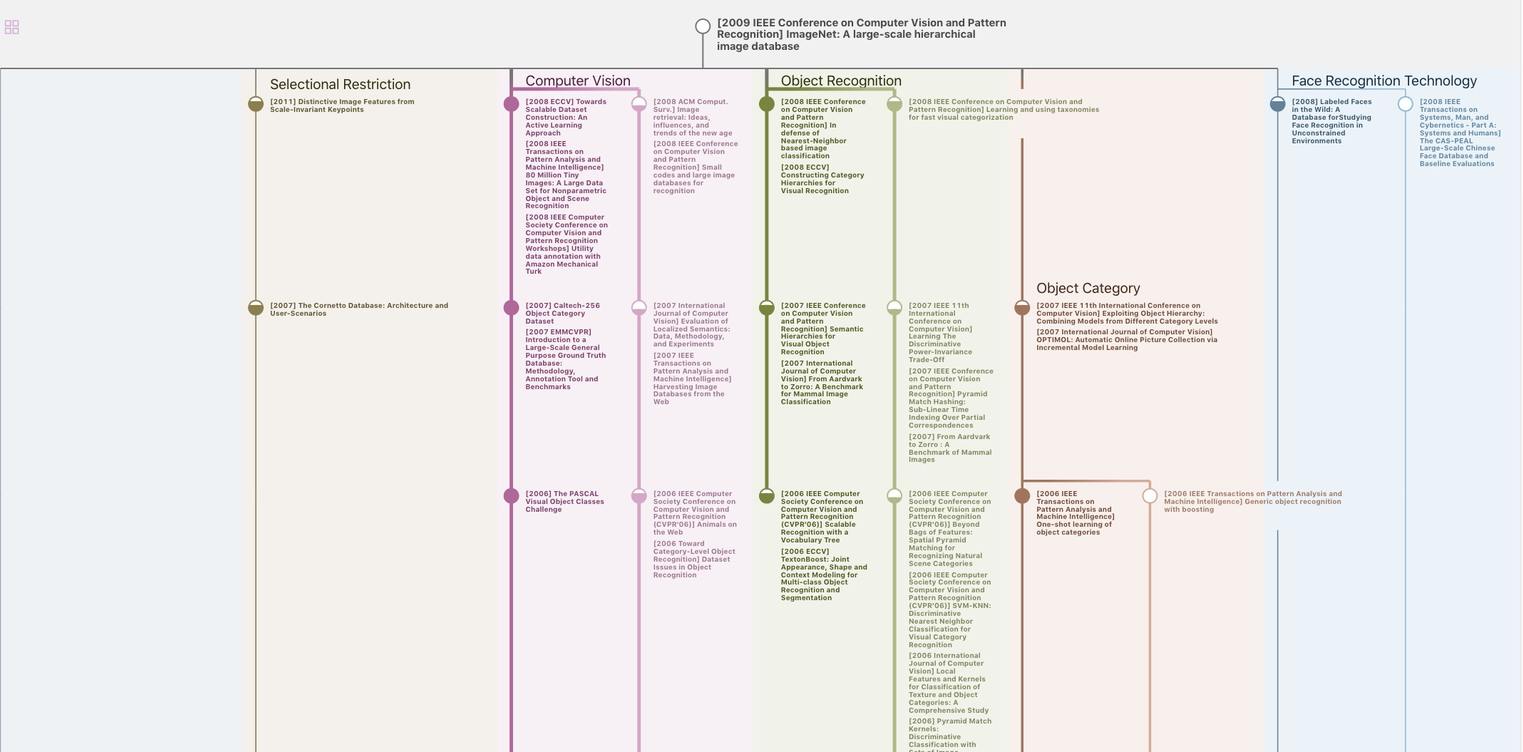
生成溯源树,研究论文发展脉络
Chat Paper
正在生成论文摘要