Scalability investigation of Double Deep Q Learning for factory layout planning
Procedia CIRP(2022)
摘要
Factory layout planning is a recurring and important process since an inadequately planned layout can significantly impede the operation of a factory. A time-efficient and automated planning method is especially important as shortened product lifecycles, changing technologies, and market requirements lead to changes in the production program, which further require the re-planning of the factory layout. Reinforcement learning (RL) has proven to be a suitable approach to support decision-making in complex layout planning problems. However, recent studies only consider comparably small and exemplary problem statements with a maximum of 4 functional units. In consequence, these approaches are insufficient for industrial applications in which numerous functional units are considered. Regarding this background, this paper investigates the scalability of a RL-based planning approach, in which a Double Deep Q Learning agent is used to solve a layout planning task for a small batch production with 20 functional units. Considering that recent approaches lack scalability, this paper presents a new state representation in combination with an action masking method that optimizes the action selection process to ensure the scalability of the approach and reduce the training time. After the training, the algorithm can generate an optimized factory layout by reducing the resulting transportation time for industrial relevant problem sizes.
更多查看译文
关键词
Reinforcement learning,Machine learning,Layout planning,Factory planning,Optimization
AI 理解论文
溯源树
样例
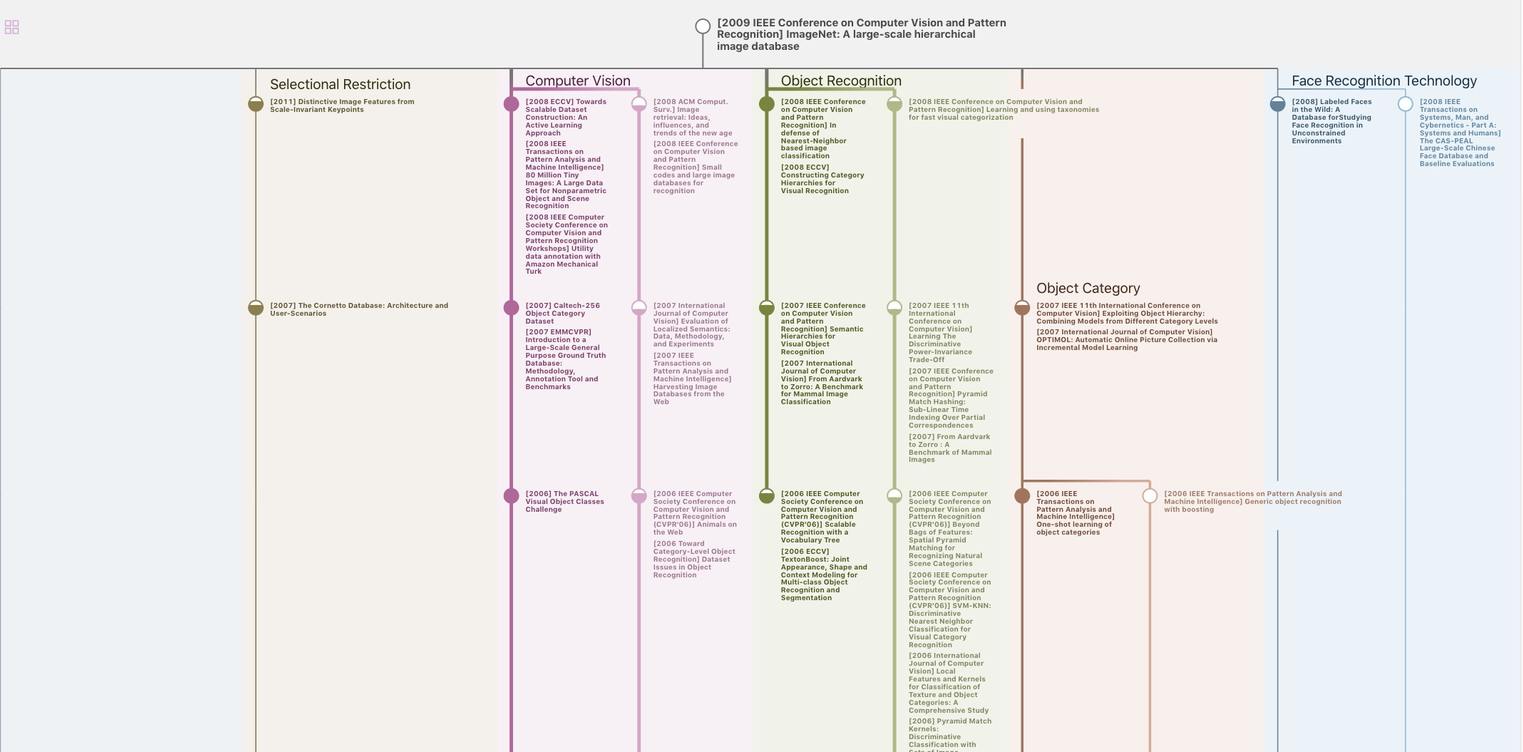
生成溯源树,研究论文发展脉络
Chat Paper
正在生成论文摘要