SimPer: Simple Self-Supervised Learning of Periodic Targets
ICLR 2023(2022)
摘要
From human physiology to environmental evolution, important processes in nature often exhibit meaningful and strong periodic or quasi-periodic changes. Due to their inherent label scarcity, learning useful representations for periodic tasks with limited or no supervision is of great benefit. Yet, existing self-supervised learning (SSL) methods overlook the intrinsic periodicity in data, and fail to learn representations that capture periodic or frequency attributes. In this paper, we present SimPer, a simple contrastive SSL regime for learning periodic information in data. To exploit the periodic inductive bias, SimPer introduces customized augmentations, feature similarity measures, and a generalized contrastive loss for learning efficient and robust periodic representations. Extensive experiments on common real-world tasks in human behavior analysis, environmental sensing, and healthcare domains verify the superior performance of SimPer compared to state-of-the-art SSL methods, highlighting its intriguing properties including better data efficiency, robustness to spurious correlations, and generalization to distribution shifts. Code and data are available at: https://github.com/YyzHarry/SimPer.
更多查看译文
关键词
Periodic learning,Self-supervised learning,Representation learning,Periodic targets,Periodicity
AI 理解论文
溯源树
样例
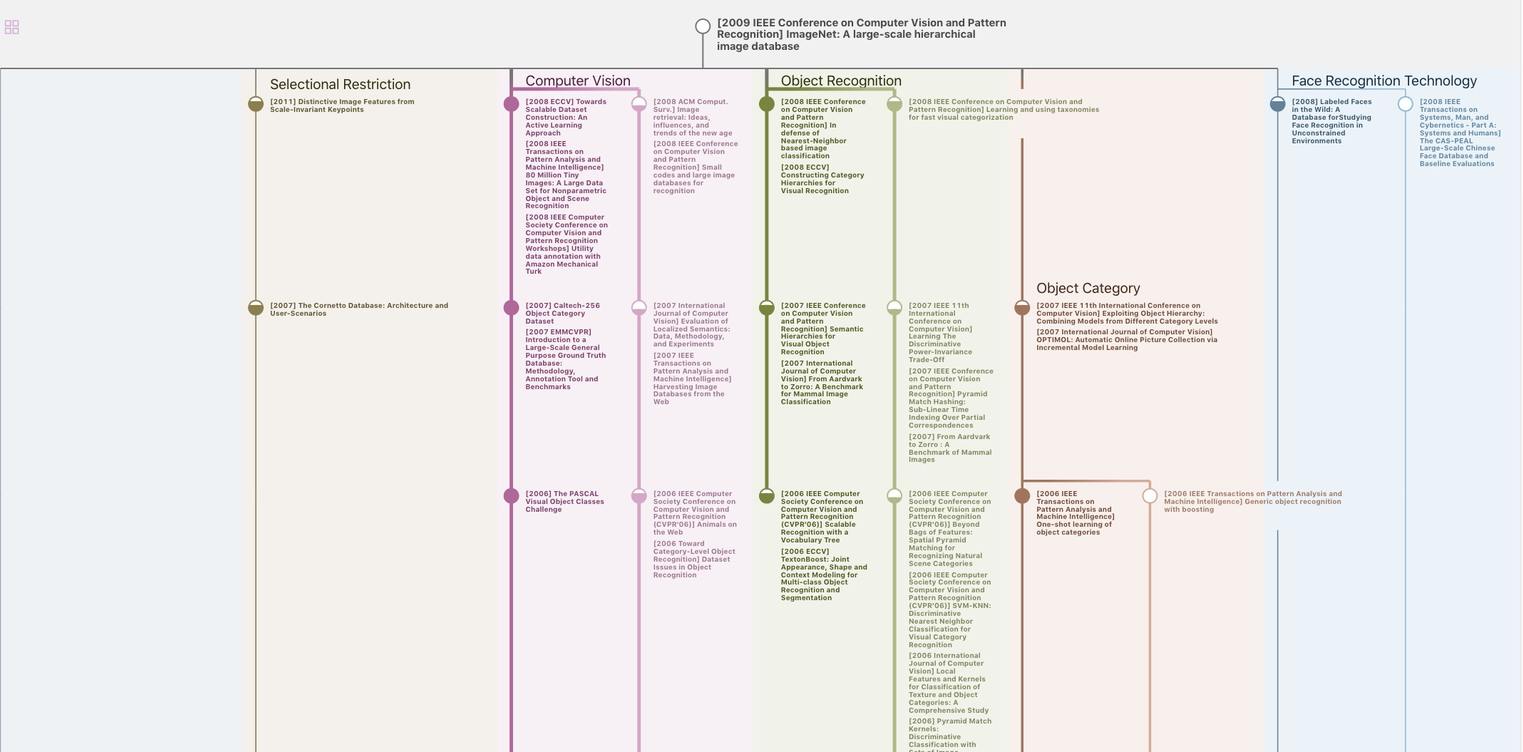
生成溯源树,研究论文发展脉络
Chat Paper
正在生成论文摘要