Explainable Abuse Detection as Intent Classification and Slot Filling.
Trans. Assoc. Comput. Linguistics(2022)
摘要
To proactively offer social media users a safe online experience, there is a need for systems that can detect harmful posts and promptly alert platform moderators. In order to guarantee the enforcement of a consistent policy, moderators are provided with detailed guidelines. In contrast, most state-of-the-art models learn what abuse is from labelled examples and as a result base their predictions on spurious cues, such as the presence of group identifiers, which can be unreliable. In this work we introduce the concept of policy-aware abuse detection, abandoning the unrealistic expectation that systems can reliably learn which phenomena constitute abuse from inspecting the data alone. We propose a machine-friendly representation of the policy that moderators wish to enforce, by breaking it down into a collection of intents and slots. We collect and annotate a dataset of 3,535 English posts with such slots, and show how architectures for intent classification and slot filling can be used for abuse detection, while providing a rationale for model decisions.
更多查看译文
关键词
explainable abuse detection,intent classification
AI 理解论文
溯源树
样例
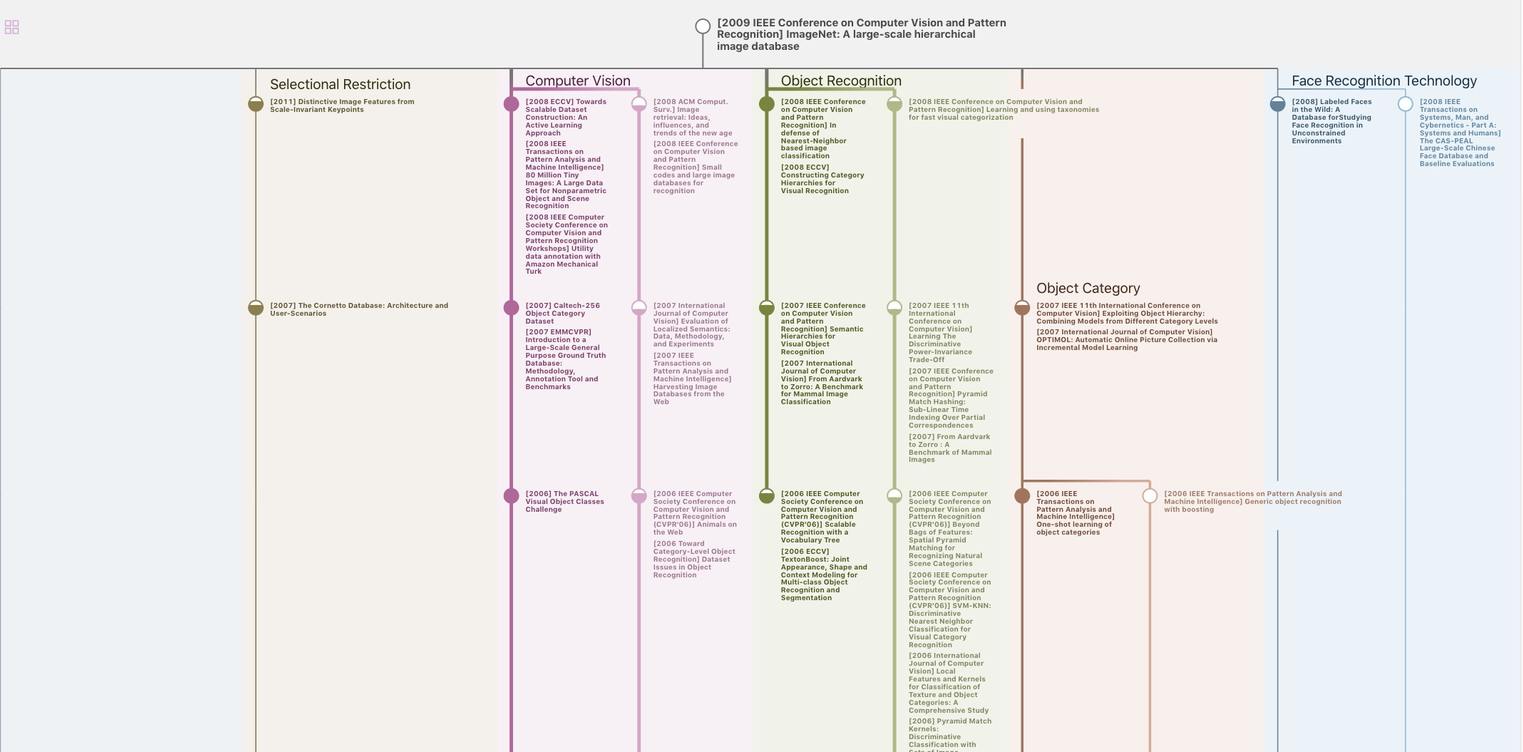
生成溯源树,研究论文发展脉络
Chat Paper
正在生成论文摘要