Adaptive evolution enhanced physics-informed neural networks for time-variant health prognosis of lithium-ion batteries
Journal of Power Sources(2023)
摘要
The accurate health prognosis of lithium-ion batteries is crucial for the safe and stable long-term operation of electric equipment. However, conventional time-invariant prognosis methods cannot capture time-variant features due to their deficient utilization of dynamic physics information. This paper presents an adaptive evolution enhanced physics-informed neural network-based time-variant health prognosis framework for lithium-ion batteries. A long short-term memory neural network (LSTM NN) model with a dynamic sliding window, informed by physical information derived from an electrochemical-thermal-solid electrolyte interphase formation simulation, is established for the health prognosis focus on state of health (SOH) and remaining useful life (RUL). An adaptive model evolution method is developed to reconstruct the structure of the LSTM NN to automatically and synchronously evolve its generalization ability, making it more sensitive to the implicit dynamic features during long-term prognoses. Eventually, the effectiveness and advancement of the proposed methods are verified on battery degradation dataset and in battery charge–discharge experiments. The results show that the proposed method provides high prognosis accuracy under different charging and discharging conditions and can improve health prognosis accuracy through adaptive model evolution during long-term operations.
更多查看译文
关键词
Physics-informed neural networks,Health prognosis,Adaptive evolution,Lithium-ion battery,Long short-term memory,Kullback–Leibler divergence
AI 理解论文
溯源树
样例
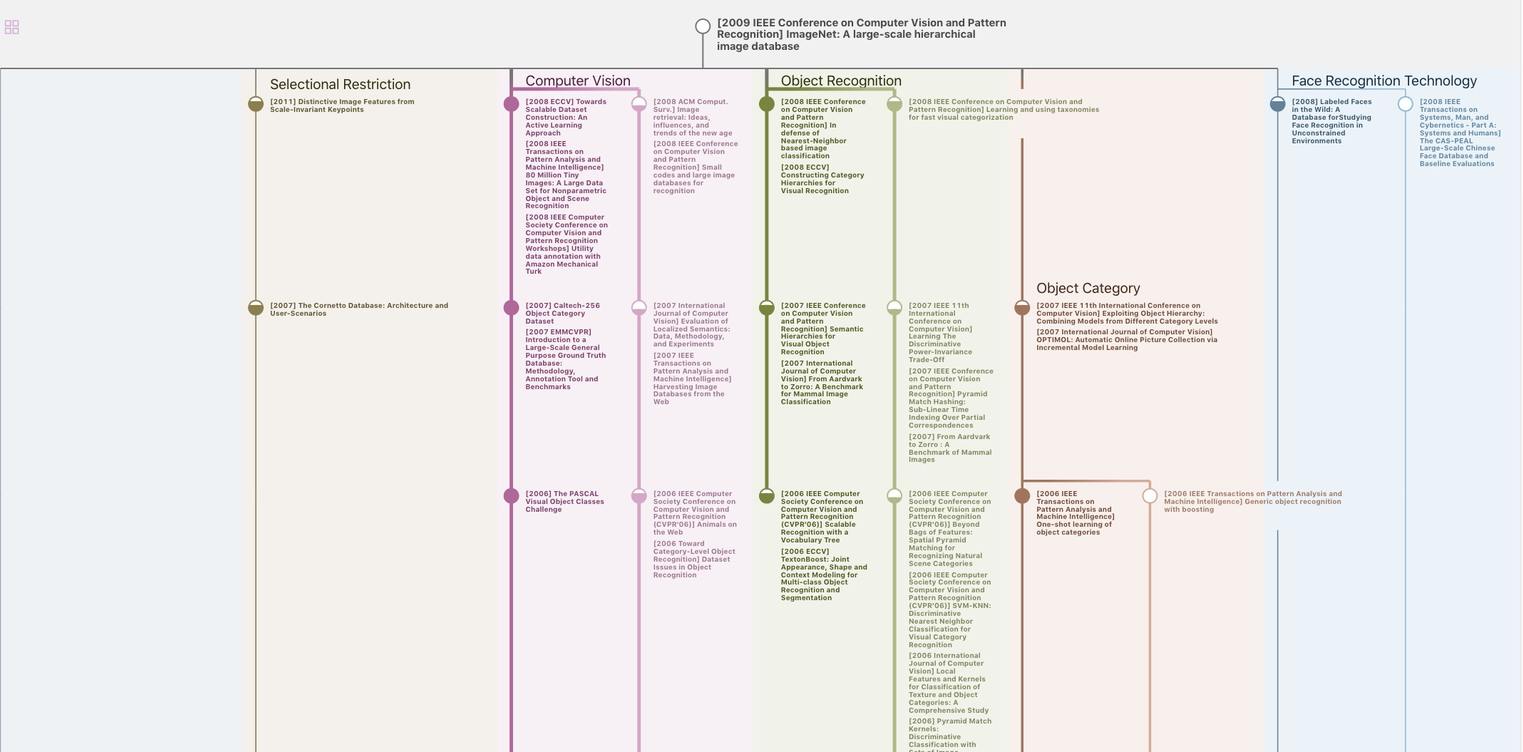
生成溯源树,研究论文发展脉络
Chat Paper
正在生成论文摘要