Dynamical systems-based neural networks
SIAM JOURNAL ON SCIENTIFIC COMPUTING(2023)
摘要
Neural networks have gained much interest because of their effectiveness in many applications. However, their mathematical properties are generally not well understood. If there is some underlying geometric structure inherent to the data or to the function to approximate, it is often desirable to take this into account in the design of the neural network. In this work, we start with a nonautonomous ODE and build neural networks using a suitable, structure-preserving, numerical time discretization. The structure of the neural network is then inferred from the properties of the ODE vector field. Besides injecting more structure into the network architectures, this modeling procedure allows a better theoretical understanding of their behavior. We present two universal approximation results and demonstrate how to impose some particular properties on the neural networks. A particular focus is on 1-Lipschitz architectures including layers that are not 1-Lipschitz. These networks are expressive and robust against adversarial attacks, as shown for the CIFAR-10 and CIFAR-100 datasets.
更多查看译文
关键词
neural networks,dynamical systems,Lipschitz networks,structure-preserving deep learning,universal approximation theorem
AI 理解论文
溯源树
样例
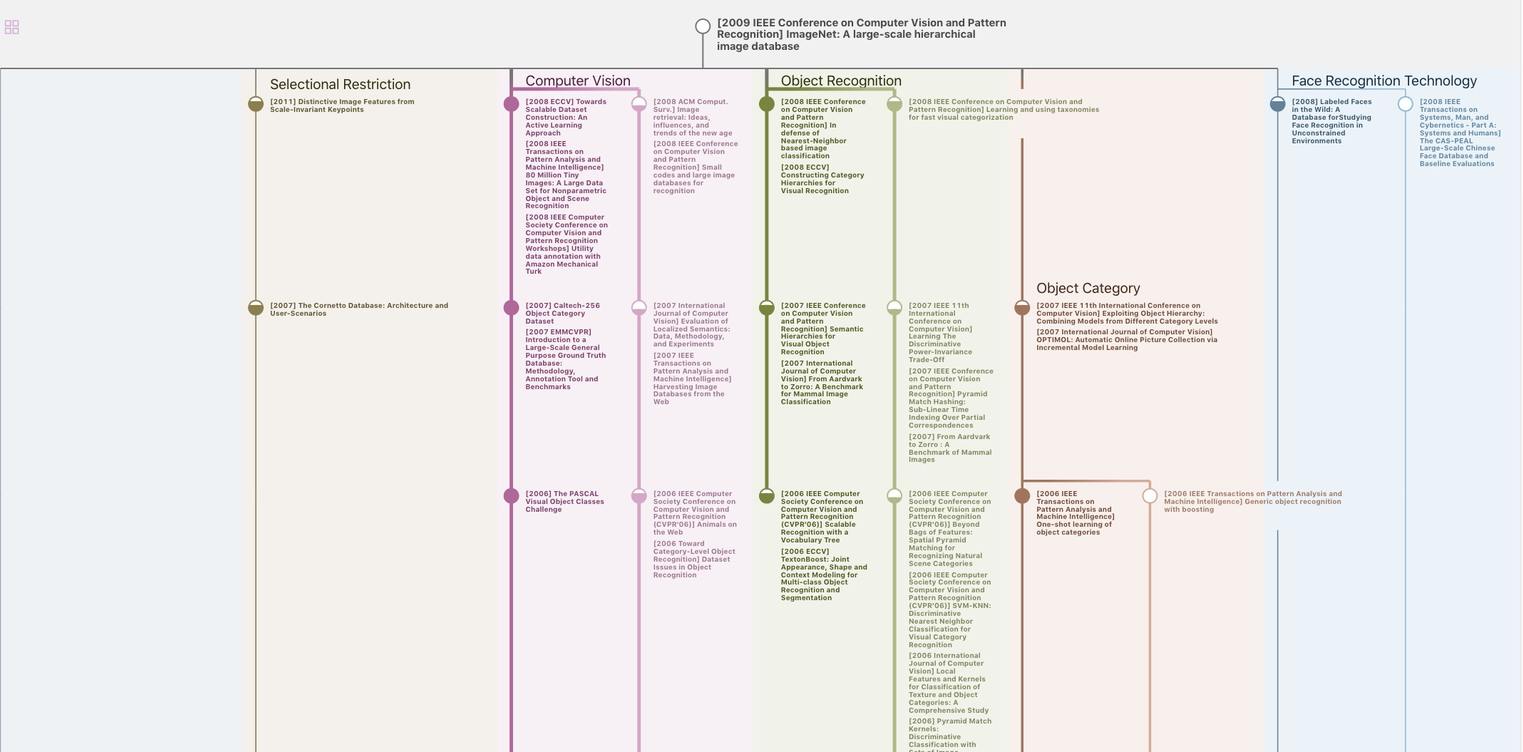
生成溯源树,研究论文发展脉络
Chat Paper
正在生成论文摘要