Hierarchical Adversarial Inverse Reinforcement Learning
IEEE TRANSACTIONS ON NEURAL NETWORKS AND LEARNING SYSTEMS(2023)
摘要
Imitation learning (IL) has been proposed to recover the expert policy from demonstrations. However, it would be difficult to learn a single monolithic policy for highly complex long-horizon tasks of which the expert policy usually contains subtask hierarchies. Therefore, hierarchical IL (HIL) has been developed to learn a hierarchical policy from expert demonstrations through explicitly modeling the activity structure in a task with the option framework. Existing HIL methods either overlook the causal relationship between the subtask structure and the learned policy, or fail to learn the high-level and low-level policy in the hierarchical framework in conjuncture, which leads to suboptimality. In this work, we propose a novel HIL algorithm-hierarchical adversarial inverse reinforcement learning (H-AIRL), which extends a state-of-the-art (SOTA) IL algorithm-AIRL, with the one-step option framework. Specifically, we redefine the AIRL objectives on the extended state and action spaces, and further introduce a directed information term to the objective function to enhance the causality between the low-level policy and its corresponding subtask. Moreover, we propose an expectation-maximization (EM) adaption of our algorithm so that it can be applied to expert demonstrations without the subtask annotations which are more accessible in practice. Theoretical justifications of our algorithm design and evaluations on challenging robotic control tasks are provided to show the superiority of our algorithm compared with SOTA HIL baselines. The codes are available at https://github.com/LucasCJYSDL/HierAIRL.
更多查看译文
关键词
Inverse reinforcement learning (IRL),hierarchical imitation learning (HIL),robotic learning
AI 理解论文
溯源树
样例
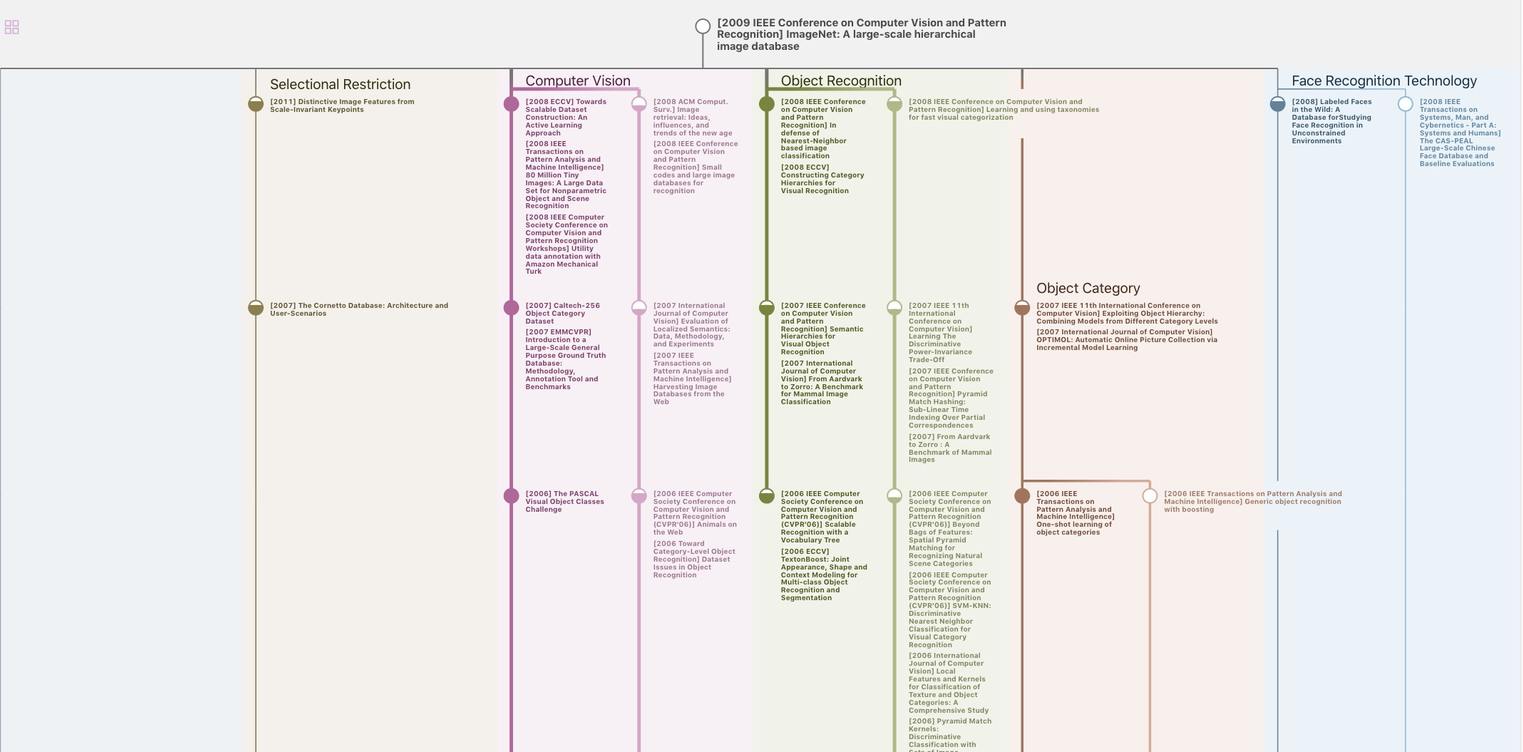
生成溯源树,研究论文发展脉络
Chat Paper
正在生成论文摘要