ContraGen: Effective Contrastive Learning For Causal Language Model
ICLR 2023(2022)
摘要
Despite exciting progress in large-scale language generation, the expressiveness of its representations is severely limited by the \textit{anisotropy} issue where the hidden representations are distributed into a narrow cone in the vector space. To address this issue, we present ContraGen, a novel contrastive learning framework to improve the representation with better uniformity and discrimination. We assess ContraGen on a wide range of downstream tasks in natural and programming languages. We show that ContraGen can effectively enhance both uniformity and discrimination of the representations and lead to the desired improvement on various language understanding tasks where discriminative representations are crucial for attaining good performance. Specifically, we attain $44\%$ relative improvement on the Semantic Textual Similarity tasks and $34\%$ on Code-to-Code Search tasks. Furthermore, by improving the expressiveness of the representations, ContraGen also boosts the source code generation capability with $9\%$ relative improvement on execution accuracy on the HumanEval benchmark.
更多查看译文
关键词
Natural Language Processing,Contrastive Learning,Causal Language Model,Natural Language Generation and Discrimination,Code Generation and Discrimination
AI 理解论文
溯源树
样例
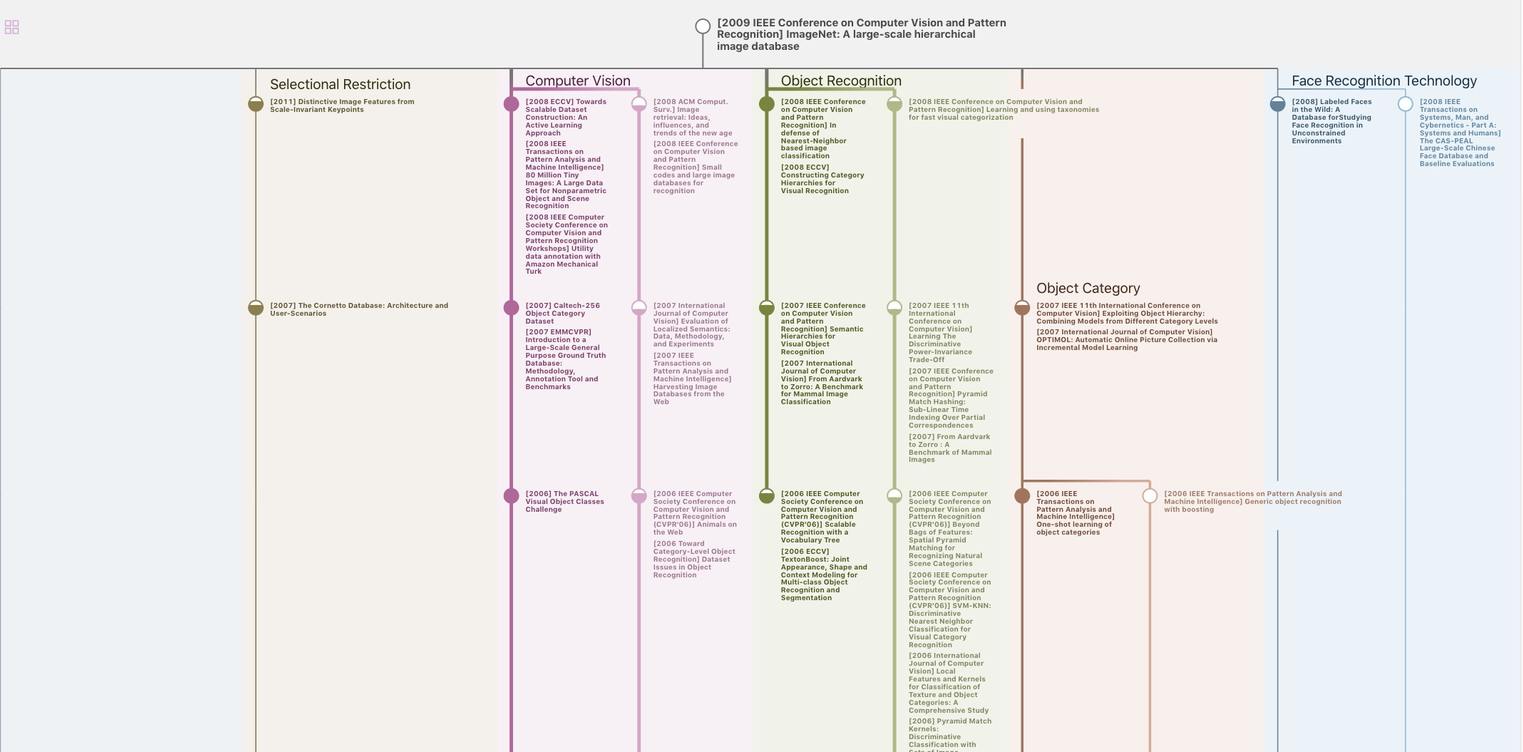
生成溯源树,研究论文发展脉络
Chat Paper
正在生成论文摘要