Multi-resolution hypergraph neural network for intelligent fault diagnosis
IEEE Transactions on Instrumentation and Measurement(2022)
摘要
Intelligent fault diagnosis has made significant progress, thanks to machine learning, particularly deep-learning algorithms. However, most machine-learning algorithms treat samples as independent and ignore the correlations between samples that contain valuable information for creating discriminative features. In recent years, graph neural networks have increased diagnostic performance by capturing the correlation between samples according to establishing the inherent structure of data, but they also suffer from two shortcomings. First, a simple graph only represents pairwise relationships of samples and cannot depict complex higher-order structures. Second, the generated graph structure is insufficient to characterize the data without an explicit structure. To address the above two issues, this article proposes a multiresolution hypergraph neural network, a novel algorithm that can discover higher-order complex relationships between samples, and mine the structure hidden in data by establishing and fusing hypergraph structures at multiple resolutions. Experiments are conducted on three datasets to demonstrate the effectiveness of the proposed algorithm.
更多查看译文
关键词
Convolution, Fault diagnosis, Correlation, Task analysis, Machinery, Machine learning algorithms, Laplace equations, Fault diagnosis, graph convolutional network (GCN), hypergraph, hypergraph neural network (HGNN), multiresolution
AI 理解论文
溯源树
样例
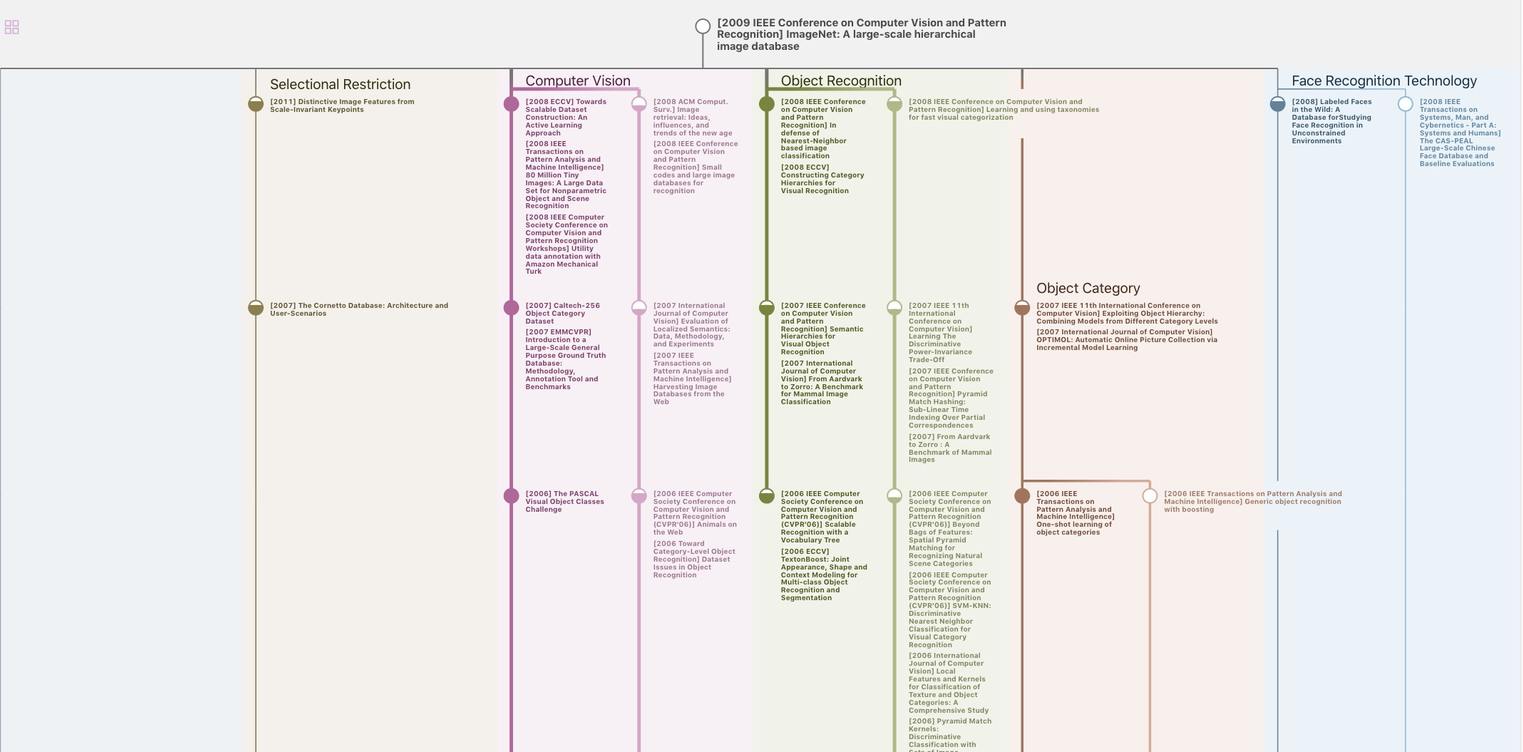
生成溯源树,研究论文发展脉络
Chat Paper
正在生成论文摘要