Ranking Models for the Temporal Dimension of Text
ACM Transactions on Information Systems(2023)
摘要
Temporal features of text have been shown to improve clustering and organization of documents, text classification, visualization, and ranking. Temporal ranking models consider the temporal expressions found in text (e.g., "in 2021" or "last year") as time units, rather than as keywords, to define a temporal relevance and improve ranking. This article introduces a new class of ranking models called Temporal Metric Space Models (TMSM), based on a new domain for representing temporal information found in documents and queries, where each temporal expression is represented as a time interval. Furthermore, we introduce a new frequency-based baseline called Temporal BM25 (TBM25). We evaluate the effectiveness of each proposed metric against a purely textual baseline, as well as several variations of the metrics themselves, where we change the aggregate function, the time granularity and the combination weight. Our extensive experiments on five test collections show statistically significant improvements of TMSM and TBM25 over state-of-the-art temporal ranking models. Combining the temporal similarity scores with the text similarity scores always improves the results, when the combination weight is between 2% and 6% for the temporal scores. This is true also for test collections where only 5% of queries contain explicit temporal expressions.
更多查看译文
关键词
Temporal information retrieval,Temporal Metric Space,texto-temporal relevance,temporal ranking,timexes,time similarity
AI 理解论文
溯源树
样例
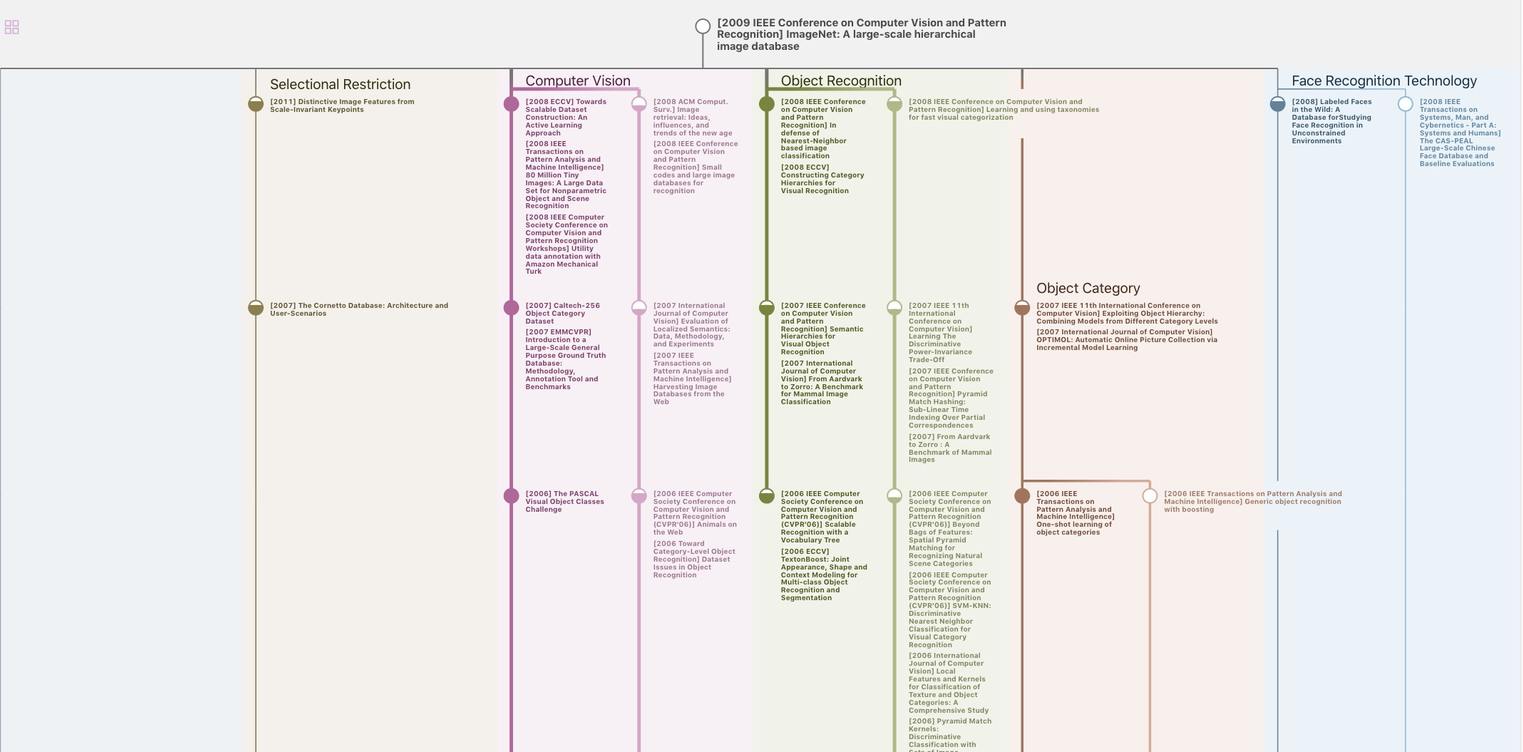
生成溯源树,研究论文发展脉络
Chat Paper
正在生成论文摘要