A Subspace Fusion of Hyper-parameter Optimization Method Based on Mean Regression
2022 IEEE International Conference on Smart Internet of Things (SmartIoT)(2022)
摘要
For object detection methods based on neural networks in computer vision, hyper-parameter is a crucial factor in the detection performance. Traditional hyperparameter optimization methods share the following shortcomings. (1) Search performance depends heavily on historical data and computational resources. (2) The open-loop structure of models may lead to unstable search results. We take missed detection targets as feedback to establish an iterative search model and propose a subspace-fusion optimization method based on mean regression. Firstly, the Successive Halving algorithm is deployed to determine the initial seeds, then detection subspaces and missed detection subspaces are generated according to the object detection results, and anchor-vector-based mean regressions are performed in the two subspaces respectively. Finally, we obtain the optimal parameters by a linear fusion of the two regression results. An early termination strategy is embedded into the search process to stop the invalid searches. Experiments show that within limited resource, this paper achieves significant improvement in search efficiency and detection performance compared with the classical methods.
更多查看译文
关键词
hyper-parameter optimization,mean regression,missed detection space
AI 理解论文
溯源树
样例
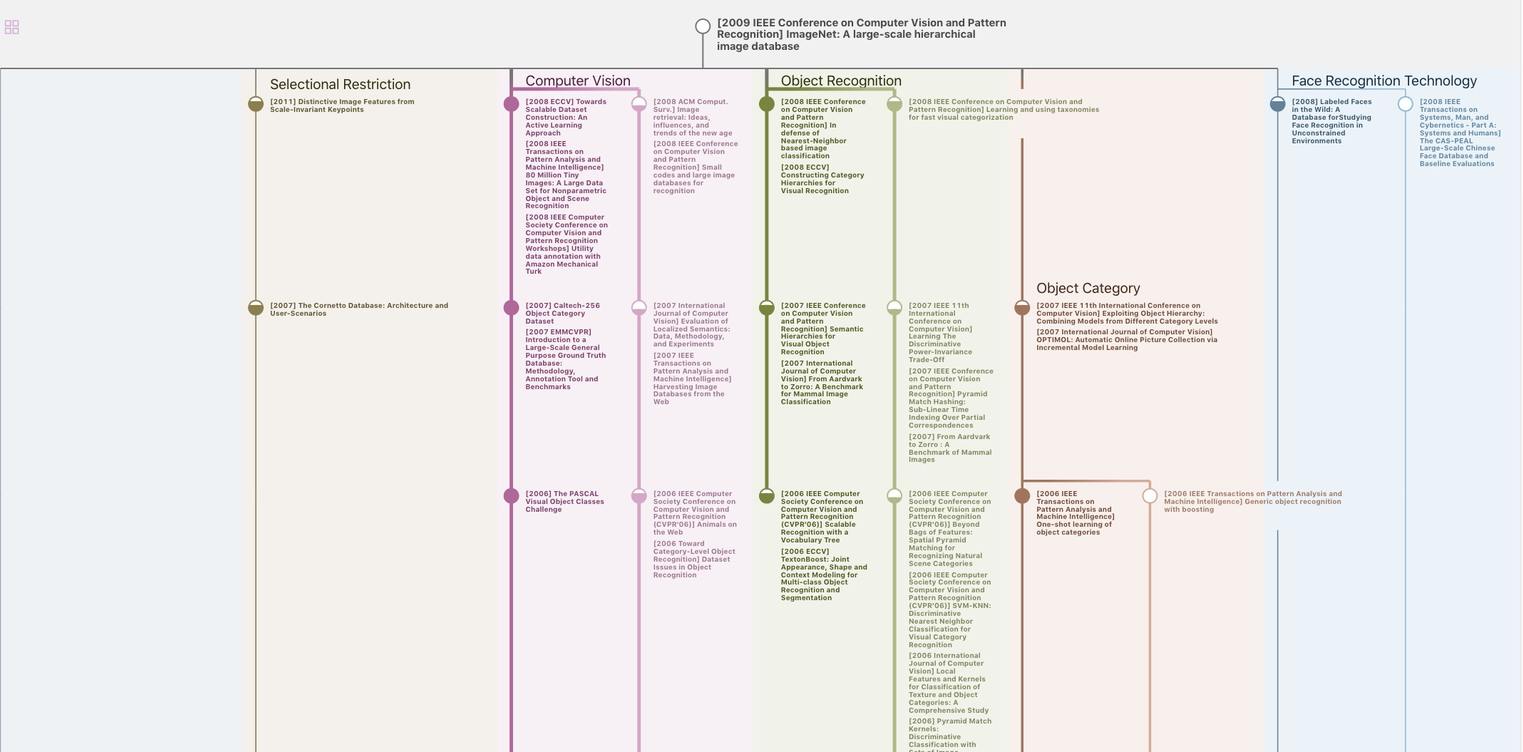
生成溯源树,研究论文发展脉络
Chat Paper
正在生成论文摘要