A Parallel Learning Approach for the Flexible Job Shop Scheduling Problem
IEEE Journal of Radio Frequency Identification(2022)
摘要
Reinforcement learning is emerging to achieve real-time response and near-optimization for solving the flexible job shop scheduling problem (FJSP), an important and NP-hard problem for intelligent manufacturing systems. Although some methods based on reinforcement learning have been proposed to solve the FJSP, there's still room for improvement. In this paper, we propose a new approach called reinforcement learning with the generative adversarial network (RLGAN) based on the parallel learning framework. A simulation-based artificial workshop system is established to generate a large number of sample plans as a training set for RLGAN to develop a near-optimal scheduling model. The case study shows that an implementation of our proposed method, QTRAN-GAN, can generate near-optimal plans and outperforms the corresponding pure reinforcement learning method, QTRAN.
更多查看译文
关键词
Flexible job shop scheduling,parallel learning,generative adversarial network,reinforcement learning
AI 理解论文
溯源树
样例
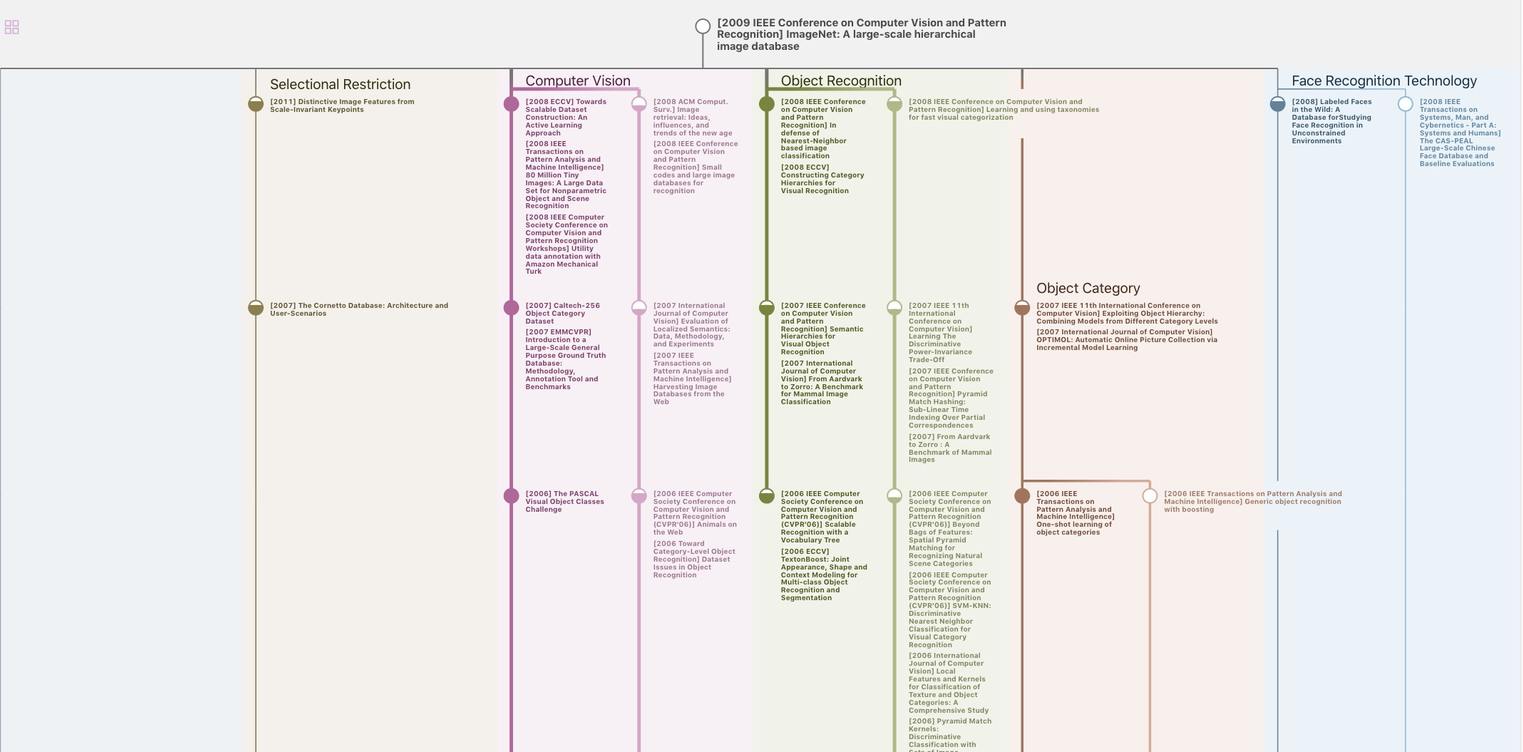
生成溯源树,研究论文发展脉络
Chat Paper
正在生成论文摘要