Learning Based Clinical Diagnosis Framework for Inconsistently and Partially Labeled Dataset
2022 Thirteenth International Conference on Ubiquitous and Future Networks (ICUFN)(2022)
摘要
This paper presents a novel deep learning framework for autonomous clinical diagnosis by dealing with the training with poorly labeled clinical dataset. Partially labeled data and inconsistent labels from multiple annotators make the model hard to learn accurate diagnosis in frequently and drastically updated clinical dataset. Motivated by such difficulties, the proposed framework introduces the weighted combination of inconsistent labels by considering multiple annotators' expertise and adapt meta-learning approach for the quick adaptation to the updated dataset. Experimental results on the posterior pelvic tilt detection in a squat motion show the proposed approach outperforms the conventional learning approaches in terms of the convergence speed and the converged mean squared error.
更多查看译文
关键词
Meta-learning,clinical diagnosis,inconsistently and partially labeled dataset,squat exercise,posterior pelvic tilt
AI 理解论文
溯源树
样例
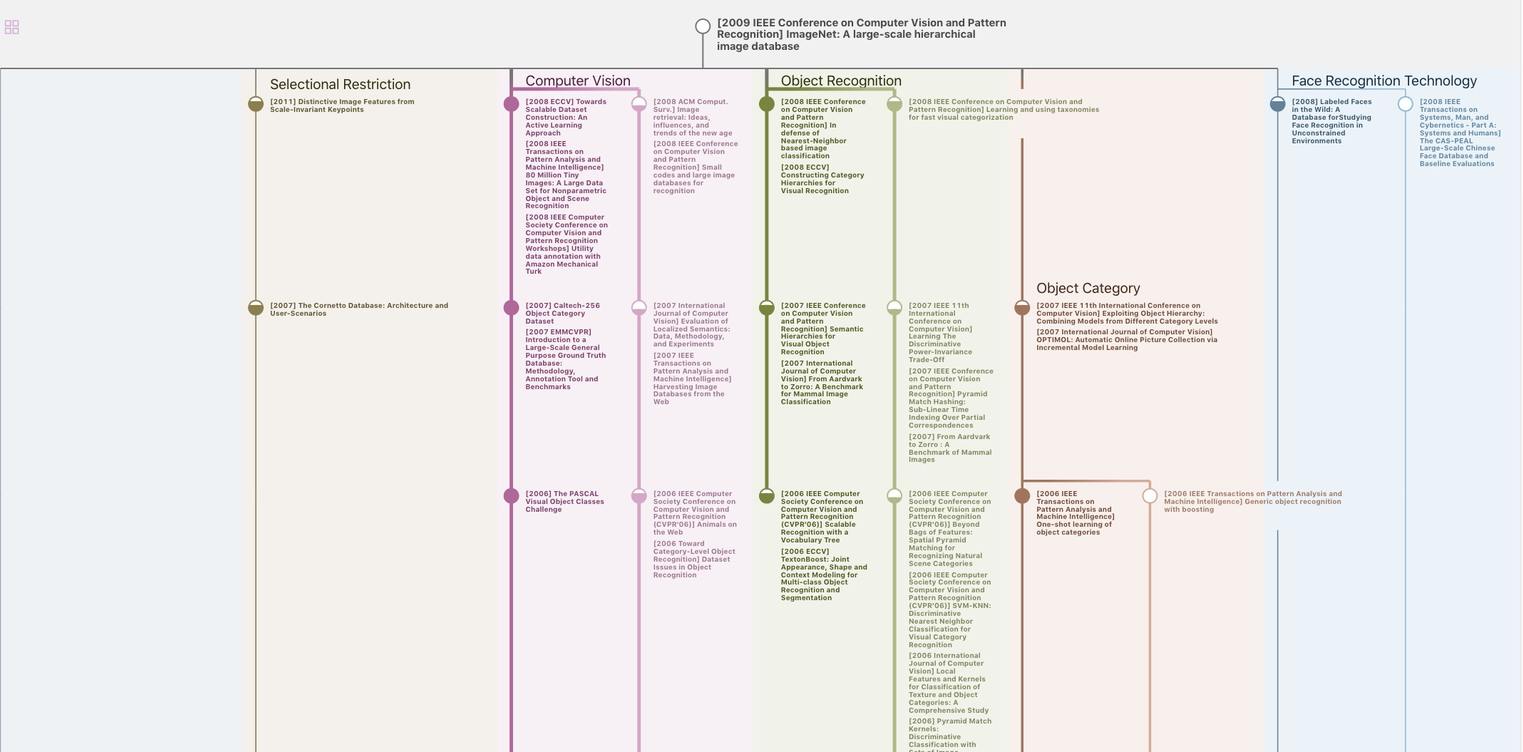
生成溯源树,研究论文发展脉络
Chat Paper
正在生成论文摘要