Reliable DNN Partitioning for UAV Swarm
2022 International Wireless Communications and Mobile Computing (IWCMC)(2022)
摘要
Recently deep neural networks (DNNs) are widely used in various fields. These intelligence applications, such as target recognition, are often computation-intensive and latency-sensitive. Since a single UAV's computing resource is limited, it is difficult to complete the DNN inference task independently. Partitioning the deep neural work into numerals subtasks and distributing them to multiple UAVs for collaborative computing seems a better way to finish the task. However, UAV usually works in a harsh environment, such as battlefield, disaster area, etc., and the link interruption or node failure in the inference process caused by uncertain factors may lead to failure of inference task. Hence, the reliability of DNN inference is of high importance. In this paper, we propose a deep Q learning-based DNN partitioning strategy for minimizing the energy consumption of DNN collaborative inference among multi-UAVs within latency and reliability requirements. To validate the effectiveness of the proposed strategy, a series of experiments are conducted on four kinds of typical DNNs (i.e., AlexNet, VGG19, GoogleNet, and ResNet). The simulation results prove the proposed strategy can effectively reduce the DNN inference cost under constraints.
更多查看译文
关键词
UAV,DNN partitioning,deep Q Networks (DQN),reliability,edge computing
AI 理解论文
溯源树
样例
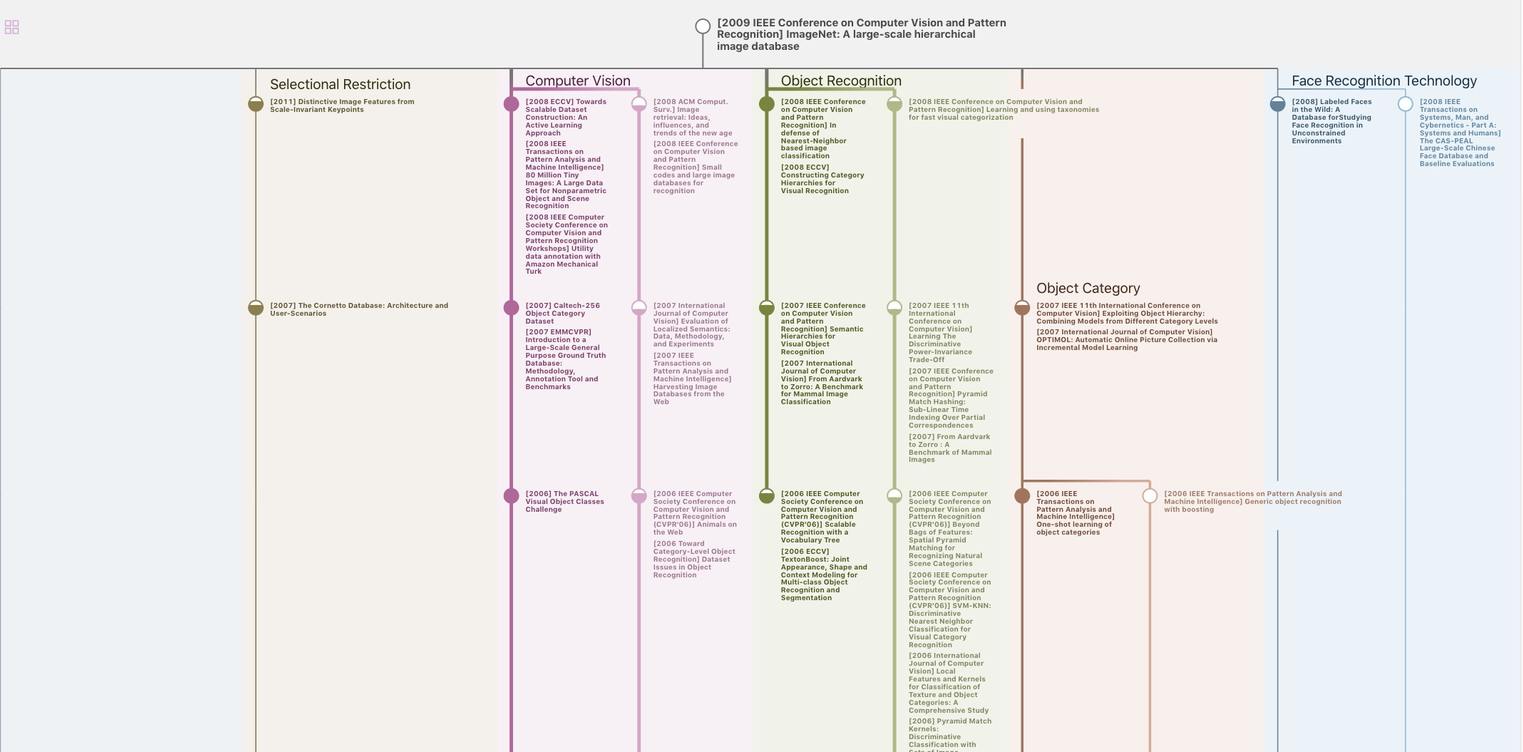
生成溯源树,研究论文发展脉络
Chat Paper
正在生成论文摘要