Explainable Detection of Fake News on Social Media Using Pyramidal Co-Attention Network
IEEE Transactions on Computational Social Systems(2022)
摘要
In today's world, fake news on social media is a universal trend and has severe consequences. There has been a wide variety of countermeasures developed to offset the effect and propagation of Fake News. The most common are linguistic-based techniques, which mostly use deep learning (DL) and natural language processing (NLP). Even government-sponsored organizations spread fake news as a cyberwar strategy. In literature, computational-based detection of fake news has been investigated to minimize it. The initial results of these studies are good but not significant. However, we argue that the explainability of such detection, particularly why a certain news item is detected as fake, is a vital missing element of the studies. In real-world settings, the explainability of the system's decisions is just as important as its accuracy. This article explores explainable fake news detection and proposes a sentence-comment-based co-attention sub-network model. The proposed model uses user comments and news contents to mutually apprehend top-k explainable check-worthy user comments and sentences for detecting fake news. The experimental result on real-world datasets shows that our proposed model outperforms state-of-the-art techniques by 5.56% in the F1 score. In addition, our model outperforms other baselines by 16.4% in normalized cumulative gain (NDCG) and 22.1% in Precision in identifying top-k comments from users, which indicates why a news article can be fake.
更多查看译文
关键词
Attention,deep learning (DL),fake news detection,long short-term memory (LSTM)
AI 理解论文
溯源树
样例
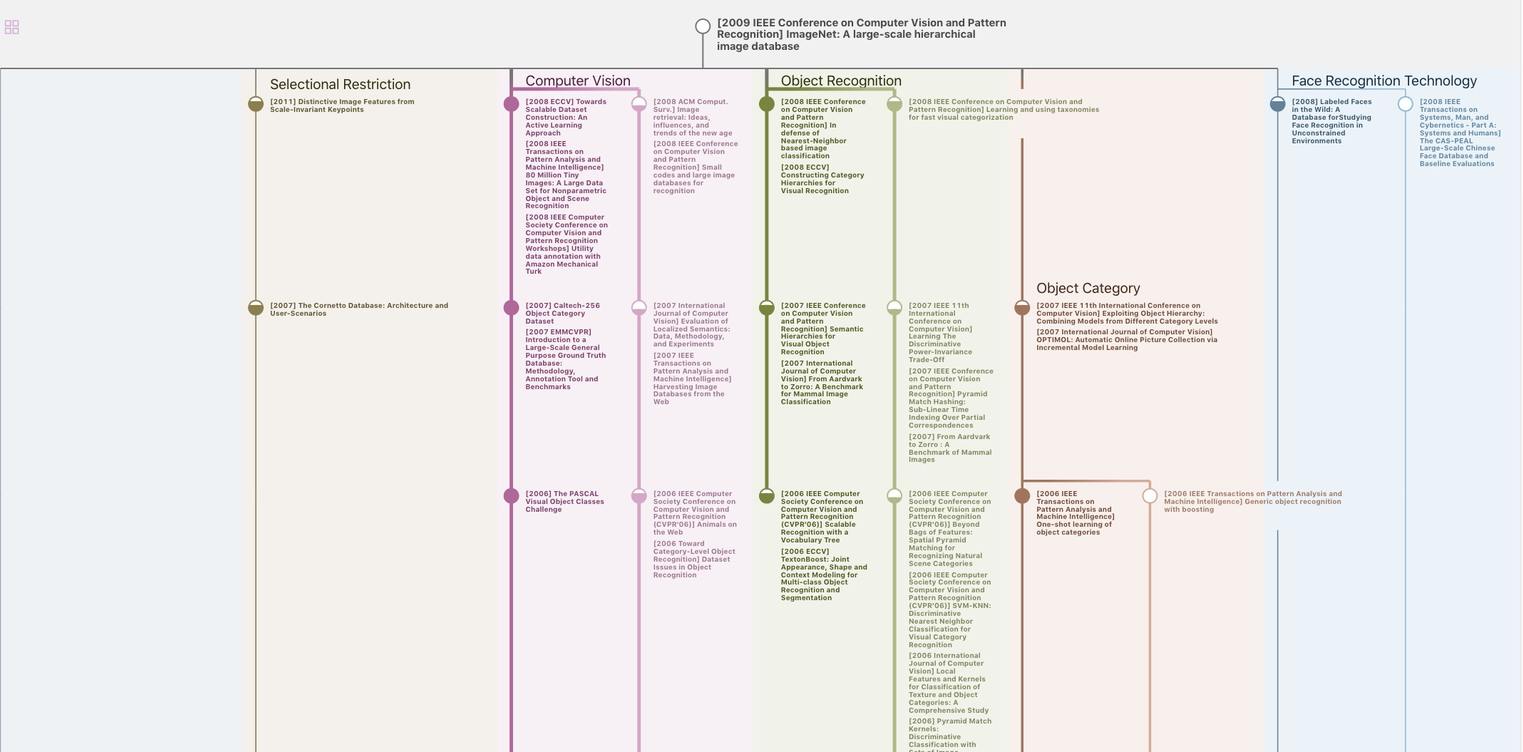
生成溯源树,研究论文发展脉络
Chat Paper
正在生成论文摘要