Combinatorial and algebraic perspectives on the marginal independence structure of Bayesian networks
arxiv(2022)
摘要
We consider the problem of estimating the marginal independence structure of
a Bayesian network from observational data, learning an undirected graph we
call the unconditional dependence graph. We show that unconditional dependence
graphs of Bayesian networks correspond to the graphs having equal independence
and intersection numbers. Using this observation, a Gröbner basis for a toric
ideal associated to unconditional dependence graphs of Bayesian networks is
given and then extended by additional binomial relations to connect the space
of all such graphs. An MCMC method, called GrUES (Gröbner-based Unconditional
Equivalence Search), is implemented based on the resulting moves and applied to
synthetic Gaussian data. GrUES recovers the true marginal independence
structure via a penalized maximum likelihood or MAP estimate at a higher rate
than simple independence tests while also yielding an estimate of the
posterior, for which the 20% HPD credible sets include the true structure at
a high rate for data-generating graphs with density at least 0.5.
更多查看译文
关键词
marginal independence
AI 理解论文
溯源树
样例
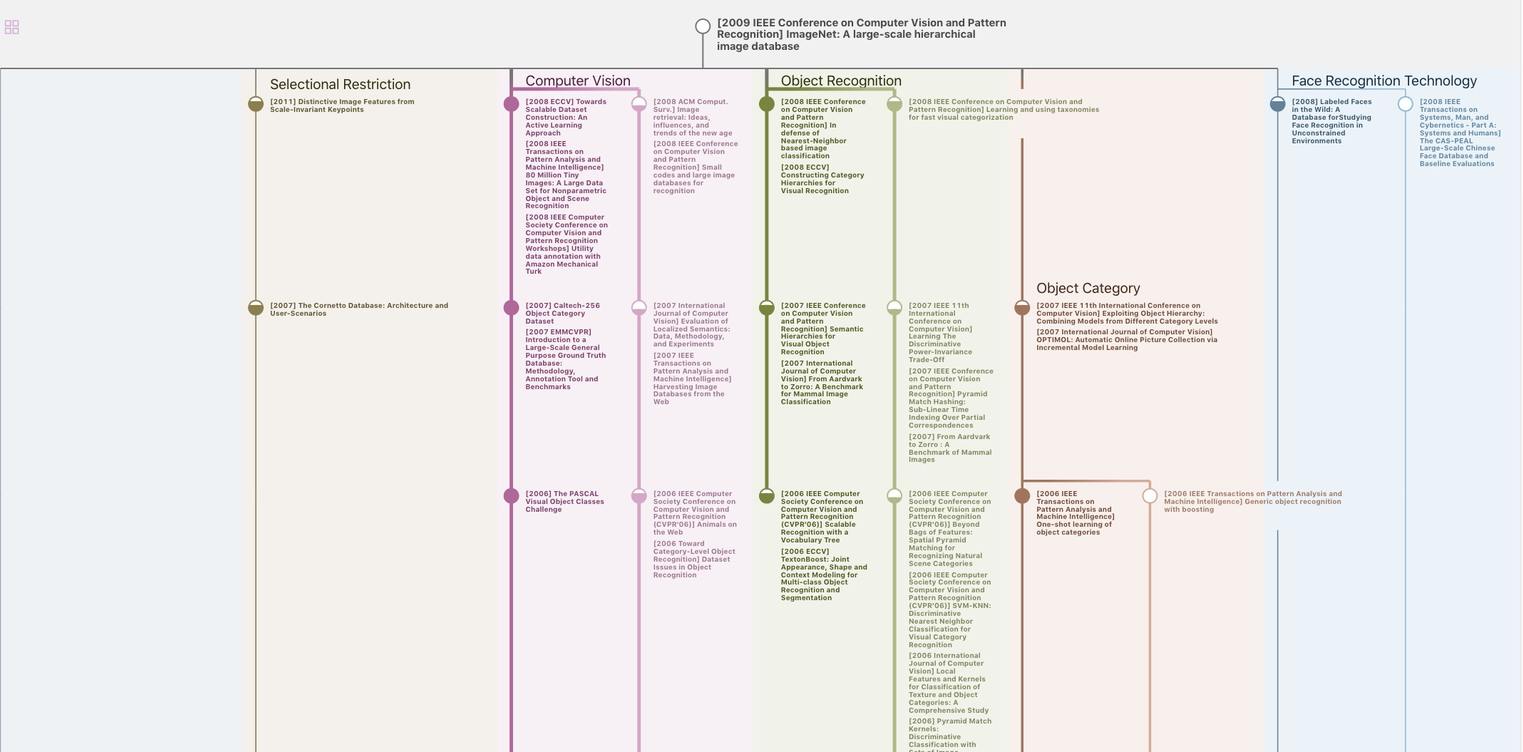
生成溯源树,研究论文发展脉络
Chat Paper
正在生成论文摘要