High Probability Convergence for Accelerated Stochastic Mirror Descent
arxiv(2022)
摘要
In this work, we describe a generic approach to show convergence with high probability for stochastic convex optimization. In previous works, either the convergence is only in expectation or the bound depends on the diameter of the domain. Instead, we show high probability convergence with bounds depending on the initial distance to the optimal solution as opposed to the domain diameter. The algorithms use step sizes analogous to the standard settings and are universal to Lipschitz functions, smooth functions, and their linear combinations.
更多查看译文
关键词
high probability convergence
AI 理解论文
溯源树
样例
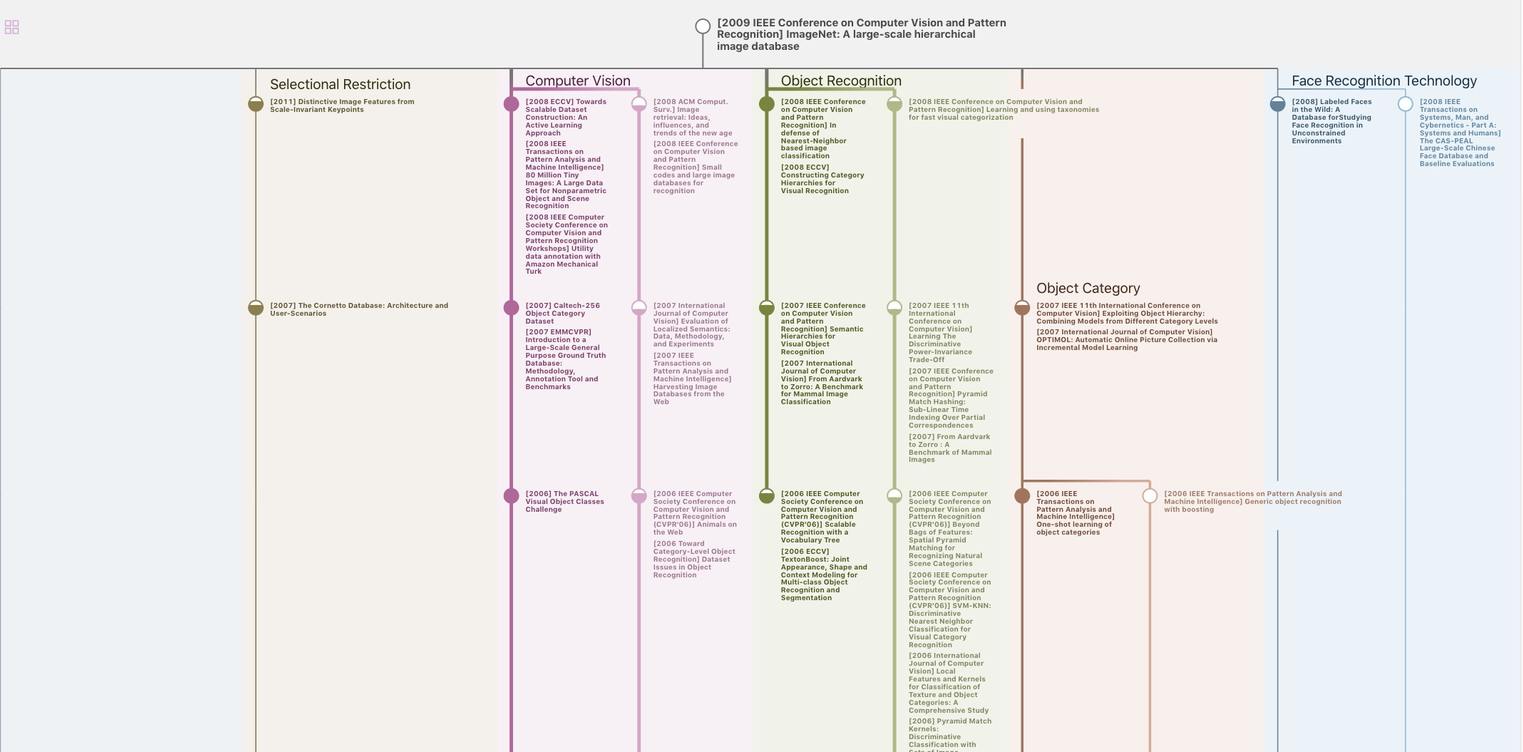
生成溯源树,研究论文发展脉络
Chat Paper
正在生成论文摘要