Learning Globally Smooth Functions on Manifolds
ICLR 2023(2023)
摘要
Smoothness and low dimensional structures play central roles in improving generalization and stability in learning and statistics. This work combines techniques from semi-infinite constrained learning and manifold regularization to learn representations that are globally smooth on a manifold. To do so, it shows that under typical conditions the problem of learning a Lipschitz continuous function on a manifold is equivalent to a dynamically weighted manifold regularization problem. This observation leads to a practical algorithm based on a weighted Laplacian penalty whose weights are adapted using stochastic gradient techniques. It is shown that under mild conditions, this method estimates the Lipschitz constant of the solution, learning a globally smooth solution as a byproduct. Experiments on real world data illustrate the advantages of the proposed method relative to existing alternatives.
更多查看译文
关键词
Lipschitz functions,Manifolds,Machine Learning
AI 理解论文
溯源树
样例
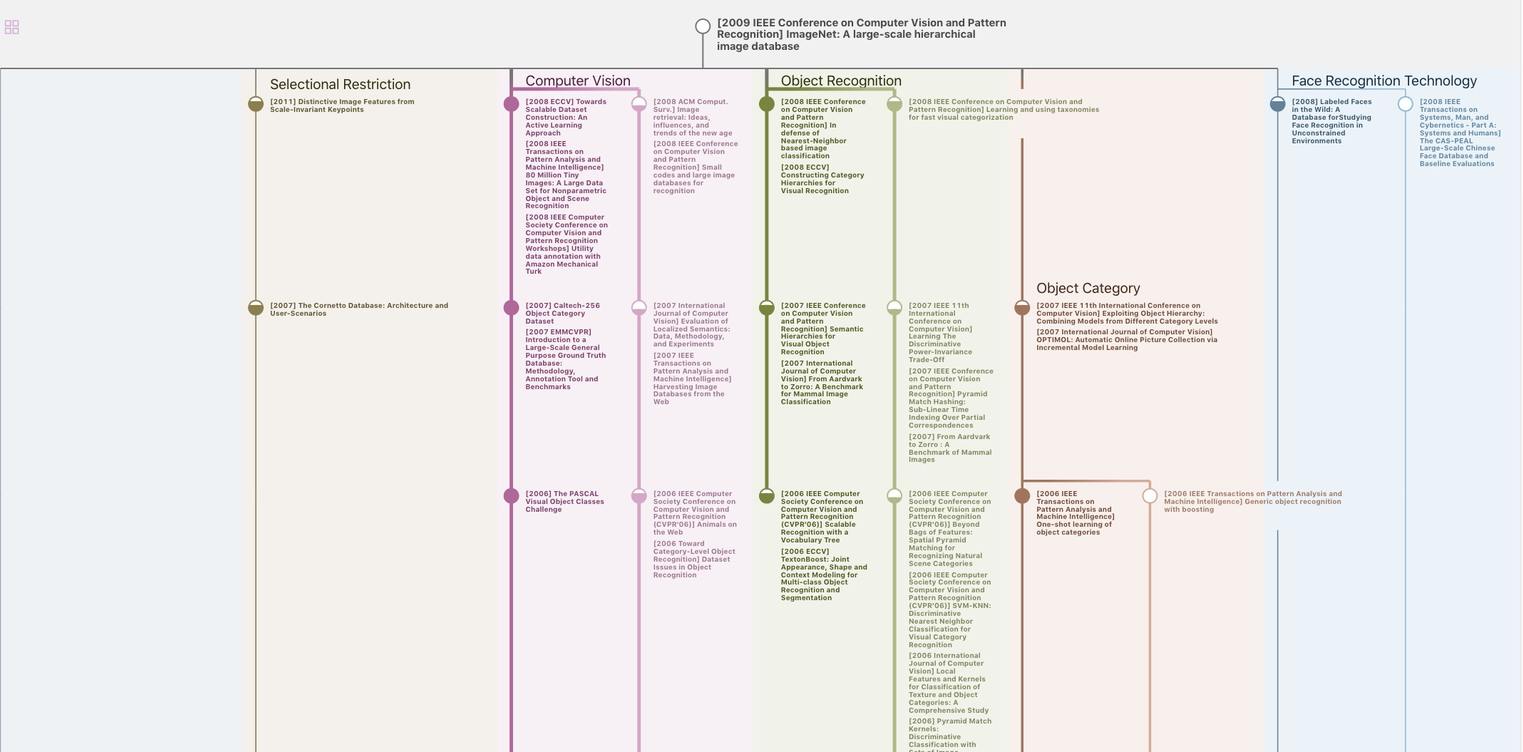
生成溯源树,研究论文发展脉络
Chat Paper
正在生成论文摘要