Spike-based local synaptic plasticity: a survey of computational models and neuromorphic circuits
NEUROMORPHIC COMPUTING AND ENGINEERING(2023)
摘要
Understanding how biological neural networks carry out learning using spike-based local plasticity mechanisms can lead to the development of real-time, energy-efficient, and adaptive neuromorphic processing systems. A large number of spike-based learning models have recently been proposed following different approaches. However, it is difficult to assess if these models can be easily implemented in neuromorphic hardware, and to compare their features and ease of implementation. To this end, in this survey, we provide an overview of representative brain-inspired synaptic plasticity models and mixed-signal complementary metal-oxide-semiconductor neuromorphic circuits within a unified framework. We review historical, experimental, and theoretical approaches to modeling synaptic plasticity, and we identify computational primitives that can support low-latency and low-power hardware implementations of spike-based learning rules. We provide a common definition of a locality principle based on pre- and postsynaptic neural signals, which we propose as an important requirement for physical implementations of synaptic plasticity circuits. Based on this principle, we compare the properties of these models within the same framework, and describe a set of mixed-signal electronic circuits that can be used to implement their computing principles, and to build efficient on-chip and online learning in neuromorphic processing systems.
更多查看译文
关键词
brain-inspired computing,neuromorphic CMOS circuits,spiking neural networks,local synaptic plasticity,online learning
AI 理解论文
溯源树
样例
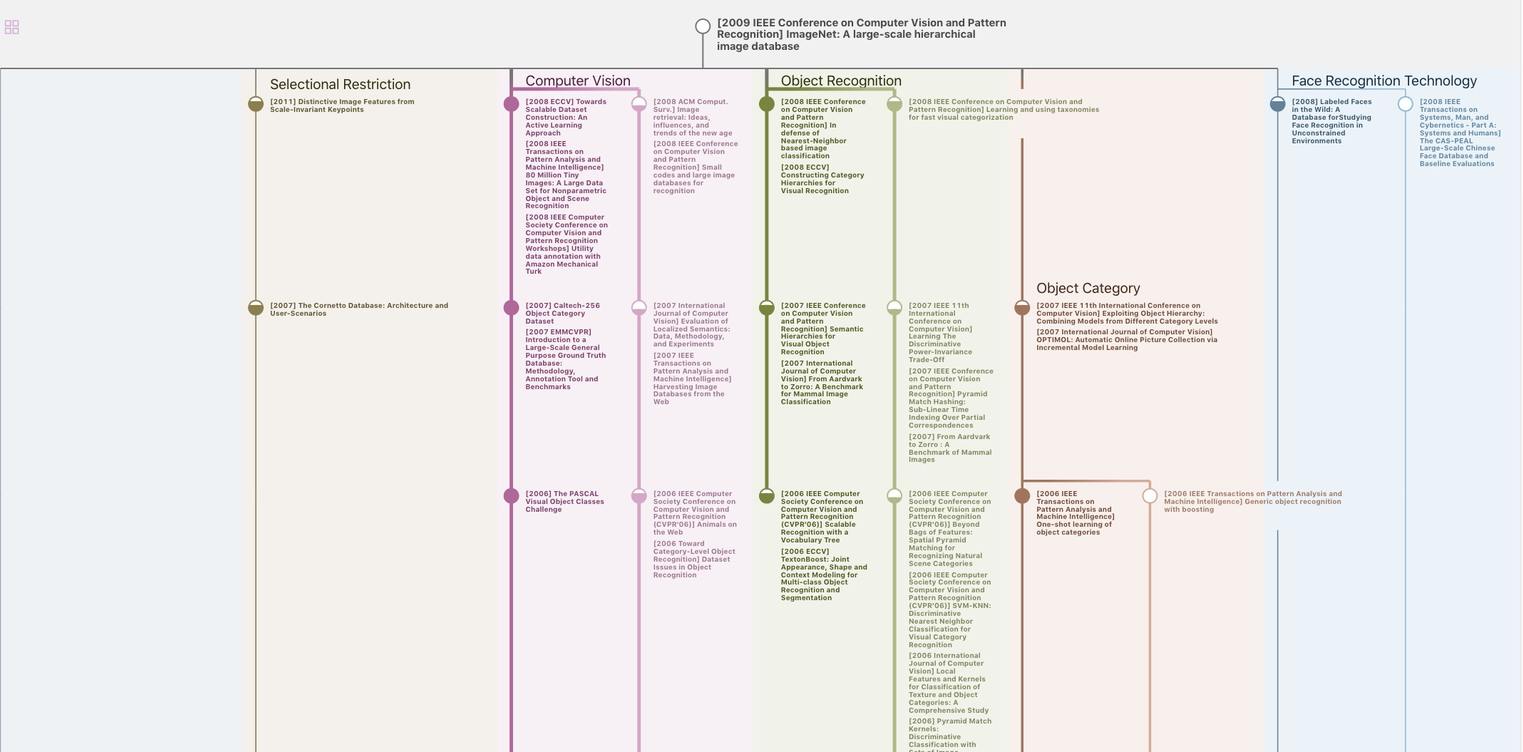
生成溯源树,研究论文发展脉络
Chat Paper
正在生成论文摘要