Safe Exploration Method for Reinforcement Learning under Existence of Disturbance
arxiv(2023)
摘要
Recent rapid developments in reinforcement learning algorithms have been giving us novel possibilities in many fields. However, due to their exploring property, we have to take the risk into consideration when we apply those algorithms to safety-critical problems especially in real environments. In this study, we deal with a safe exploration problem in reinforcement learning under the existence of disturbance. We define the safety during learning as satisfaction of the constraint conditions explicitly defined in terms of the state and propose a safe exploration method that uses partial prior knowledge of a controlled object and disturbance. The proposed method assures the satisfaction of the explicit state constraints with a pre-specified probability even if the controlled object is exposed to a stochastic disturbance following a normal distribution. As theoretical results, we introduce sufficient conditions to construct conservative inputs not containing an exploring aspect used in the proposed method and prove that the safety in the above explained sense is guaranteed with the proposed method. Furthermore, we illustrate the validity and effectiveness of the proposed method through numerical simulations of an inverted pendulum and a four-bar parallel link robot manipulator.
更多查看译文
关键词
Reinforcement learning, Safe exploration, Chance constraint
AI 理解论文
溯源树
样例
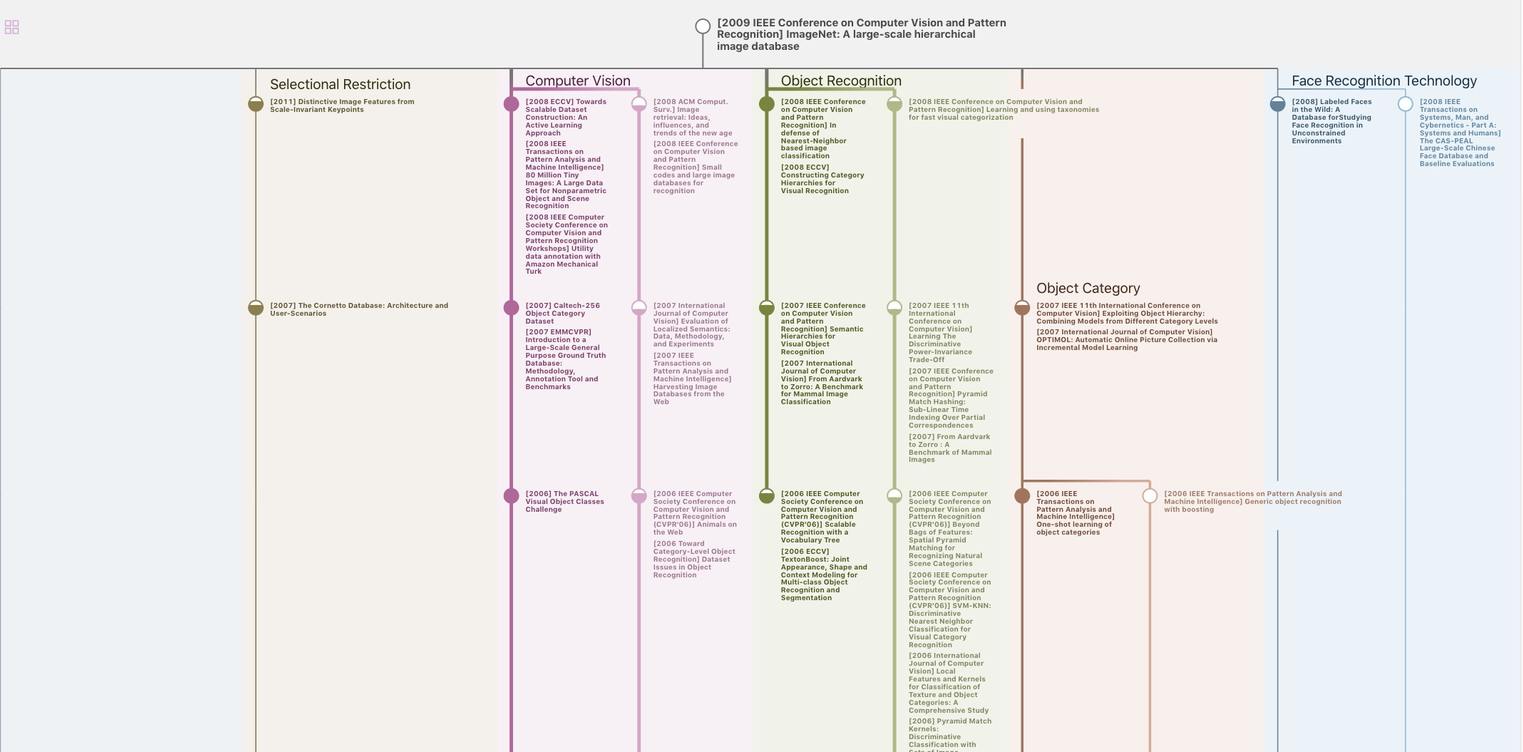
生成溯源树,研究论文发展脉络
Chat Paper
正在生成论文摘要