Identifying enhancing tumour without contrast-enhanced imaging
NEURO-ONCOLOGY(2022)
摘要
AIMS Brain tumours are heterogenous entities comprising multiple broad tissue sub-types when imaged with MRI. Delineating the enhancing tumour component is vital for neuro-oncological therapeutic planning, to-date only demonstrable with contrast-enhanced imaging. But not all patients can undergo this necessary contrast-enhanced acquisition, whether due to allergy, renal impairment, or scanning acquisition parameters. We therefore evaluated how well fully convolutional deep learning models identify a patient’s enhancing tumour when contrast-enhanced imaging was not available. METHOD We constructed a suite of deep-learning models to segment brain tumours when contrast-enhanced imaging was missing. Specifically, we developed all possible combinations of other structural sequences being provided, including T1-weighted, T2-weighted and FLAIR. Models were trained and tested with five-fold cross-validation on the 2021 BraTS-RSNA glioma population of 1251 patients, with additional out-of-sample validation with neuroradiologist hand-labelled lesions from our own centre. RESULTS Models missing post-contrast imaging still achieved a Dice coefficient for the whole tumour of 0.942. Model performances for identifying enhancing-tumour – despite no contrast-enhanced imaging being provided to the model – ranged from Dice coefficients of 0.759 (single sequence model) to 0.790 (three sequence T1 + T2 + FLAIR model). Moreover, models lacking contrast-enhanced imaging still robustly quantified the volume of enhancing tumour (R2 range 0.953-0.976). CONCLUSION Models missing contrast-enhanced imaging still identify both whole lesions and enhancing tumour components, and accurately quantifying the enhancing volumetric burden. These models provide opportunity for lesion detection in patients or clinical situations in which contrast-enhanced imaging cannot be acquired, and challenge the current nosology of defining ‘enhancing tumour’.
更多查看译文
关键词
tumour,imaging,contrast-enhanced
AI 理解论文
溯源树
样例
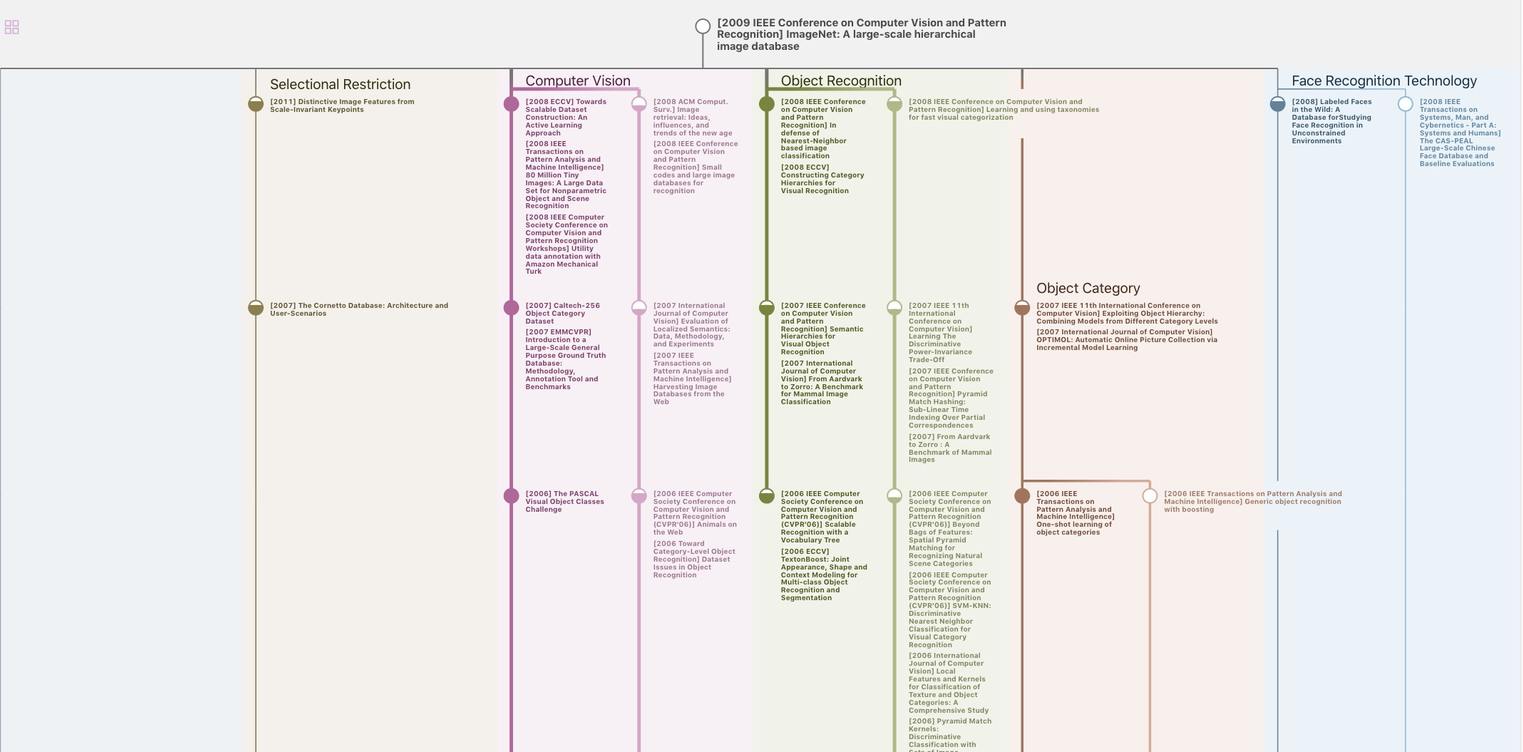
生成溯源树,研究论文发展脉络
Chat Paper
正在生成论文摘要