A novel multiple temporal-spatial convolution network for anode current signals classification
International Journal of Machine Learning and Cybernetics(2022)
摘要
Anode current signals (ACS) play an important role in aluminum reduction production. Owing to the complexity dynamic and temporal-spatial dependency characteristics, classification of ACS is a challenging problem and the existing classification methods are failed to capture these characteristics. To address this issue, a multiple temporal-spatial convolution network (MTSCN) combining graph convolutional network (GCN) and one-dimension convolutional neural network (1-D-CNN) is proposed in this paper. Firstly, a adjacency matrix is first introduced to characterize spatial structure of ACS. Secondly, based on the spatial structure, a novel machine learning framework which combines GCN and 1-D-CNN is proposed. Specifically, multi-layer of 1-D-CNN and multi-layer of GCN are used to capture temporal and spatial dependencies of ACS, respectively. The obtained data-dirved model is able to identify abnormalities of ACS. Finally, results carried out in real-world ACS data set are given to verify the effectiveness of the proposed method.
更多查看译文
关键词
Graph convolutional network,Temporal-spatial characteristics,Anode current signals,Aluminum reduction cell,Classification
AI 理解论文
溯源树
样例
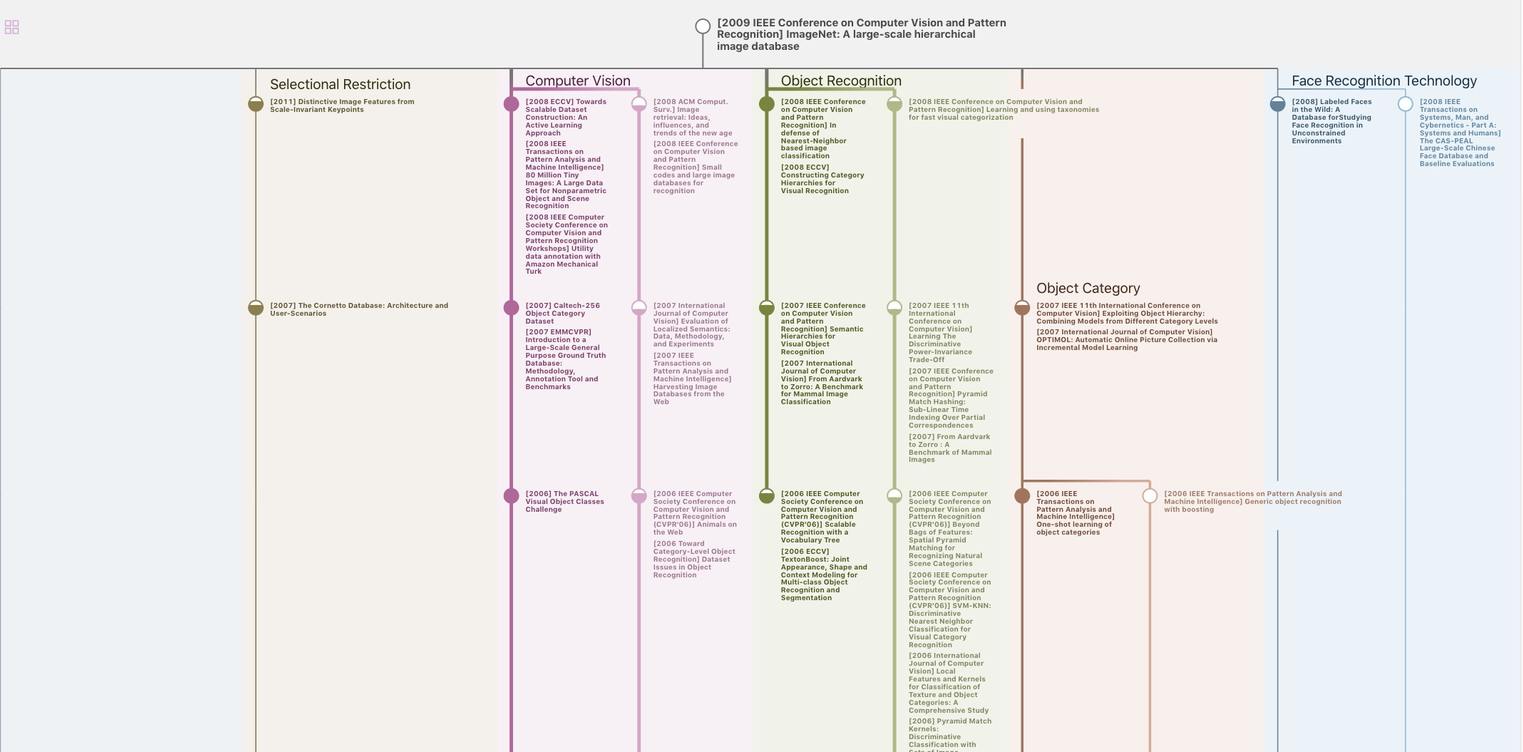
生成溯源树,研究论文发展脉络
Chat Paper
正在生成论文摘要