Downscaling UAV land surface temperature using a coupled wavelet-machine learning-optimization algorithm and its impact on evapotranspiration
Irrigation Science(2022)
摘要
Monitoring evapotranspiration (ET) is possible through land surface temperature (LST) measured by satellites and unmanned aerial vehicles (UAV). The assumption that the higher resolution of LST may improve the performance of remote sensing ET models was verified in a recently published article showing that higher resolution LST led to increased performance of the Two-source Energy Balance Model (TSEB)—one of the well-known ET models. However, because of technology limitations, the spatial resolutions of satellite and UAV thermal imagery are coarser than those in optical and near-infrared (NIR) bands. Therefore, developing thermal sharpening techniques and assessing their impacts on ET models performance are imperative. Although previous studies have developed and evaluated downscaling LST methods for satellite imagery, implementation of those methods on UAV imagery is limited. In this study, a coupled wavelet, machine learning, and optimization algorithm was implemented for downscaling UAV thermal imagery from 60 cm to the resolution of UAV optical imagery (15 cm) because 60 cm pixel resolution still incorporate mixed temperatures from the soil, vine canopy, active cover crop and shaded regions. A 2D discrete wavelet transform (2D DWT) was employed for the decomposition of inputs to 60 cm and inverse transformation of low thermal resolution to higher resolution. Four machine-learning-based algorithms (Decision Tree Regression (DTR), Ensemble Decision Tree (DTER), Support Vector Machine (SVM), and Gaussian process regression (GPR)) along with four linear regression-based models (linear, interactions linear, robust linear and stepwise linear) are used as the potential fitting models, and a grid search algorithm is used for auto-tuning parameters of the machine learning algorithms. Additionally, a novel sampling technique was designed to provide more representative samples for training steps in the regressing models. Four sets of high-resolution images were provided by the Utah State University AggieAir sUAS Program as part of the ARS-USDA GRAPEX Project collected since 2014 over multiple vineyards located in California. After applying the proposed downscaling algorithm, a separation method was used for estimation of canopy and soil temperatures from the original and sharpened thermal imagery. Ultimately, the TSEB model was executed for these pairs of temperature components, and its performance compared to eddy covariance measurements. Results demonstrated that the proposed sampling algorithm can significantly accelerate the computation time for the UAV temperature sharpening efforts. Among all the fitting models, GPR, SVM and DTER were the most accurate in terms of R-square. GPR, SVM and DTER’s R^2 were higher than 90
更多查看译文
AI 理解论文
溯源树
样例
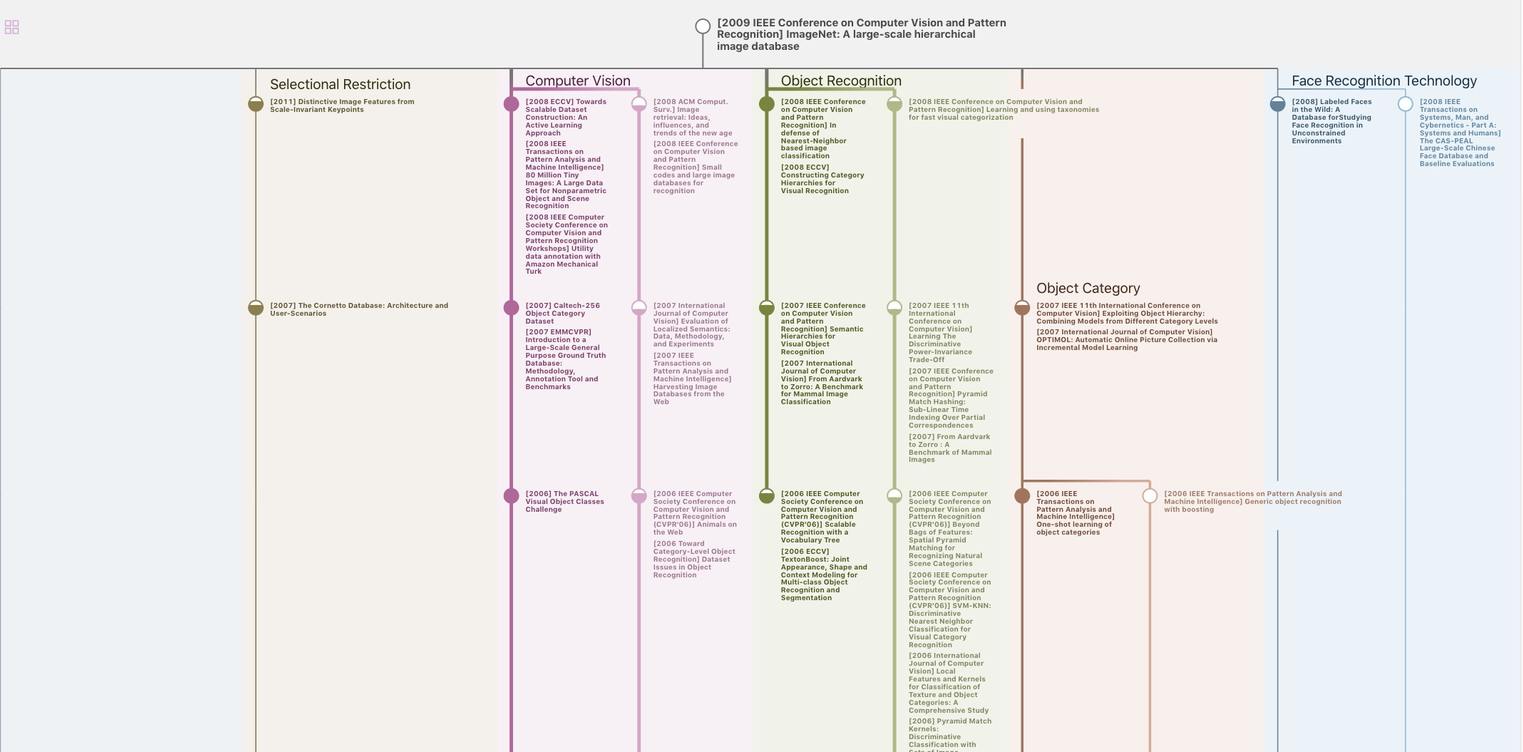
生成溯源树,研究论文发展脉络
Chat Paper
正在生成论文摘要