Efficient Neuromorphic Signal Processing with Resonator Neurons
Journal of Signal Processing Systems(2022)
摘要
The biologically inspired spiking neurons used in neuromorphic computing are nonlinear filters with dynamic state variables, which is distinct from the stateless neuron models used in deep learning. The new version of Intel’s neuromorphic research processor, Loihi 2, supports an extended range of stateful spiking neuron models with programmable dynamics. Here, we showcase advanced neuron models that can be used to efficiently process streaming data in simulation experiments on emulated Loihi 2 hardware. In one example, Resonate-and-Fire (RF) neurons are used to compute the Short Time Fourier Transform (STFT) with similar computational complexity but 47x less output bandwidth than the conventional STFT. In another example, we describe an algorithm for optical flow estimation using spatiotemporal RF neurons that requires over 90x fewer operations than a conventional DNN-based solution. We also demonstrate backpropagation methods to train non-linear spiking RF neurons for audio classification tasks, suitable for efficient execution on Loihi 2. We conclude with another application of nonlinear filtering showing a cascade of Hopf resonators exhibiting computational properties seen in the cochlea, such as self-normalization. Taken together, this work presents new techniques for an efficient spike-based spectrogram encoder that can be used for signal processing applications.
更多查看译文
关键词
Neuromorphic computing, Resonator neurons, Spiking neural networks, Optic flow, Speech recognition
AI 理解论文
溯源树
样例
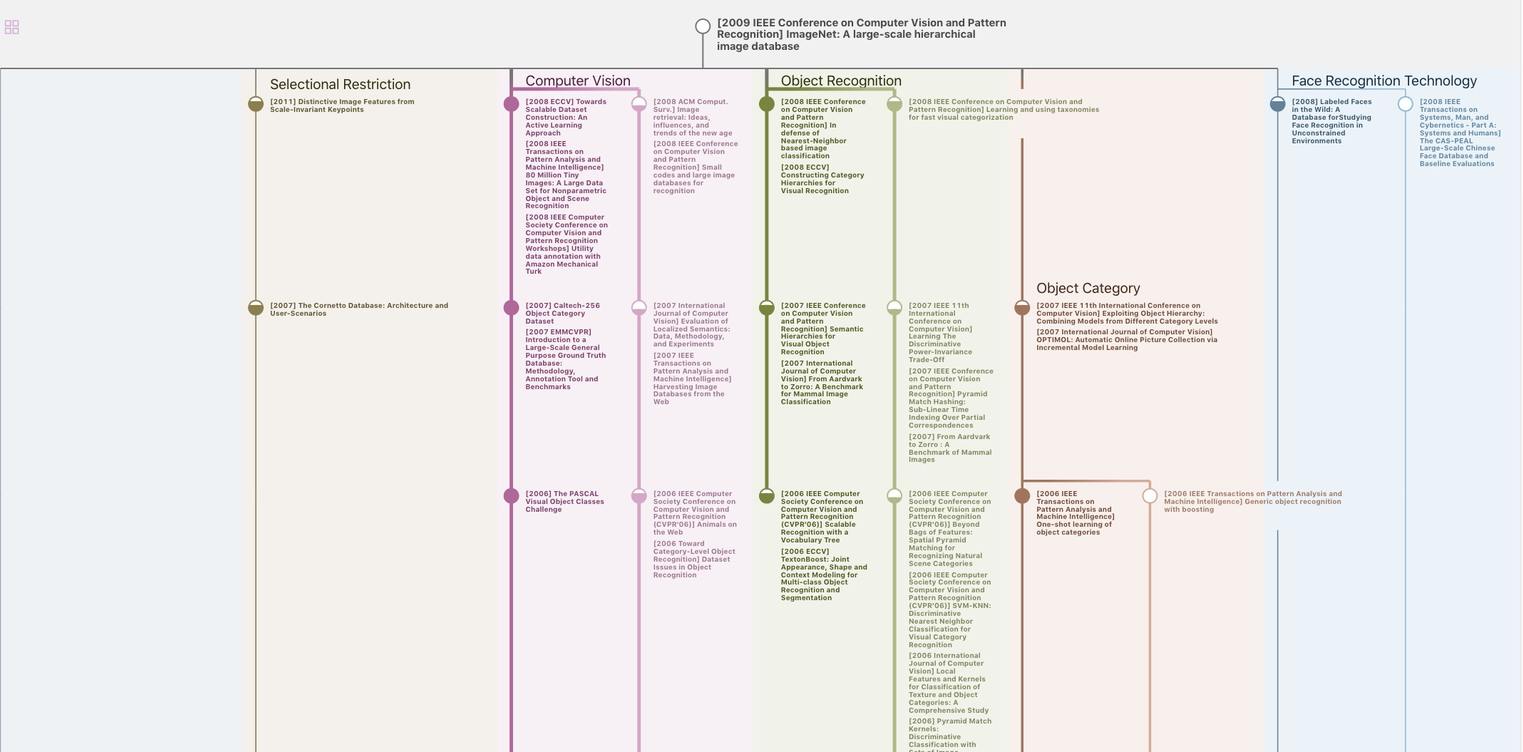
生成溯源树,研究论文发展脉络
Chat Paper
正在生成论文摘要