A Deep Reinforcement Learning Framework for High-Dimensional Circuit Linearization
IEEE Transactions on Circuits and Systems II: Express Briefs(2022)
摘要
Despite the successes of Reinforcement Learning (RL) in recent years, tasks that require exploring over long trajectories with limited feedback and searching in high-dimensional space remain challenging. This brief proposes a deep RL framework for high-dimensional circuit linearization with an efficient exploration strategy leveraging a scaled dot-product attention scheme and search on the replay technique. As a proof of concept, a 5-bit digital-to-time converter (DTC) is built as the environment, and an RL agent learns to tune the calibration words of the delay stages to minimize the integral nonlinearity (INL) with only scalar feedback. The policy network which selects calibration words is trained by the Soft Actor-Critic (SAC) algorithm. Our results show that the proposed RL framework can reduce the INL to less than 0.5 LSB within 60, 000 trials, which is much smaller than the size of searching space.
更多查看译文
关键词
Deep reinforcement learning,circuits calibration,high-dimensional searching,attention scheme
AI 理解论文
溯源树
样例
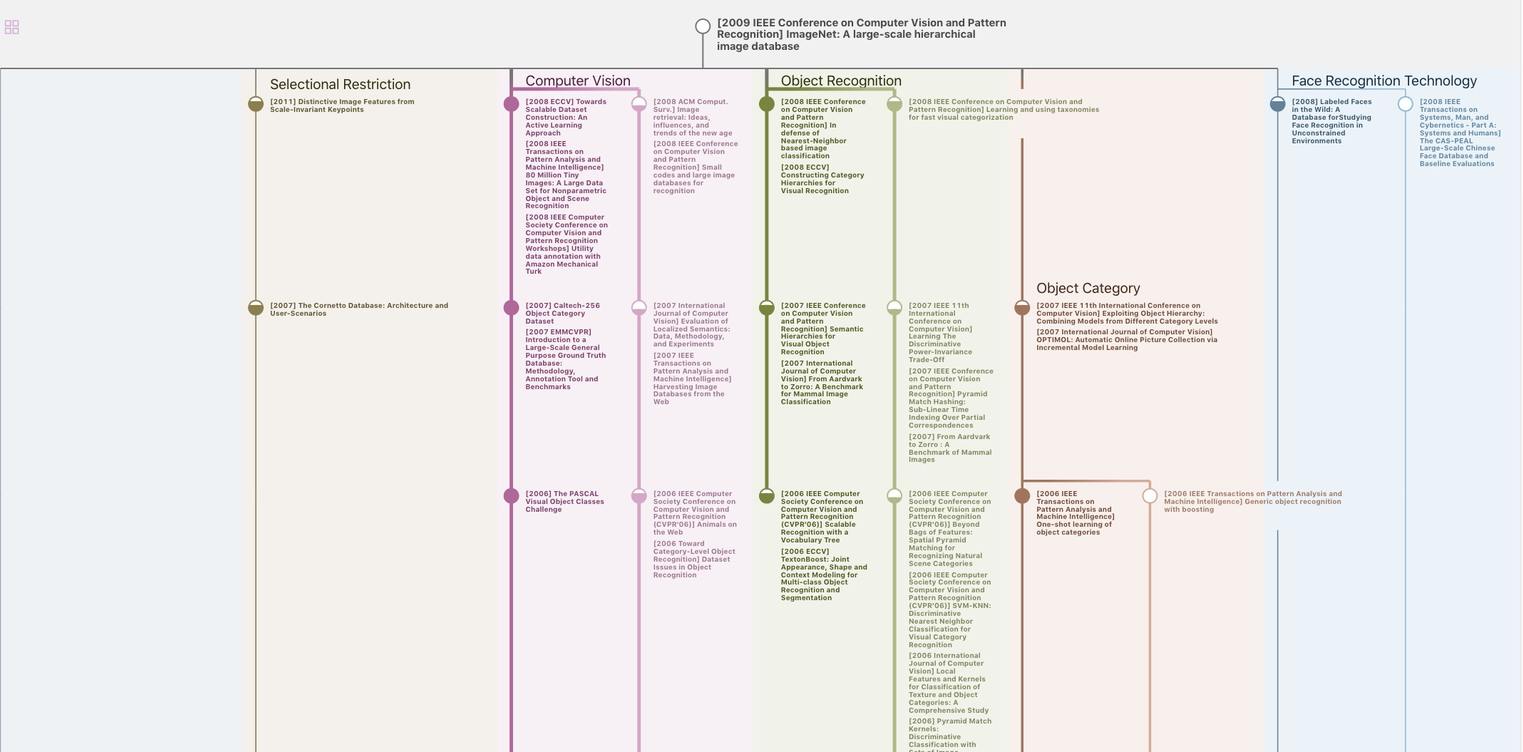
生成溯源树,研究论文发展脉络
Chat Paper
正在生成论文摘要