A 384G Output NonZeros/J Graph Convolutional Neural Network Accelerator
IEEE Transactions on Circuits and Systems II: Express Briefs(2022)
摘要
This brief presents the first IC implementation of graph convolutional neural network (GCN) accelerator chip. A sparsity aware dataflow optimized for sub-block-wise processing of three different matrices in GCN is proposed to improve the utilization ratio of computing resources while reducing the amount of redundant access of off-chip memory. The implemented accelerator in 28-nm CMOS produces 384G NZ outputs/J for the extremely sparse matrix multiplications of the GCN. It shows 58k-to-143k, 38k-to-92k and 5k-to-13k Graph/J for the benchmark graph datasets of Cora, Citeseer and Pubmed, respectively. The energy efficiency in Graph/J of the proposed 16b ASIC implementation shows about 4-to-
$11\mathbf {\times }$
and 8-to-
$25\mathbf {\times }$
improvements compared to the previously reported 8b FPGA and 32b FPGA implementations, respectively.
更多查看译文
关键词
Graph convolutional neural network (GCN),hardware accelerator,machine learning accelerator,sparse matrix multiplication,application-specific integrated circuit (ASIC)
AI 理解论文
溯源树
样例
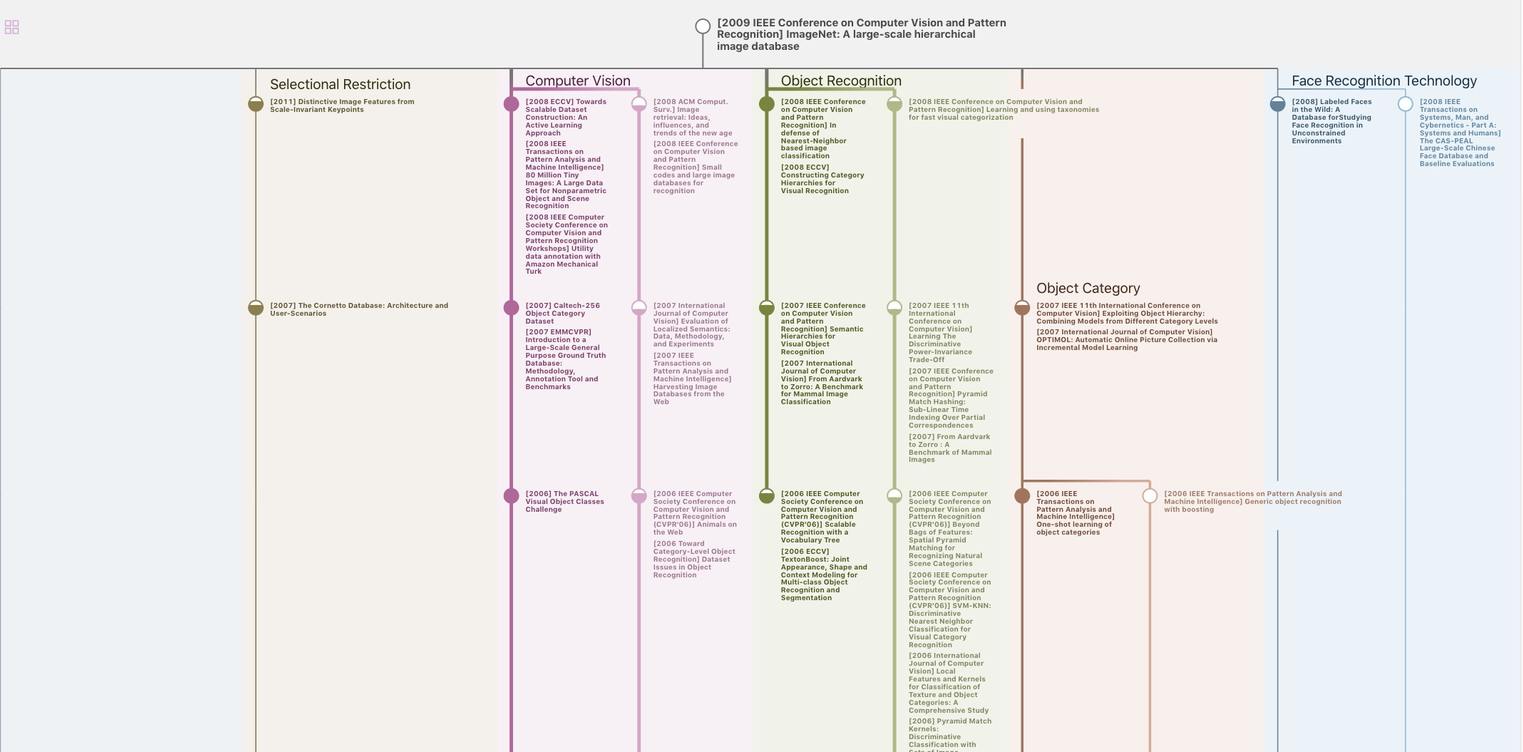
生成溯源树,研究论文发展脉络
Chat Paper
正在生成论文摘要