Graph learning in low dimensional space for graph convolutional networks
Multimedia Tools and Applications(2021)
摘要
Graph Convolutional Networks (GCNs) recently have been adopted in several feature representation studies for different classification tasks. While many of these methods are used to work with irregular structure data, they are rarely used to learn regular structure data. It is crucial to construct an excellent graph representation to traditional classification tasks for obtaining the sufficient data representation, including attribute representation and relative representation. In this context, we propose a novel method to construct a reasonable graph representation by capturing the relations in low dimensional space among the data. In order to get the well-represented, we introduce the low-rank constraint and L2-norm regularization to the graph learning framework simultaneously. Experiments demonstrate that the proposed method to learn graph representation is helpful for classification task, and leads to improved performance when compared to state-of-the-art graph learning methods on twelve data sets.
更多查看译文
关键词
GCNs, Graph learning, Dimension reduction
AI 理解论文
溯源树
样例
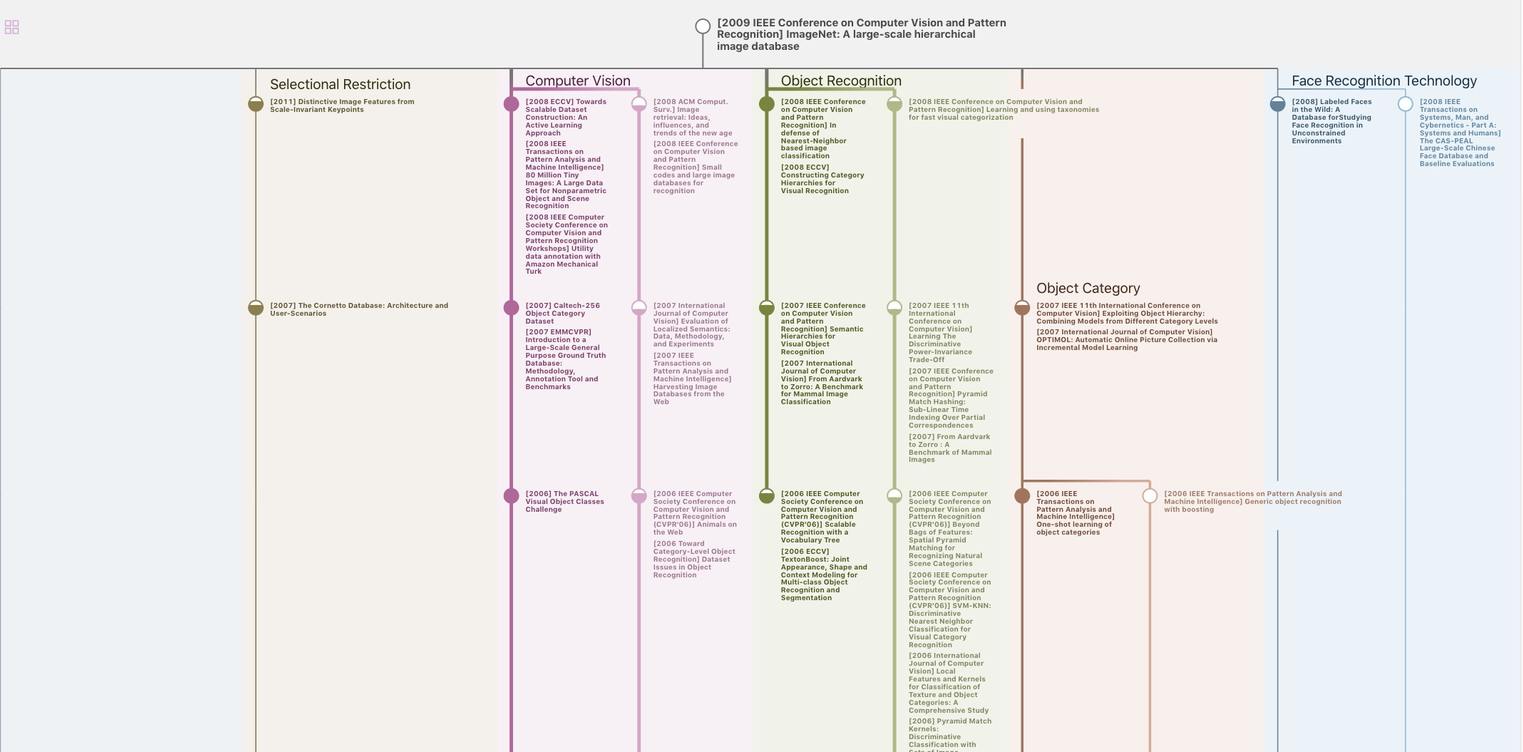
生成溯源树,研究论文发展脉络
Chat Paper
正在生成论文摘要