Application of Gradient Boosting in Evaluating Surgical Ablation for Atrial Fibrillation
SN Computer Science(2022)
摘要
Atrial fibrillation (AF) represents a condition of irregularities of heartbeats. Timely diagnosis and treatment of AF are crucial to avoid serious consequences such as stroke, heart failure and death. AF is detected from patient’s rhythm data as a binary longitudinal sequence. In this article, we introduce an approach for analyzing the binary longitudinal sequence of AF. Our approach is based on gradient boosting, a machine learning approach. We use multivariate tree as a base learner to model complex relationships between multiple covariates and response. In order to model covariate-time interactions, we use B-spline. Application of our approach to a randomized trial data provides the importance of surgical ablation in the treatment of AF among patients with persistent or long-standing persistent AF. Comparison of prediction performance of our approach with other methods using randomized trial data and simulated data shows that our approach has a better prediction performance. Variable selection using variable importance has identified duration of AF as an important covariate that has strong interaction with post-surgery time. This helps to identify patients’ variability and groups of patients who derived the most benefits from the treatment. Our method can be implemented using the R package boostmtree.
更多查看译文
关键词
Gradient boosting, Binary longitudinal response, Multivariate regression tree, B-spline, Atrial fibrillation
AI 理解论文
溯源树
样例
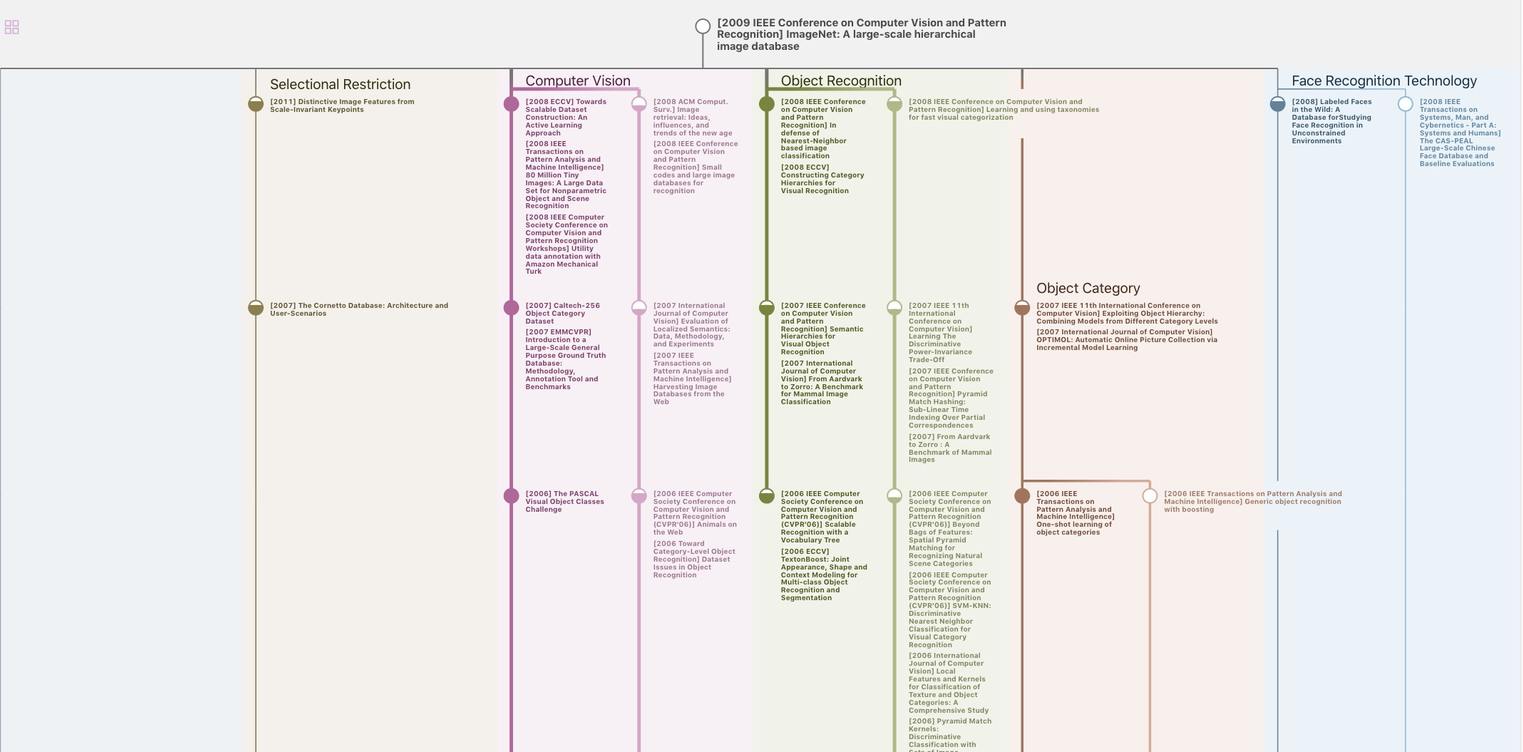
生成溯源树,研究论文发展脉络
Chat Paper
正在生成论文摘要