Boosting for Multivariate Longitudinal Responses
SN Computer Science(2022)
摘要
Boosting, a machine learning approach, has gained popularity over the years in its application to various types of data, including longitudinal data. However, its application to data involving multivariate responses is limited. In this article, we present a new approach where we apply gradient boosting, a generic form of boosting, to model multivariate longitudinal responses. Our approach can handle time-varying covariates as well as high dimensionality of covariates and responses when some of the covariates and responses are pure noise. A key feature of our approach is that it is designed to select covariates that affect responses differently at different time intervals; thereby, an overall effect of any covariate can be dissected and represented as a function of time. A novel feature of our approach is that, in addition to covariate selection, we also perform response selection for different time intervals. This helps to identify and order responses based on their importance for a given time interval. Simulation results show that the prediction performance of our approach does not deteriorate in high dimensionality and can approximate the true model. Application of our approach to a clinical laboratory data evaluates the behavior of bilirubin and creatinine for the heart failure patients before and after the heart transplant, and identifies important risk factors that affect their behavior. Our approach can be implemented using the R package BoostMLR
更多查看译文
关键词
Multivariate longitudinal responses, Gradient boosting,
B-spline, Variable importance, Response selection
AI 理解论文
溯源树
样例
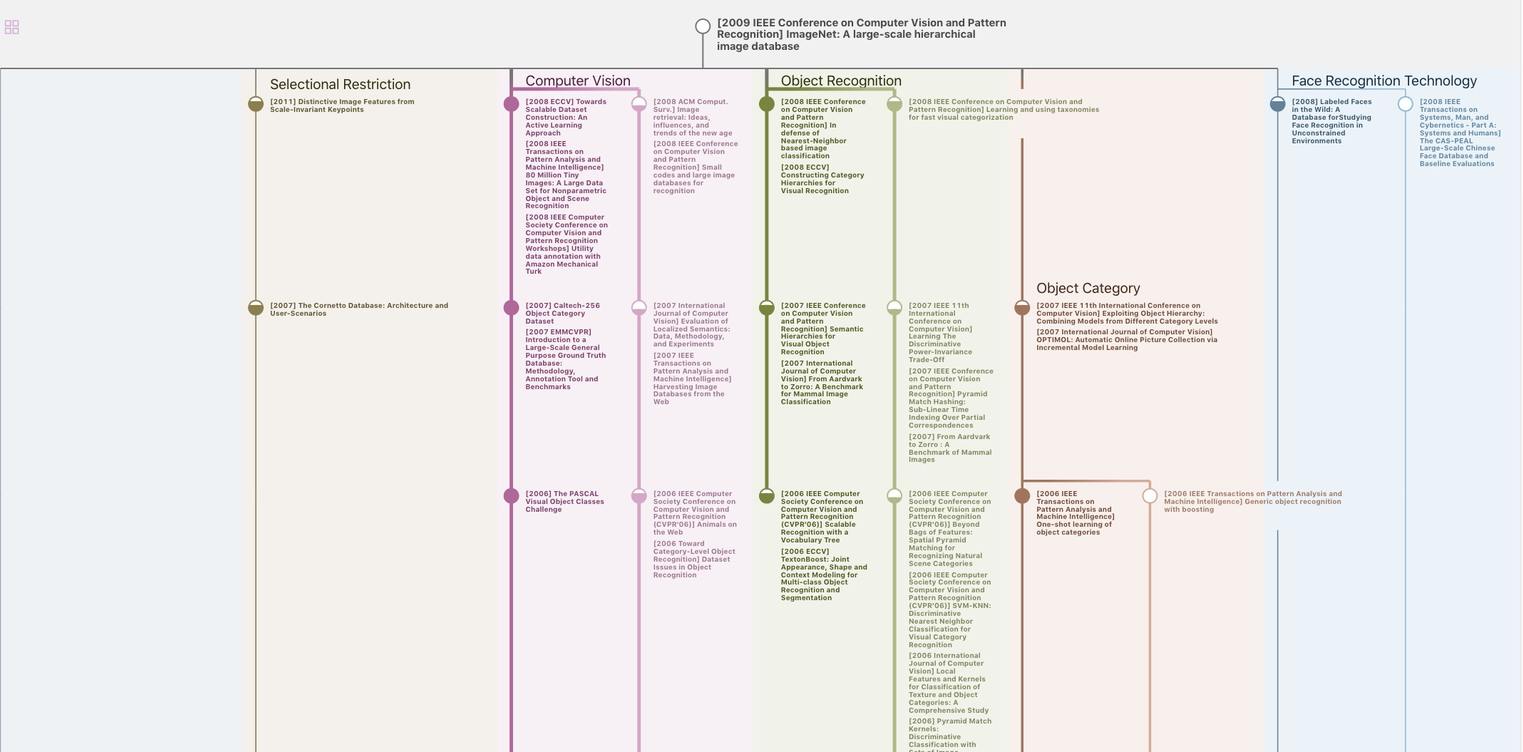
生成溯源树,研究论文发展脉络
Chat Paper
正在生成论文摘要