Recovering Bathymetry of the Gulf of Guinea Using Altimetry-Derived Gravity Field Products Combined via Convolutional Neural Network
Surveys in Geophysics(2022)
摘要
Traditional methods of bathymetry inversion from altimetry data often use gravity anomalies and/or vertical gravity anomaly gradients. These two gravity products are usually computed from vertical deflections; however, vertical deflections are rarely used for bathymetric studies. We argue that if gravity anomalies and vertical gravity anomaly gradients are derived from vertical deflections, then it suffices to conclude that vertical deflections also contain bathymetric information that can be exploited. To this end, convolutional neural network (CNN) was used to merge these three gravity signals to enhance the bathymetry of the Gulf of Guinea. The CNN-derived model compared well with individual models computed from each gravity signal using conventional methods of bathymetry inversion, as well as ship-borne depths, SRTM15+V2 and GEBCO_2021 used as references. Bathymetric profiles from all four inverted models compared well with profiles from ship-borne depths, proving that bathymetric information from vertical deflections is reliable. This eliminates the extra time and resources required to convert vertical deflections into gravity anomalies and vertical gravity anomaly gradients before inverting bathymetry. The predicted bathymetries from each gravity field signal generally depicted the ship-borne bathymetry. They yielded almost same performance metrics; however, these metrics were poorer than those observed from the CNN-derived model. The mean errors, error standard deviations, and correlation coefficients of the CNN-derived bathymetry and conventionally derived models were, respectively, − 6.45 m, 123.93 m, 0.9750, and − 9.86 m, 151.60 m, 0.9623. Spectral coherency analysis showed that the ship-borne depths correlated with the CNN-derived model better than with the other models. Results from this study testify to CNN’s computational efficacy in extracting features from geospatial datasets as witnessed in other geoscience disciplines.
更多查看译文
关键词
Convolutional neural network,Bathymetry,Deflection of the vertical,Gravity anomaly,Vertical gravity anomaly gradient,Gulf of Guinea
AI 理解论文
溯源树
样例
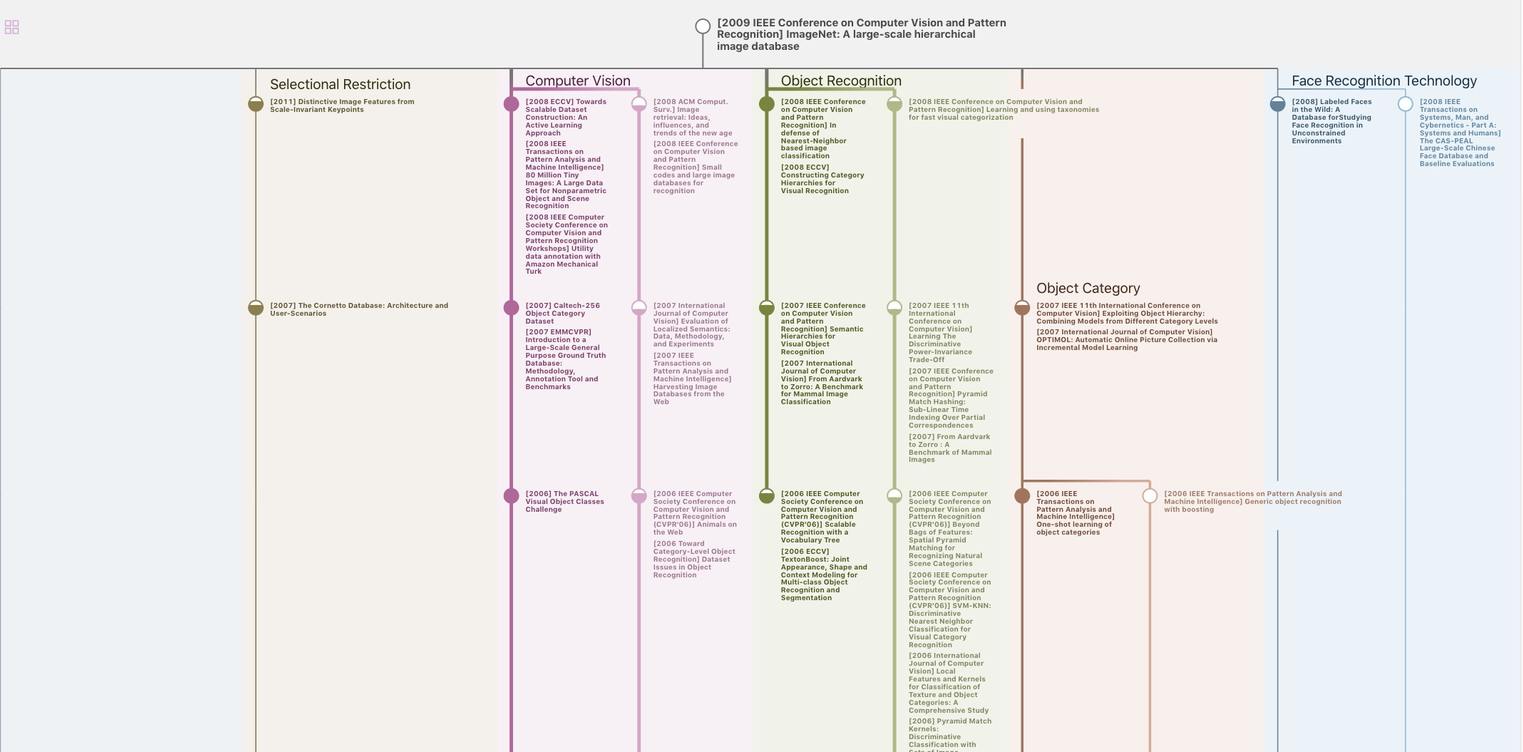
生成溯源树,研究论文发展脉络
Chat Paper
正在生成论文摘要